SHOW ME THE METRICS w/ Abhi Sivasailam
- 0.5
- 1
- 1.25
- 1.5
- 1.75
- 2
Speaker 1: This is Catalog& Cocktails, presented by Data. world.
Tim Gasper: Hello, hello, hello, everyone. Welcome to Catalog& Cocktails, presented by Data. world. We're coming to you live from Austin, Texas. It's an honest, no- BS, non- salesy conversation about enterprise data management with tasty beverages in hand. I'm Tim Gasper, longtime data nerd, product guy, customer guy at Data. world, joined by Juan Sequeda. Hey, Juan.
Juan Sequeda: Hey, everybody. I'm Juan Sequeda. I'm the principal scientist at Data. world, and as always, it's a pleasure. We're having good drinks today. We'll talk about that in a second. But we are actually live from Data Council Austin here, and we have a fantastic guest today, Abhi Sivasailam, and the backstory about Abhi is that we've been communicating over Twitter, and just, " Hey, I love what you're saying. We're on the same topics about semantics, about knowledge graphs, about calling people out when they're focused too much on the technology and not focused on providing value." And think we've really hit it off on the Slacks and on the Twitter DMs. How are you doing?
Abhi Sivasailam: Good. I'm the president and only member of the Juan Sequeda Fan Club. And it's lonely, if anyone wants to join me. And yeah, I'm doing well. For anyone that would think about joining this podcast, though, I do want to make it clear, I don't have cocktails in front of me. I was shortchanged, I was promised cocktails, I don't have cocktails. Make sure you get it in writing that they're going to give you cocktails.
Juan Sequeda: Yeah, I think we had a little... So here's the thing, is that unfortunately Tim is not here, we were going to do the whole thing, but Tim actually is a little bit sick, so because Tim is sick, we're not having drinks. So it's actually Tim's fault.
Tim Gasper: I'm sorry, guys. ... with y'all.
Juan Sequeda: So tell and toast. What should we be drinking, and what are we toasting for?
Abhi Sivasailam: I would be drinking Glenfiddich 21.
Juan Sequeda: Oh, fancy.
Abhi Sivasailam: That's the sweet spot. It's like the 30 is too mature for me, and the 18 is not enough, so it's the 21. And we'll toast a good community and good vibes. Data Council has been a good time.
Juan Sequeda: I'm with you on that. I think it was great last year just getting this community together, and then again, everybody's here, and I feel that we have... It's just the right time of everybody getting together. And I was at Gartner last week, so it's a different community, so I'm really excited. Cheers for community. How about you, Tim, what are you toasting for today?
Tim Gasper: Well, I'll toast to Data Council. Unfortunately, I'm missing it today, and I'll actually toast with my chamomile tea, which is funny because y'all didn't drink. I actually got some Portland Orange Bitters in here, so I'm the only one with alcohol today.
Juan Sequeda: All right, let's take it off with our funny warmup question. So today's episode, we're covering fast value and metrics. What are the things in life that you wish happened faster?
Abhi Sivasailam: I am the father to a two- year- old, and I would really like to fast- forward through the next couple of years. Does this get easier? You have kids, right? Your kids are younger.
Juan Sequeda: I have a five- month- old, and I guess when I'll get to two, I'll get back to you on that one. See if I want to fast- forward.
Abhi Sivasailam: What I'm learning is, kids are a lot like you, and I realize I suck, I guess, because all the things don't like about my son are things I don't like about myself, apparently. But they're very expressed at two years old. So waiting for a couple of years.
Juan Sequeda: Well, Tim, you got three kids. Do you want to follow up on that one?
Tim Gasper: Oh, man. Cherish it while it's happening. Yeah, it sucks, but terrible twos, you'll love it later. Just take lots of videos.
Juan Sequeda: All right. Well, let's just kick it off. We've got a lot to discuss. So you just finished your talk, and I have to say, I think you're tied with my favorite talk. There's two favorite talks that I've seen.
Abhi Sivasailam: I'll go fight him afterwards.
Juan Sequeda: The talk by Tristan from Continual was actually excellent this morning, but honest though, what are data teams actually supposed to be doing?
Abhi Sivasailam: Yeah. So I mean, what we talked about in the talk was, my background is I run data teams, I run growth teams, usually both of them at the same time. The mandate that I charge my data teams with is to build the company's growth model. The goal for our data team is, help the company define a growth model, which is essentially just how the company works, what are the mechanics of the company, and what's the fundamental formula of the business? And to operationalize that fundamental formula to help the rest of the company see how value is actually created and generated and transmitted within the company. And then to evolve that fundamental formula, because the business will change, the mechanics will change, the drivers will change. And the job of data team is to make sure they're on top of all of that change and expressing all of the variance in how the business is operating in a growth model, and to continue that cycle.
Juan Sequeda: So here's the thing, you have a very clear definition and you've talked about defining the growth model, operationalizing it, and evolving. Honestly, how many teams people actually do that? Because I'm going to call bullshit. I don't think most people do that. And you actually brought up something which, I don't know if it was a joke or not, I'm going to think it's not. You said there's too many data people, we should fire 75% of them. I completely agree with you. I don't know if that was a joke or not, but if it was, I think that's true.
Abhi Sivasailam: Yeah, I mean-
Juan Sequeda: Honest, no BS.
Abhi Sivasailam: Yeah, we just need to, can we close the door a little bit?
Juan Sequeda: No, keep it open. People listen to this stuff. This is exactly...
Tim Gasper: ... are a little scared right now.
Abhi Sivasailam: The number of companies that actually, what I preface my talk with is that nothing I am going to talk about today was revolutionary, right? The notion that growth models matter, that we should think about the fundamental formula of your business, how the fundamental formula of your business works, none of these things are revolutionary. What's revolutionary is actually taking it seriously, taking those implications seriously, and running your company like that. I have seen very few. Basically the ones where I'm running growth of data. But I've seen very few that actually live the practice. And that's true for a variety of reasons, and I think we can talk about a few of those, but that's true for a variety of reasons. But to answer your question on, is it BS that we have too many data people or not? No, it is absolutely, I'm serious that we do have too many data people. Look, at Flexport, I had 67 analysts in the BI org. There's way too many data analysts in the BI org. The reason why you have so many data analysts, so many analytics engineers, so many data engineers, is because we don't know what good looks like. If we actually anchored on where we want to end up at the outset, we painted a picture of, these are the metrics we want, these are the kinds of analyses we want, this is what we want to be able to do with our data, we can work backwards from there. We can work backwards from those primitives and we can define the rest of the ecosystem. The problem is, most data people don't know what good looks like. Most business partners don't know what good looks like. They don't know what that end state should look like, and so they're just grappling accretively. They're just day over day, week over week. And this kind of ad hoc grappling towards that future state is inefficient. And that's why you need a lot of data people. And I think the hope is, and we're going to talk a little bit about standardization, the hope is that with more standards, we can help more companies understand what good looks like and help them shortcut the path to getting there.
Tim Gasper: I love that. Well, so what are people then wasting their time on? Is it a lot of the, for example, transformation work? Like, " Oh, I got to write another job to make a new thing to output another thing." Is that the grind where we're wasting a lot of time these days?
Abhi Sivasailam: Well, I like to call this arbitrary uniqueness. Within companies, most of the variance of what we do with data, the analytics that we do, the internal- facing data science that we do, the metrics that we report on, the dashboards that we make, should be standardized, could be standardized, across business model grounds. For the most part, for all these things I'm talking about, B2B is B2B is B2B, marketplace is marketplace is marketplace. E- commerce is e- commerce is e- commerce. And the wasted efforts in all of these companies, in all these data teams, is folks that don't take seriously how much variance you can explain with business model, how much you can actually just standardize on, " I am a B2B SaaS company, therefore I should be doing these kinds of things. I should actually be looking at these kinds of dashboards, these kinds of metrics. This is the fundamental mechanics of this business that I work for." And that arbitrary uniqueness manifests as rework on metric calculations, rework on defining the metrics, rework on data models, what should our ideal state of data models look like? Rework really every step of the way. And that rework creates along the way, data quality problems, it creates along the way, efficiencies in tech debt that you have to work around. But it's all grounded in this arbitrary uniqueness where we're reinventing the wheel when we don't have to.
Juan Sequeda: So couple things here. One is, I think is it not just I think the data teams, and not just data teams but just technical side, the technical folks in general, don't understand how the business works. And I think this is one of the stuff that I've been just banging this from, I think we agree a lot of this is that, we talk about data literacy when you have more of the business literacy. And I think having these metrics is one of those things to make it clear what this stuff actually means. So understand what the business is. Why aren't they understanding the business? What are the motivations or what are the behaviors you need to go change to get that? And second, I want to bring up something that, your partner in crime here is Ergest.
Abhi Sivasailam: Mm- hmm.
Juan Sequeda: Right? Ergest has actually been a guest on our podcast before. And Tim, you brought this up recently too, Ergest wrote this tweet saying, " Hey, data teams should participate, help out in the whole P& L." And this was a backlash around Twitter, like everybody... And I'm like-
Abhi Sivasailam: That was very controversial.
Juan Sequeda: He was very controversial. So let's dive on this thing, on this point again a bit like, data teams involved in how the business works and how... Thoughts, comments.
Abhi Sivasailam: Well look, I mean, part of the problem, so let's start with the business piece, part of the problem with a data team, an engineering team, a what- have- you team, understanding how business works, is, well look, the business might not understand how itself works. Your business partners in marketing may actually fundamentally not understand their own growth model. And if they don't understand their growth model, how can you be expected to understand your growth model? How can that context be communicated to you? So I mean, one of the reasons why Juan and I started talking is this notion that this knowledge around these processes, how value is created in the business, basically these ontologies, why I love knowledge graphs, those are at best tacit in people's heads, but often not even tacit in people's heads. There isn't that exercise of let's actually document how value is created in this company, how value is transmitted, how it's transferred in this company. That artifact doesn't exist. I think that's an important artifact. This dream of having this knowledge graph of how the business works, I think would go a long way towards creating that shared understanding. But we don't have that today.
Juan Sequeda: I've lost count how many times I've said in the last week about how I disliked the word data catalog because it's more about not just about cataloging data, it's about cataloging knowledge. And it's exactly what you're saying that we need to have more. I think the metrics is one of the first steps, is to understand, what are we measuring? How do we define success around that stuff? And then it's about how are these things related? What are those most important things, the most important concepts, what are the relationships under... And keep tracking of the business processes. And you know what? I understand that we don't know, that there's not one definition of customer, there's 10, 15. What are those? Let's go write them down. And it doesn't matter if we agree. We need to figure out where we don't agree to figure out later on where the North Star is, where it should be.
Abhi Sivasailam: Yeah. And I think metrics are at the core. I think metrics properly defined, again, if you know what good looks like, if you know what the end state needs to look like, metrics form the core. And metrics are actually one of the most durable concepts in an enterprise, and they're often more durable than some of the concepts that underlie it. So you mentioned customer, right? Well look, the metric churn is actually more durable than the entity customer underneath it. A customer could mean one thing today, it could mean another thing tomorrow, it could mean five different things in five different organizations. But the metric around churn, the calculation around churn, the business logic around churn, again, properly construed, properly thought about with an end state in mind, is actually more durable than the underlying concepts. Which is one of the reasons why you start with the notion of metrics. I look at an enterprise as, well, I mean, everything for me is knowledge graphs, and everything is kernel knowledge graphs. But I think at the heart of an enterprise, the most foundational graph is actually a metric ontology. Everything else hangs on top of that. You start with the metrics ontology, then you hang initiatives on top of metrics, you hang people on top of initiatives, you hang resources on top of people. But the core of the enterprise should actually be metrics. That's the most durable concept, properly construed, in the modern enterprise.
Tim Gasper: Abhi, can you talk a little bit more about, what do you think of as a metric, and then what do you think of as a concept or some of these other things that you're talking about? Just to get into the definitions a little bit more.
Abhi Sivasailam: Yeah. Well, I mean, in the case, by way of example, in the example that we were using, the metric would be churn. It's a business fact about how value is created in the enterprise or performance is measured in the enterprise. These concepts that underlie those metrics are the inputs into the calculation. So in this case, customer, well customers is an entity for which we measure the value through the me metric of churn, for which we understand the performance through the measure of churn or acquisition.
Tim Gasper: Okay. And how does knowledge graph tie to this? Why is knowledge graph everything around this?
Abhi Sivasailam: Well, I think knowledge graphs are a useful abstraction. We don't have to make it everything, but I do knowledge graph as a useful abstraction of thinking about, what are the constants in the business, and most importantly, how they relate. One of the most important things that's missing when we work with metrics is that metrics are missing the relationships between metrics. If I just have metrics on a dashboard, all those metrics are treated in isolation. I have metric A, metric B, metric C, but I have no sense of the hierarchy of those metrics, the influence that one metric has on another, the interconnected web of relationships across these metrics. Well, what does that interconnected web sound like? It sounds like a knowledge graph. It sounds like a graph that expresses how entities are related to one another, and related semantically. So I think it's one useful way of thinking about abstractions, but really when I talk about knowledge graphs, what I'm interested in is concepts, but concepts and their relationships with each other. And that's missing in a lot of how we think about both metrics, but also how we think about business entities.
Juan Sequeda: So then actually one of the questions I heard at the end was about operational questions. And I was just, if you have a well- defined semantic layer, your knowledge graph of all your core entities, effectively, the metrics are just going to be a function over that. It's just going the inputs, as you mentioned, the inputs are going to be the customer and all these things that happen around that. And it's really a query of function that's operates over that. And then the operational questions are going to be some other type of question over that, that are not then fully related possibly to metrics. I think that's how I personally see this. And what I'm struggling with or the people are just, in this world of being so efficient that they don't separate this. So then they do this all this work and write all this SQL to do that when you're like, " Wait, you had important abstractions, important concepts, important knowledge, important semantics that you just hid inside of your dbt transforms or queries that end up ... these CTEs and you're like, " You should have abstracted that so you could go reuse that." And because later on, there's important business knowledge there that, we lose it. And I think-
Abhi Sivasailam: Yeah. But the problem, I mean, you mentioned dbt transforms, the problem isn't dbt, the problem is, again, accretive redevelopment. The problem is-
Juan Sequeda: People.
Abhi Sivasailam: ...you don't know. Yeah, you don't know what the end, you don't know what good looks like. You're not starting at the solution. You're not starting by taking a step back, what the house you're trying to build looks like, you're building it as you go. And as you go, you create that debt. And that tech debt looks like the abstractions that you steam roll over. The semantic knowledge that you might have actually pulled out if you had a plan of attack on how you were actually going to go about this.
Tim Gasper: This is super interesting because, to your point, Abhi, I think that folks tend to steamroll over the abstraction piece. And to even go further than that, I think we've spent basically decades thinking about the physical manifestation of the data, and to some degree, the logical models and things like that that we're building around the data, but very close to the data constructs themselves. And then we talk about business definitions and concepts and things like that. And there's obviously things like dashboards. So it's like we've got the three corners of this triangle, but then we just gloss over the middle. We're like, " Ah, that's the means to the end." And the end is the corners. So is part of what you're articulating here, we need to think of the middle more as part of the end? We need to treat it with a lot more respect and a lot more focus?
Abhi Sivasailam: Yeah. I think that's a good way of describing it. And I think, again, I keep going back to this accretive development problem. The middle will be clear if you know what the ends look like. If you know exactly where those points in the triangles look like and how they're expressed, it'll be easier to design the middle. We're more likely to end up with a solid middle.
Tim Gasper: Okay.
Juan Sequeda: So let's talk about standardization. And you've been doing all this SOMA standards metrics so, floor is yours, continue.
Abhi Sivasailam: Well look, I mean, we're talking about knowing what good looks like, and we're talking about cutting arbitrary uniqueness. So I mentioned a few minutes ago, for the purposes of metrics, analytics, internal- facing data science, which by the way is where 90% of the value from data teams comes from, for those artifacts, largely, what you do should be determined by your business model. Are you a B2B SaaS company? Are you a B2C SaaS company? Are you an e- commerce company or you're a marketplace company, et cetera? And if you understand that, then we can actually create community- driven standards for what good looks like. Where does the kind of end- goal state look like for metrics for analytics, and work backwards from there. And that's what we're trying to do with this open- source project I'm leading with Ergest Xheblati called SOMA, Standard Operating Metrics and Analytics. And the notion there is, so we are, we're just starting to soft launch it, but you can follow us on social media and whatnot, and you can see us release updates to our GitHub repo and our website. But the notion is, for a B2B SaaS company, look, there's 250 or so standard metrics. Doesn't matter what B2B SaaS company you are. These are basically your core metrics that you should reason about when you understand how your business works. For each of those metrics, we specify a definition, a standardized definition, because these definitions are often gamed. Net dollar retention means different things in different companies, for totally arbitrary reasons that aren't justifiable. So what should net dollar retention actually look like from a best practice perspective? What are the dimensions that you want to pivot that definition on? What are the aggregations you should look to apply? What are the grades you should look to calculate that on? So we start there. The standards that we're talking about starts by anchoring on the actual exposures, the metrics and the analyses and the dashboards, what should those look like? And then we work our way backwards and we say, " What is the fastest way for us to streamline, get to those metrics in a way that doesn't create tech debt, that preserves all of the local knowledge that we have about how the business works?" And for us, we think that way that you get there is through a mapping of your raw data to activities, business activities. And so this is another big thing for me. I really like what Ahmed and co have done with Narrator or the activity schema. I think the activity schema is an underrated concept, and basically the activity schema is, hey, instead of thinking about your business as a bunch of tables, instead of modeling your data as a bunch of tables, instead model your business as a ledger, as an immutable ledger of facts that happen in the business. And we take that core concept and we go a step further, and we create, in SOMA, we create, for SaaS for instance, we create 110 or so business events. These are very semantic business business events. SDR books a demo where SDR and books and demo means something, and they have identifiers. Customer renews a contract, where each of those things mean something. It's a very semantic business event. And what we say is, " Look, if you can map your raw data to these activities, if you can generate these activities, then those activities are designed to plug into the metrics. And we know what those metrics seem to look like, so we'll hang those metrics on top of these activities." That's the long and short of how we're thinking about it. And the hope is, again, fire 75% data people. The hope is that we can cut a lot of the arbitrary uniqueness. We can cut that accretive development, which is totally unnecessary, and the much smaller data teams can focus on what is actually bespoke to the business. For instance, one thing that's actually hard to standardize is product metrics. You can standardize these at certain levels of abstraction, but only certain levels of abstraction. I can say a PQL, a product- qualified lead, is a metric. I can create an activity in our world that maps to that PQL metric. But if I wanted to dig deeper and I wanted to understand, for Data. world, what is the PQL? What are the drivers of that PQL point? There's only so much I can standardize. At some point, it will start becoming product specific, it will start becoming long tail, it will start becoming bespoke. But that's what data team should focus on. They should focus on bespoke value, they should focus on what is truly unique to the business. Whereas today, most of the work is actually on the non- unique. It's on the arbitrarily unique. So the goal is, if we can define what that core looks like, we can hang the uniqueness on top of that, that core structure of standards.
Tim Gasper: Interesting.
Juan Sequeda: That's a very key important takeaway there, is that you could probably look at the work and you could divide how much of the work is just reinventing the wheel that other people in other organizations, similar, I mean the same industry, are like, we're asking the same type of questions. There's no reason why we should be able to... The work that I did here, somebody else somewhere else is doing that. And that's not even competitive work. Then there's other work that is very specific to your organization, to your company. You're getting into the specifics of how our product uses. So data teams are just, you should basically focus on stuff that's specific to you, and not spend the time on stuff that is just freaking repeatable. It's like, " Why am I doing this?" Because there's other people typing in the exact same character somewhere else. That's waste of time.
Abhi Sivasailam: How do you really feel?
Juan Sequeda: I am just so freaking frustrated to go see... Look, I'll be very honest, hearing everybody saying, " Oh, the data engineers are spending too much time. They're so bad." I'm like, " Yeah, because they're doing stupid amount of work that is just repeated, repeated all the time that they shouldn't be doing that." If they would be actually focusing on this stuff that is very specific to the organization that provides unique value, that's the stuff that we need to make sure that we need to empower them. But we're like, you're spending all this time on stuff that's like, you should've done that. You're reinventing the wheel. And I think this is why we take one step forward and like three step backs full of time and then we reinvent the next technologies does this and we got to go do that again. And then we just keep going around in circles and circles. Anyways.
Abhi Sivasailam: And I will say, there have been attempts at automating all of this. There have been attempts, I mean, the notion of Fivetran, Transform, whatever they call it, dbt packages, Looker Blocks. There have been lots of attempts and tons of commercial tools that try to sit on top of your Salesforce instance and give you all your sales metrics, that try and send top of your Stripe source or Google Analytics and try and give you all the relevant metrics. Look, my view is, that fundamentally doesn't work. Sources are too heterogeneous. This is certainly true for complex sources like Salesforce where no two Salesforce instances in the world are implemented the same way. But it's also true even for simple sources like Google Analytics, or even simpler sources like Stripe. Even the semantics of charge codes within Stripe or the way I actually, from a business process perspective, handle things like refunds, are heterogeneous. So it's very hard to standardize. So there has to be translation, there has to be a translation, there has to be one layer of abstraction up. And this is why all these tools have failed. All these approaches I think have failed because there has to be an abstraction layer in between. That abstraction layer should be highly semantic, it should think about the relationship of these core entities, and then humans have to do the mapping. All right, I hear a comment that poor Tim needs to get a word in. So Tim, you got the floor for five minutes.
Juan Sequeda: Oh shoot. Somebody called me out. Okay, I'm going to shut up. All right.
Tim Gasper: No, you're fine here. I'm actually fascinated by this recent turn of the conversation here.
Juan Sequeda: ...
Tim Gasper: So you're saying that there shouldn't be automated integration. You're saying that actually, what humans should do is to translate things to the semantic layer. Now, I think what is interesting about what you're saying is that we don't have to get super, super creative on that semantic layer. You're saying that we could actually leverage more of a standardized off- the- shelf semantic layer. And I think that's a super interesting concept, and I'm curious for you to add more to that, but I also want to put a fear out there, and my fear is, and I'm curious, Abhi, how you respond to this, is that we're just starting to get this industry to the point where they're like, " Oh, wait a second. Maybe I do need a semantic layer. Maybe that idea from 30 years ago was a pretty good one and we should actually come back to that." But now you're actually kind of taking it one step further and being like, " Hey, and don't get super creative about that. Let's just use more of a standardized semantic layer," which is like, okay, maybe is that a leap too far? Curious about your thoughts.
Abhi Sivasailam: Well first of all, you have two more minutes to talk. We said we would give you five, so got two minutes of air time to kill.
Tim Gasper: I give you two minutes to the floor.
Abhi Sivasailam: So I don't think it's a bridge too far. Let me start there. I don't think it's a bridge too far. I think it actually lowers the activation energy. It makes it easier, because instead of having to do a lot of the rework, and instead of the vendor lock- in from having proprietary semantic- layer implementations, you have open standards for how you think about that semantic layer. So no, I think it actually streamlines the adoption and makes it easier to move on to the semantic layer. What was the other question before that?
Tim Gasper: The idea that automated integration is not the goal here. It's really more, a human should be part of that.
Abhi Sivasailam: Yeah, no. So yeah, I fundamentally think automated integration is impossible. I think humans have to do the translation to abstractions. And we should define what those abstractions are, and we can help humans tremendously. Because look, one of the problems, data modeling is itself just a process of humans doing the integration, mapping to abstractions. The problem is data models, dimensional modeling, is a hard abstraction to couple to. And it's a very vague abstraction to couple to. You're basically given a set of first principles on how to generate dimensional models, and you're told to run. Instead, I think we can do a lot better. I think we can help people understand, what are these nice semantic primitives that you should map your data to, and if you can button map your data to those semantic primitives. And the way we implement this in SOMA is by the way, actually as RDF triples, so it's like actor, action, object. The actual payloads themselves are designed to be very semantic, so that we can definitely use them in a relational database context, but you could also ideally load them into a knowledge graph. You can also finally start running... There's all this notion, this hype around LLMs running SQL queries and all that. I don't think that's ever going to work unless the data that's underneath is highly semantic. And so this is a way to get the data highly semantic. So yeah, look, I think we can be prescriptive, I think we should be prescriptive, and I think being prescriptive lowers the activation energy that should hopefully expand adoption.
Juan Sequeda: Can I speak now?
Abhi Sivasailam: Yeah, you're good.
Tim Gasper: ...
Juan Sequeda: So I'm already thinking about the naysayers saying, " Hey, here is this standard, you should be able to go off to use off the shelf. And they're like, " No, no, but we're different." I mean, as you said, you're not snowflakes. We're not a-
Abhi Sivasailam: Arbitrary uniqueness force field is strong. Yeah, arbitrary uniqueness force field is strong.
Juan Sequeda: Because you're put this thing up, people are like, " Well, hold on. We can't use that as- is because we're very different, so we're going to go spend time and go change things."
Abhi Sivasailam: Like this is not a hard problem. It's good cop, bad cop. Juan's, bad cop. All right, we'll just come to every organization, we'll knock on their door. Juan's obviously bad cop, and I'll be good cop, and we'll change the world. Juan company at a time. Now look, the arbitrary uniqueness force field is strong. I hear that. I think about this a lot. What is the wedge? How do you break in to driving this kind of standardization when there are strong headwinds? But look, what I've seen is, let's take marketing orgs for instance. I've worked with a lot of marketing leaders in my career where they come in with a set of metrics they think they want. And they say, " This is how I like to look at a business, and these are dashboards I want." But I've never had a conversation with a CMO where, if I brought them standard, a true open standard, and I said, or at least what I told them was a standard, and told them, " Well, this is actually a better way to define the metrics reason about the metrics, and this is a better set of metrics and here's why," where they didn't buckle. And I think the key is actually the imprimatur, the aura around the standard itself. No one says, " My business is a special snowflake, so I'm not going to use GAAP, generally accepted accounting principles." Now, part of the reason they don't say that is because of the legal ecosystem around it, but part of it is actually the existence of a standard. And the existence of a standard where... I mean, look, bandwagon effect is a powerful thing. And if you know that peers of yours are having faster time to value and have coupled to an open standard, I think there's strong incentives to also couple that standard. If you have pressures, I mean, I talked to a lot of VCs who are now pressuring their portfolio companies to couple to standards like SOMA, because otherwise, those VCs can't actually understand how one portfolio company is doing against another. It makes benchmarking impossible. So look, there's carrots here, there's sticks here, there's bandwagon effects here, but you need a standard to exist first before the standard can be adopted. And so far, we haven't had that. So the idea is if you have that, then we'll try and use carrots, sticks and bandwagons to try and make it work.
Juan Sequeda: This is another big takeaway you just said there for the investors or VCs in the audience, just, you probably need to be the one forcing down this metric standard, because that's going to help you, the investor, the VC, saying, " I have a standard way of comparing." So now you're just basically mandating your companies to go do that. So I think that's actually a really reasonable thing to go mandate. And at the end of the day, it's like you make the investor's life easier, you make your life easier as a company startup. I hope that happens. I hope we start seeing this.
Abhi Sivasailam: I hope so too.
Juan Sequeda: So what's next for SOMA, and yeah?
Abhi Sivasailam: So we're just starting to baby launch SOMA. So we're starting with just a snippet of growth accounting metrics, just for B2B SaaS. Over the next couple of months, really by July, we're targeting rolling out all of B2B SaaS, all of the activities associated with B2B SaaS, all of these metrics and the standard calculations. And then we'll slowly start to expand to B2C, e- commerce, marketplace. A lot of this work has been done already, but we want to make sure the standard is battle tested. So we want to make sure we are actually working with companies, we're actually doing implementation, we can point to real- world examples of success to help drive that adoption. But you can follow us, I mean, I would recommend you follow both Ergest Xheblati and myself on Twitter, as well as right now, somastandard.com is pointing to a GitHub page, and that's where you can follow, again, just the baby feather launch. But there'll be more launches here following in quick succession, over the coming weeks.
Tim Gasper: And that's github.com/ SOMAStandard, right?
Abhi Sivasailam: Or go somastandard. com, it'll route you there. Yeah.
Tim Gasper: somastandard. com. And if a company wants to start using this, what's the best path for them? And then more specifically, as a data person, if I want to think about, how does my life change and how does it engage with something like SOMA? If I wanted to start adopting it, I'm a data engineer or an analytics engineer, what does that mean for my life and my day?
Abhi Sivasailam: Yeah. Well, I mean, look, the way I... dbt Is very common these days, and I'm sure a lot of your audience has dbt. So I'll take a a dbt- focused approach to this. The goal for me is that, for all of the activities we're talking about, these 110 business activities that you can represent a SaaS business with, these very semantic events that happen, those are basically expressed now as dbt models where instead of a dbt model for every entity or staging tables or whatnot that you have. What you start with is by mapping your raw data in whatever form, by using that local context, to map that data to activities. Activity by activity, dbt model by dbt model. And the idea is that once you have that, now you've generated the raw materials for the rest of SOMA to translate into metrics, to have this immutable ledger that can then be modified and exposed into varying outlets, whether it's a knowledge graph or a BI tool directly, or just for people to query. But the starting point is, start thinking about your business as activities. And I think, even if you don't use the rest of it, the thing is like, SOMA's designed to be modular. You can use all of it, you can use none of it, you can use some of it. There are folks that can just use the metrics, but nothing else, and no calculations, no automated calculations. But you can use the metric definitions, and that alone is helpful. It alone is helpful just to know that as a marketing team, I should use these metrics. As a data analyst, a supporting marketing team, these are the metrics I should be thinking about. This is how I should slice them. This is probably how my dashboard should look. That alone is helpful. If you would like to just use activities, I think that alone is helpful too. Thinking about your business as a set of activities, reasoning out your business as these semantic activities, and that being a new staging layer, and then building entities, or whatever kind of data models, wide data models, dimensional models on top of that. Or you can use them both together. So I think there's a lot of different ways to go, depending on what's most salient. I think most teams should probably start by just metrics and metrics definitions, and trying to fold those definitions into their current way of operating, trying to present those metrics to business partners, and then slowly start expanding backwards into pipelines.
Juan Sequeda: So in your talk today, you focused more about the design.
Abhi Sivasailam: Mm- hmm. Of metrics trees. Yeah.
Juan Sequeda: But we didn't get into the building part which people would want to go here. So from what I've extrapolated, the building part is a lot about activities, and specifically, you're talking about the activity scheme, the scheme. That's one. Second, a way of implementing this would be through dbt models. In fact, we're just writing a bunch of SQL in this stuff. Is that the way? Are there more ways around that? And also, who are the people? So we talk about data engineers, we're talking about, there's the analytics engineers. Who are the people who are actually implementing building these things within an organization?
Abhi Sivasailam: Yeah. Well, the metrics, it depends on your organization. There's at least four different definitions of what a data science person does at a company, data analyst, BI analyst. There's this ...
Juan Sequeda: So after we fire those 75% people, the 25% left are who?
Abhi Sivasailam: Well, look, there's folks that are focused on metrics and BI. Those folks are going to be focusing more on using the metric standards, whatever that looks like, but using those metrics and presenting those metrics in dashboards or WBRs, NBRs, whatever interface makes sense. And then there's folks more on the engineering side that are actually doing the data mapping. But I think that's how you see the work splitting up here, that there's folks that are actually doing the raw mapping of raw data into these activities, into this abstraction, and there's folks that are hanging at the end of the value chain that are taking the data that exists, either after it's in a activity format, or taking the data as it currently exists in its current format, and expressing it as metrics. What we also talked about in the talk was, what do you do with those metrics when you have them? And the focus of the talk that Juan is referring to, that we just got out of, is that we started this conversation with this notion that what data teams should do is help build a growth model. But we haven't talked at all about what a growth model is in this podcast. So once you have the metrics, the key is to figure out how to tie those metrics together. Again, relationships are what matters. So it's great that you have new MRR, it's great that you have existing MRR, these growth- accounting metrics. What's important is to start building a tree, building a web, of, " This metric has these drivers, and I'm actually going to start specifying these relationships." Because if you can start getting explicit, and you can start getting explicit just on paper. You can just say, " I have these 10 metrics. I'm going to draw out metric one, metric two, metric three, and I'm actually going to draw out the relationships." But if you can start getting explicit about those relationships, then you can start doing all the things, all the use cases that we talked about in the talk. Once you start getting explicit about that, root cause analysis, which is a major pain point for data teams, can be completely automated because you're actually explicit about what the driver chains are. Forecasting can be largely automated because forecasting is just a function of forecasting inputs to get those outputs. You can operationalize these metrics in a much better way by using the relationships between the metrics to run planning cycles. How many planning cycles have you seen be successful? I've seen very, very few. And that's because planning cycles aren't based on a web of metrics and their relationships. No one actually knows how metric A ties to metric B. This is why OKRs end up being a disaster in most organizations, because your team has OKRs, my team has OKRs. We have no model to relate our OKRs together. But if you actually build that model of, this is how the constellation of metrics is interrelated, then the initiatives that we layer on are oriented towards that web. Now, you're looking a little stressed.
Juan Sequeda: No, no, now you open up a weird question, which is, what's the relationship here between all the metrics and things like SOMA and stuff that you're defining with OKRs?
Abhi Sivasailam: We talked about this a little bit a few minutes ago. I think the problem with OKRs is simply that, well, the predominant problem with OKRs is simply that they don't have a model that they hang on, of how all of these symmetric are interrelated. Most OKR- based planning cycles fail because you create your metrics in isolation, I create my metrics, my OKRs in isolation, and they're not hanging on a concerted model. So again, if you can focus on, what is this... What we talked about earlier today was, the goal for me when I run these data and growth teams is to build one giant driver tree. I start at the North Stars for the business or the organization, and I start decomposing, decomposing, decomposing, inputs, inputs, inputs. And what you want to do is you want to take all of these metrics and you want to plant them on a web where you understand how all of these metrics relate to another, they decompose, what's their hierarchy? Because that's the real power. And that's the problem with BI tools today, that they're static, you don't have those relationships. But if you have those relationships, and those relationships are explicit, planning now becomes much easier because you orient your planning towards that web. Forecasting, root cause analysis, business reviews become easier, because you start on a part of the tree and you work your way down. All of this is possible if you actually think about the metric ontology, if you will. And the idea is that SOMA and all of this make it easier, because we know what the metrics are, then we have those metrics created, and so now it's easier for us to start sketching out those relationships.
Juan Sequeda: No, this goes back to, sometimes the business doesn't even know how the business works, so then it's hard to even define what these top- level North Stars are and the other metrics. And that's why OKRs can be a disaster around these things. Wow. So much stuff. I mean, we're taking notes here, as you can see. Tim, you got any final thoughts before we go to our lightning round?
Tim Gasper: No, I queued up a couple of lightning round questions that I think are going to open up some interesting conversations. So maybe we leave a little bit of extra room for context on our lightning round.
Juan Sequeda: All right. Well, let's kick it off. Let's move to our lightning round question, which is always presented by Data. world. I'm going to, number one, we talked about the importance of metric layers and the metric standard. Is it important to have a metric store?
Abhi Sivasailam: By metric store, do you just mean that the metrics are actually pre- calculated, and serve as a pre- calculated entity?
Juan Sequeda: I would say that there's now products and technology around metrics themselves. There are even tools that do this. So basically, do we need more technology around this stuff? Or frankly just, it's a bunch of SQL, you write an activity scheme and that's it?
Abhi Sivasailam: Yeah, look, I mean, think I'm not so concerned about implementation details here. What I do at every company is, I actually just pre- calculate the metrics. So I build something, I joke that it's called a data net. A net is a projection of a cube in 2D space. But every company I go to, it's like blank stats. So Flexport is Flex stats HoneyBook, it was HoneyBook stats, and it's just a stats table that's a flattened cube, and it's just every metric at every grain, at every dimension, at every period, at every aggregation has a row. And that becomes the predominant interface. That's what powers dashboards, which means dashboards go lightning quick. That's what powers exploratory... In Looker, that's actually the most important Looker explorer. Because most of the time, people don't want to do a bunch of exploratory analyses on entities. They just want to find metrics. They just want to find metric relationships. They just want to know what the metric answers are. And so that's what we hook up the primary, the most used Looker explorer in the company to. And when you really pre- calculate metrics and you treat them as a primitive, then you can much more easily do things like correlation analysis, automated forecasting, automated root cause analysis, et cetera. So look, maybe this is stupid or simple, but I pre- compute everything at those grains, at those permutations, and I persist them, and that's one big metrics table, and that's what I use. I don't think you need to be more complicated than that necessarily, but to each their own.
Tim Gasper: Right.
Juan Sequeda: I love it. You don't even need to complicate it.
Tim Gasper: Yeah. No matter how you decide to implement it, by doing this, you can solve a lot of problems, and the performance should be a lot faster too.
Abhi Sivasailam: Yep.
Tim Gasper: Interesting. All right, second lightning round question. Five years from now, will metrics layers be commonplace, or is the adoption cycle going to be longer than that?
Abhi Sivasailam: Metrics layers? I think metrics layers are... Look, the challenge of metrics layers is where should they live? The challenge of metrics layers that aren't in BI tools is, how do the BI tools talk to them? And I think the metrics layer tools that we see on the market today are facing this existential question of, well, most of the world uses Power BI or Looker or Tableau or something else. How are they going to talk to the metrics layer? And I think it's an unsolved problem, and I think it's a really existentially deep problem for these metrics layer tools. And I don't know that there is a clear resolution. I think it's very possible that the right metrics layer solution is actually an open- source standard for metrics layer. I mean, years ago, I thought Looker should have just open sourced LookML, and that everyone should just use LookML. And basically the idea was, "Oh, LookML is now our standard metrics layer. Everyone uses it. We create network effects around this protocol, but we are the first and best consumer of that protocol because we are the sponsors, we invented it, et cetera, and we focus on the last mile. We focus on the last mile value you can get from this protocol." I think if I had to bet on a long- term, steady- state evolutionary equilibrium, it would be that metrics layers have to live very close to BI tools, and the best way for them to live very close to BI tools is that's a shared protocol where BI tools now just focus on that last mile. But that's an unlikely world for a lot of reasons.
Tim Gasper: Interesting. I think, I have a little bit of a follow- up question that'll come later, interestingly, about that, but I think what's also interesting is that you actually separate this idea of standardized metrics from this idea of the metrics layer quite a bit, it seems like. And I think that's a big aha moment for me.
Abhi Sivasailam: That's an implementation detail. I think the metrics-
Juan Sequeda: But it's an important one. Because I think people will combine them.
Abhi Sivasailam: It might be, but again, to my notion about this Flex stats or HoneyBook stats or whatnot, we can bypass that implementation detail entirely. So people might conflate it, but it is an implementation detail. Metrics themselves, you can think about more broadly, whether it's in a metrics layer, whether it's, you do not pass go, and you jump straight to a metrics table or something. Lots of different ways you could do this. Again, I don't know what the steady state of that implementation layer is. I will say, SOMA thinks about jumping right to the nets. So in Selma, we're trying to create nets because that's vendor agnostic.
Juan Sequeda: I appreciate how we've been trying to get you a little bit more into the technical details and you're like, " Nah, it's an implementation detail, it's not important." So next question I got, as we implement standard metrics, will that help data teams understand the business more?
Abhi Sivasailam: Yeah. I mean, the hope is that there... Look, there's information in the metrics. There's information in the definition of the metrics. But also, once you have the metrics, if you know what the metrics need to be, if you know how to get to the metrics easily, you cut a lot of that wasted arbitrary uniqueness, and that you can focus that on not only what's unique, but also, as a data person, getting context. So it frees up just a misallocation of data team resources that we struggle with.
Juan Sequeda: Final question, Tim.
Tim Gasper: Final question. It's going to bring back something you said earlier, Abhi.
Abhi Sivasailam: Okay.
Tim Gasper: If we follow standardized metrics all the way forward, this vision comes to full reality here, does BI and analytics actually become a simple automated last mile thing?
Abhi Sivasailam: Well, yeah. I mean, I think the ideal for me is that everyone should focus on last mile. BI tool should all just focus on varying approaches to last mile. That could look like being the best visualization tool possible, that could be look like being the best, I guess what people call data activation or whatever, tool possible, where you are... I mean, Looker does this really well, where Looker is integrated, you send reports from Looker to a constellation of other tools. Reverse ETL lite, if you will. But you can send email campaigns directly, you can send customer engagement campaigns directly, et cetera. So I think, look, the ideal would be that we commodify what should be commodified, and the commodification creates platform network effects that allows people to create value on the long tail. And that's where I would love for them to create long- tail value, where I would like them to create value. In the same way that we misallocate human capital with arbitrary uniqueness, we also misallocate company capital, financial capital, by investing in all of these tools that are also reinventing the wheel over and over again, when large swaths of what those companies do should be utterly commodified. And we should then allow those companies to focus on what makes them unique. That's the hope.
Juan Sequeda: This has been a fascinating conversation. We got so much notes here. Tim, kick us off with the takeaways.
Tim Gasper: All right, data team mandate, build a company's growth model. Define a growth model, operationalize the fundamental formula, and evolve the fundamental formula. I think this was a pretty big aha moment, a big takeaway, because I think that data teams sometimes feel that they are very much this supporting function, and they're an order- taking organization, and the idea that they could be so integral and so involved in helping the company to understand their business and evolve it and make it better, despite being slightly obvious on its face, is also a very under- implemented model. Metrics aren't new. What's revolutionary is maybe taking it more seriously. Too many data people. This is one thing where, if we really implement these metrics models, maybe actually, a lot of the effort that we're playing right now and that data teams are doing, is very redundant, repetitive, mundane work, that it should actually be focused on just mapping to the standard model. And they could be focused on what you call these more important long- tail activities, the things that are truly unique to your business, not the stuff that everyone has to spend what seems to be many months redoing the same work over and over and over again. And you mentioned this idea of arbitrary uniqueness. This idea that every company feels like they're a snowflake and that they've got to spend those... All of us have worked at a lot of companies, and if you are redoing your data stack, you're like, " Oh, we're in for the next three years, we're going to redo our data stack." But is that really true? Do we always have to go through these same motions? The same idea of arbitrary uniqueness. And you said, " Hey, B2B is B2B, marketplace is marketplace, E- commerce is e- commerce. There's much less variance than we think. We shouldn't be doing all this rework on metrics, definitions, and data models." Why aren't data teams involved more in the business? Well, the business may not understand itself how it works, and a lot of times, the business model is either tacit in people's heads or not even tacit. It hasn't been documented, it's not explicit. What we should be focused on is the metrics. We should be trying to make sure that the metrics are durable, things like churn. That is a business fact, that is a calculation. We could really be building all of our data around these durable pieces of these durable elements, and not enough people are focused on this. And we shouldn't steamroll past this idea of the abstractions. We should really not just focus on the edges of the pyramid, which are the reports and the source data and the concepts, but we should focus a lot on that middle piece, making sure that the abstractions and the connections are good and really making metrics for first- class citizen. So I have so many other takeaways, but Juan, I'm going to pass it over to you.
Abhi Sivasailam: You mean I could have just said that in three minutes?
Juan Sequeda: Well, that's why when the podcasts come out, you can just listen to the takeaways part. You don't have to go listen to everything.
Abhi Sivasailam: ...
Juan Sequeda: One thing we talked about, I really loved about the metrics is they're durable, and then the entities, those we can change. I think that's also a very key takeaway right there. We need standards for what good looks like. That's another important one, because we don't know what good looks like. And I think when you're doing with SOMA, the Standard Operating Metrics and Analytics, this is a first approach towards that. You have over 100 business semantic events, like a customer renews a contract. And effectively what you want to go do is start to map your existing raw data into those activities and do that. And yeah, people have actually been trying to go do all this stuff before, but the thing is that all their sources, trying to automate this, but the sources are all very different. No two Salesforce implementations are the same. So you really want that translation layer to be semantic because the humans need to be able to co- create those connections. Well, it does open the question, will it make it hard to adopt these semantic layers that we have these metrics that we were defining? And you're saying no, because actually being prescriptive lowers that activation energy right there. And at the end of the day, this is all just triples, you put this into a knowledge graph, that's one way of how you can make this really highly semantic. So how do you break this arbitrary uniqueness force field? Well, you said marketing shows up, they say they have these metrics that they want. They want these dashboards. They already know what they want when it comes to metrics. No one is saying, " I'm super special. I won't do GAAP accounting." No. There's always even regulations requirements. So you need to have the carrot and the stick. And one example that came up was like, hey, VCs, they just need to standardize the way to tell their portfolio companies, " You need to go do metrics this way," because it's going to help them do benchmarking. So that may be the carrot and the stick to go do there. How do we manage people implementing this? Well, you guys are showing this right now in dbt. Then this can go into knowledge graphs and into BI tools and SOMA's very modular around that. So what's next for SOMA? You're starting with the B2B SaaS metrics and you're fleshing that out. And I think B2C and e- commerce are coming out. And then finally, I think what's interesting to close this back out with OKRs, and I think one of the reasons why this fails a lot is because the business doesn't even know how the business works, and we lack that North Star. So if we are very clear about what that North Star is, we can start decomposing it, fill into metrics, and then into the actual entities and activities around there, which forces us to really understand how the business works.
Abhi Sivasailam: Sounds great.
Juan Sequeda: How did we do? Anything we missed?
Abhi Sivasailam: I think that's it. I think the only thing I'm missing is my cocktail.
Juan Sequeda: All right, we're going to get that. So we're going to throw it back to you for three final questions.
Abhi Sivasailam: All right. More questions?
Juan Sequeda: Yes, that's it. Wrapping this up. Now, number one, what's your advice about data, about life, whatever? Second, who should we invite next? And third, what resources do you follow? What people podcast, blogs, newsletters, conferences, so forth?
Abhi Sivasailam: So I'll answer them in reverse order. So I think Data Eng Podcast is a good podcast, your guys' podcast is great. The Analytics Engineering Roundup is a great newsletter. I think Data Eng Weekly, Ananth Packkildurai puts it on, I think is also great. I think folks to chat with, lots. So someone sitting in the room right now is Ahmed from Narrator. And I think I was telling Ahmed this last night, Activity Schema was in some ways just ahead of its time. And that time might be now. And I think, for a variety of reasons, the rise of AI and LLMs that need a more natural semantic querying interface I think is a big one. I think the state of where data is and how it needs to be mapped, a lot of things that we've been talking about, I think thinking about your business as instead of entities and whatnot, as that first level of abstraction being a set of activities, and seeing your business as a set of activities that companies do and customers do, is I think, important, and I hope gets more traction. So I think Ahmed is a great person to have on, lots of others I can share, but maybe we'll start there. And then advice, look, the mandate for our data team is to build your growth model. And if you see your mandate as building and owning that growth model, I think it's transformative. I think you'll no longer have questions about, " How do I create value? Do I create value?" Your company will not have those questions. We talked about metric trees here. I see the goal of a data team as to understand that growth model, to help people understand it, but then to also, and this is very important, to identify new levers for that growth model. I think of a business as having growth levers. Data people should see themselves as using analysis and experimentation to find new growth levers. They're in the process of growth lever discovery, growth lever verification, so that operators can pull those levers. That's how a business grows. And if you are engaged in that cadence, there will never be questions about are you creating value or not. But you need to get into that cadence. And so everything we've talked about today are ways to grapple at getting into that cadence.
Juan Sequeda: That's the best way to finish this. That's excellent advice. I'm glad Ahmed is here because I've been wanting to have you on the podcast, so it's really cool we're connecting here. And with that, just a quick reminder, next week we have Benny Clive Benford, who is the former CEO of Jaguar Land Rover. Really excited about that conversation. If you're not following him on LinkedIn right now, you are truly missing out about all the stuff that he's been talking about, just driving value from data teams. And with that, Abhi, thank you, thank you, thank you so much. Thanks, Tim. Too bad you're not here, because we're going to go out for a cocktail now. Thanks to Data. world, lets us do this every single Wednesday. Been 130 episodes, I don't know, almost three years. Three years soon. Thank you.
Abhi Sivasailam: Thanks folks. Have a good one.
Tim Gasper: Thanks, Abhi. And enjoy the ...
Speaker 1: This is Catalog & Cocktails. A special thanks to Data. world for supporting the show. Karli Burghoff for producing, John Loins and Brian Jacob for the show music, and thank you to the entire Catalog& Cocktails fan base. Don't forget to subscribe, rate and review, wherever you listen to your podcast.
DESCRIPTION
Metrics are one of the most important and durable primitives in a company. And if data teams are looking to create value FAST… they have to learn how their businesses work.
To figure out how you business works, you have to find the right starting point; back to the METRICS.
Join Tim, Juan and special guest Abhi Sivasailam from Levers Lab on this week’s episode of Catalog & Cocktails to hear more about the most valuable use cases.
Today's Host
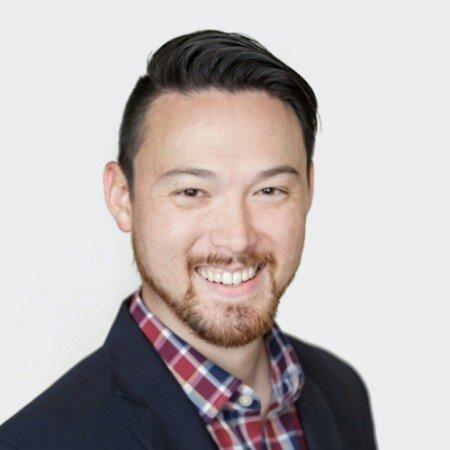
Tim Gasper
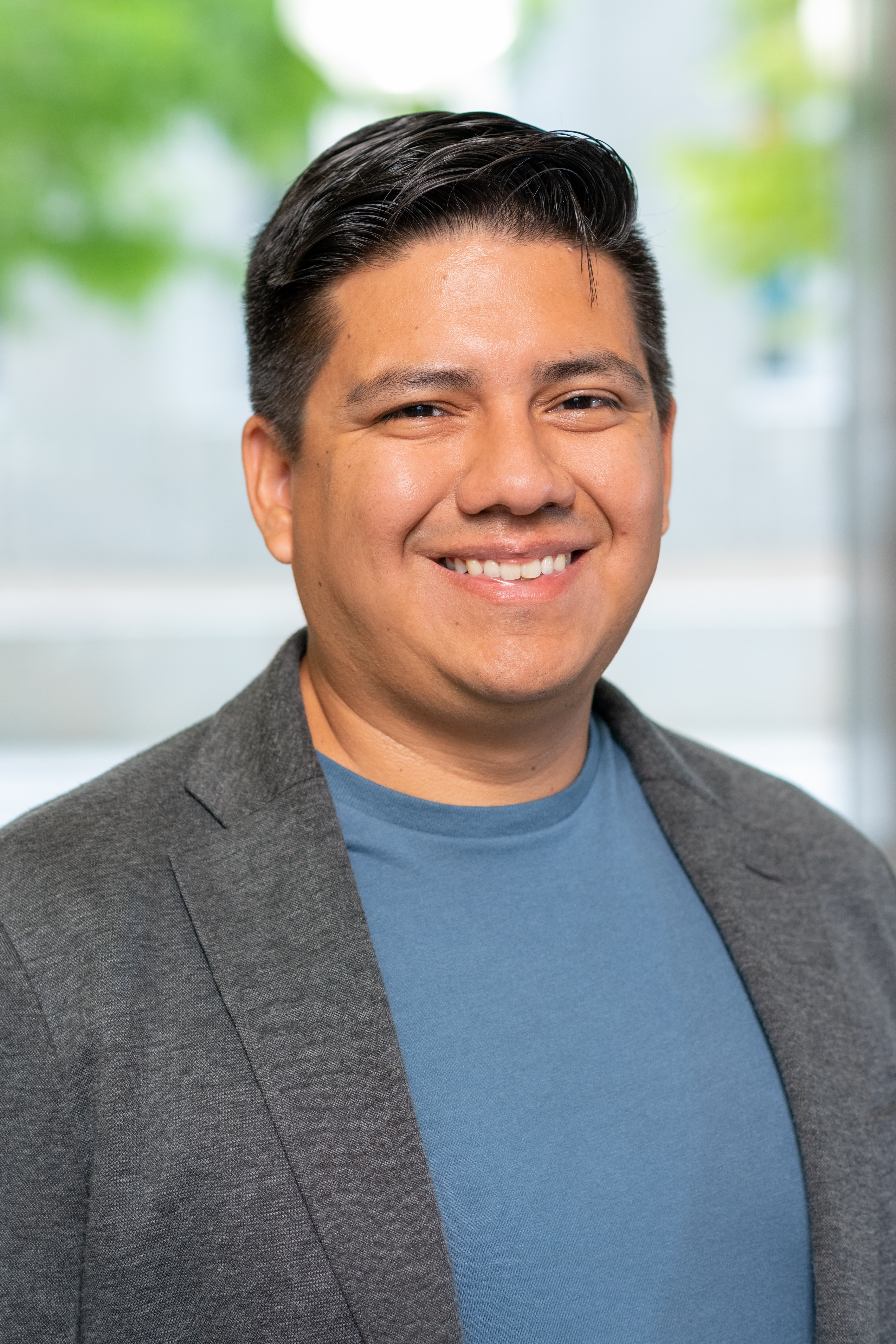
Juan Sequeda
Today's Guests
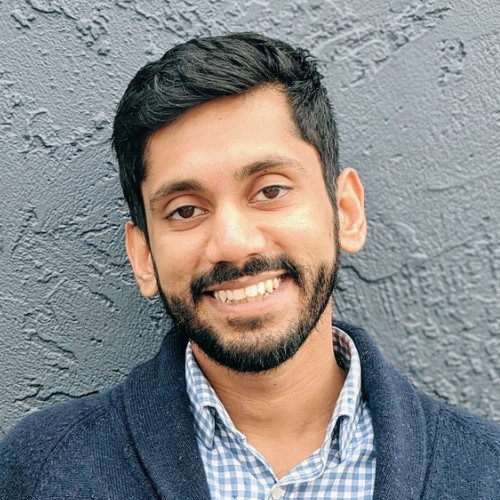