Everywhere you look, there it is; entity resolution w/ Jeff Jonas
- 0.5
- 1
- 1.25
- 1.5
- 1.75
- 2
Speaker 1: This is Catalog& Cocktails presented by data. world.
Tim Gasper: Hello, everyone. Welcome to Catalog& Cocktails. It's your honest no BS non- salesy conversation about enterprise data management presented by data. world. I'm Tim Gasper, longtime product guy, customer guy, and data nerd at data. world, joined by Juan.
Juan Sequeda: Hey, everybody. I'm Juan Sequeda, principal scientist at data. world. As always, a pleasure middle of the week, end of the day, and that time to go just take a break and chat about data. Today, we're going to be talking about a topic that I always wonder, " Why don't we talk more about it?" Because I always believe it's everywhere, but it's something that we don't talk about it. I think we got the best person probably on earth to be able to talk about this. I'm so excited to have Jeff Jonas here. He's the CEO of Senzing. He's a former IBM fellow, which I think was most amazing things that can happen at IBM and a renowned expert on entity resolution. Jeff, it is a pleasure and honor to have you as a guest here at Catalog& Cocktails. How are you doing?
Jeff Jonas: Hey, I'm great. I'm sitting in Brooklyn. Life is good.
Juan Sequeda: All right. Well, I'm still here in playground in Carmen. So, if things are loud, it's just because this is how life is over here. Where are you Tim?
Tim Gasper: I am at the Los Pines Resort Hyatt a little bit off east of Austin where we just had our executive offsite. It's very nice out here. Some pleasant trails and things like that. A little muddy though, but enjoying the Austin weather today.
Juan Sequeda: Awesome. So, we got our tell and toast. So, let's kick it off. What are we drinking and what are we toasting for today? Jeff, kick it off.
Jeff Jonas: Well, I am drinking a mocktail because it's still in the middle of my workday. It's a grapefruit oriented sparkling water. I do have it in a fancy glass with some cubes and I would be toasting to the beauty of adding more data to getting better answers and better decisions.
Juan Sequeda: Well, I'm going to go for we need to eliminate the garbage in, garbage out thing. So, I think this is all about let's really start understanding the value of the knowledge around the data to make sure that we have really beautiful data to make better decisions with. I'm having a piña colada today. Actually, I don't think I've ever had this cocktail here during the show. How about you, Tim? What are you up to?
Tim Gasper: It's pretty good. I've got some vodka and Sprite over here. So, keeping it very, very simple but enjoying the libations as well. So, what should we cheers to?
Jeff Jonas: Data. More data good.
Juan Sequeda: Very much more good quality data. That's for sure we need.
Tim Gasper: Well, Juan, I want to comment on that. Oh, wait, we're going to do cheers now. Okay, cheers. Juan, what if I said to you that errors and natural variability in the data actually made systems smarter? Let me give you an example of this, okay? When you search Google and it says, " Did you mean this?", it's not looking in a dictionaries. It remembers people's errors. Similarly, in MySpace, natural variability, misspellings, my brother's name's Roadie, but some people don't know how to spell that. They haven't seen it. So, they misspell it. It turns out those errors in the data later can become your friend. Bad data good.
Juan Sequeda: I think they're observations of what's occurring in the world and then they can be interpreted in different ways and they can be interpreted as an error, but then at the end of the day, this is what actually happened and then we want to learn from those things. I think we learn from what others can consider errors. So, I think that is a very good accurate point that there's a lot to be learned from errors.
Jeff Jonas: By the way, I see the world as filled with observations. What we observe as humans and what systems observe, what they collect in their eyeballs, so to speak, is observations. By the way, another example of bad data good is I have a son that has two dates of birth. It's a bad daddy, very embarrassing story. Okay, but I'm going to tell you really fast. I know I'm forcing on you and you can start the whole thing. Okay, get this. My kid's born on September 2nd, but I in my head get it wrong. I convinced the grandparents, the mom, everybody, that it's September 5th. We celebrate this kid's birthday on September 5th for years. Okay, now, he's like five or six. I get his birth certificate so I can take him to Mexico. To my surprise, his birthday's wrong. First you go, how do you sell it to your kid? You teach them their birthday. Now you're like, " I got it wrong your whole life." But imagine if you put the same birthday in a bunch of systems, healthcare systems, Ricky Rick Magazine or whatever, and five years later, you see one bad date of birth. How does ever dissent fester and become dominant if every system in his right mind cleaned out the one? Because there was 200 pieces of evidence about September 5th. So, it turns out this is another case where bad data can be your friend because you have to let it fester. Okay, fine. Enough about bad data.
Juan Sequeda: Oh, no, we got more of this to go, but all right. Well, actually, this is a great segue to our warmup question, which is what is something you thought to be true in life that ended up being false? I just realized that maybe you could only have one birthday, but apparently, yeah, you can have two.
Jeff Jonas: Plenty of cases actually, where bad data can be your friend. I mean, do you want me to really take a shot at that or should we just-
Juan Sequeda: Yeah, please, please. Yeah. Something profound.
Jeff Jonas: I would've thought in my maybe early twenties that you get married and you just stay married and you'd be married. But now that I have three ex- wives, now I'm a good ex. It now to me relates to the thing I've been convinced out of is relationships are arcs and they have beginnings and middles and ends. You do them as long as everybody's winning.
Tim Gasper: That's very deep.
Jeff Jonas: This is the data story. The data story is I have a lot of data on that with my ex- wives. What'd you say?
Juan Sequeda: How about you, Tim? What's yours?
Jeff Jonas: Yeah. What were you going to say, Tim?
Tim Gasper: I was just saying, I think that's deep. Relationships are an investment and they go in all sorts of different directions. You know what? I always think that words mean certain things and then I find out later that they mean something different. I'm terrible with the purity of semantics and what they mean. I know that's a generic thing. I can't think of a specific word because I probably don't have it at the tip of my tongue. It's part of the problem, but I mess up words all the time. So, that's stuff that I think is true and ends up being false.
Juan Sequeda: Words matter, but all right, let's dive in here. So, Jeff, honest, no BS, if entity resolution is everywhere, why don't we appear to really realize it?
Jeff Jonas: Well, I don't know, man. I struggle with this. How come it's so buried? Part of the reason I think is it has so many names, it comes in so many forms. Some people heard of match merge in marketing or database marketing list of pen where you're adding some new fields. You had the entity. You have to match it to something. Link detection, fuzzy record matching. Oh, man, there's a lot, patient record matching. There's like 50 terms for it, but it sits underneath and it's the bane of analytics, because is it really three people or one? How good can analytics be if you can't count? The entity resolution is really counting your entities. How many customers do we really have? By the way, you have duplicates in your phone. I bet you have a few dupes in your phone. That's an entity resolution problem. That's crazy, man, because you're the only curator. Imagine that you're the only curator. There's dupes in your phone. Imagine you're a bank, an insurance company, social service agency. They have all these people entering stuff. Man, they got you think you got dupes. It's everywhere.
Juan Sequeda: This is a fascinating definition. Entity resolution is really just about counting your entities, knowing how many entities you have. I think that's a very clean, crisp definition right there that we need to be able to understand. So, it's not just linking things together, but also duplication and so forth around that. There's so many different names. I mean even if you go to the Wikipedia article about anti- resolution, all these different names that this thing has. So, that's definitely one of the things. But if we look at the tech stacks, all the modern data stacks, everything out there, there's the databases, there's the analytics, but there isn't the tool that people are buying for entity resolution. Why is that the case?
Jeff Jonas: It's how ETL flows. Most people are trying to build it themselves, but it's not obvious to folks that you could spend$ 20 million in five years and have something that's still not very good and not very competitive and definitely not agile and it doesn't let you to pivot into new markets. Then you add some new data sourcing and get some new features, and now you got a Twitter handle and you got an IP address on this and that. Now you have to go back to your data scientists and they studied the dark art of any of the resolution and they will work for you the rest of their lives. That's like the state of the union. But the headline is if you can't count, you can't estimate and predict. Whether you're trying to do marketing or risk score, whether you're trying to figure out whether you should sell them something or shoot them with a laser from space, man, if you can't count your entities, you missed the obvious resources.
Tim Gasper: I think that's a great way to simplify things. To riff Juan off of what you were saying, people talk about a lot of different concepts when they think about this area of entity resolution, record matching, link detection, fuzzy matching, duplication. Do you see those things as all being within the umbrella of entity resolution? Do you see them as being different? How do you think about all these different terms and how they all relate?
Jeff Jonas: It's all the same thing. Whether you're finding duplicates in a single data source and call that a vertical dupe or you're trying to combine it with secondary data to get matches across there because you're learning new columns or how many records in this system are the same and that system, you're all just trying to figure out how many unique entities. Another way to view this is every pile of data in an organization is just different pile of puzzle pieces. The blue puzzle pieces over there, it's in the CRM, and the red puzzle pieces are over there because you've done some investigations. Then the gold puzzle pieces over there, your high rulers and the blah blah, blah. The question is, can you figure out how they all relate to each other? If you can't see that, these people building machine learning models on data that's not been properly resolved, I'm telling you these models, they think you have three customers when it's one. Hey, crazy funny story. I know somebody that that wasn't my stuff, because, well, that would be bad, but they had some bad entity matching and they put somebody on the quarantine COVID bus, but it was a bad match because they're supposed to be on the green bus, not the red bus. After five minutes, they were on the right bus because they were exposed. They have to live alone there. It's been two weeks in quarantine.
Juan Sequeda: Ouch.
Tim Gasper: That's a bad mistake.
Juan Sequeda: All right. So, let's go into the technical side because I think for one perspective, actually, it was a conversation we had last week with Malcolm Hawker who actually called you out as the next guest. Hey, guess what? He's actually-
Jeff Jonas: Oh, great.
Juan Sequeda: So let's talk about the history and we're looking at MDM, master data management as the traditional... I always call it the old school approach of just managing all your records and creating that" single version" of the truth type approach. Where are we right now within technology? Let's talk about technology. How have we been doing this today? What is the limits of the technology that we are today and what are we hoping for? What is missing and how are those gaps being filled?
Jeff Jonas: Well, on the long arc of time since I've been building at any resolution systems since I was like 22 years old and then built five different generations and then sold fifth gen to IBM and then had a vision, woo, vision on how to build another engine, a general state of the union is it's very expensive. It's complicated. The results are pretty iffy. It's very hard to do yourself. Some of the evidence of this, by the way, is there has been three quarters of a billion dollars in venture capital or growth funding in the last five years for entity resolution center companies. I know single companies that have raised a quarter of a billion dollars each. I know two, quarter billion each that have very strong entity resolution. That's expensive. Then you can go on with other list of names, but there's an enormous amount of money that's been going into it. But the state of the union is, for the most part, it's very expensive, very long projects, and it's hard to get a lot of joy out of it. I mean, my purpose in life has become, it wasn't this a while back, but it has become this. Can we not just commoditize it so it's available for everybody? To get the good stuff, man, if you don't have at least a million dollars. I just heard today that somebody said they got their false positives out of their banking system, but the project was nine digits. That's$ 100 million or more.
Tim Gasper: That's an incredible level of investment. That makes me wonder. People talk about, for example, master data management and I know that you can invest in a master data management software tool set for quite a bit cheaper than nine digits, but it seems like that some of these projects are at a very different scale. Is that true? Some of these really massive and challenging entity resolution problems, are they a very different class of problem than master data management type solutions? Actually, is it a slider, a gradient?
Jeff Jonas: When you get to systems that have a billion records, a billion identities, name, address, phone, that's a record. Not counting all the names and all the addresses and all the phones. Each identity is a record. When you get into billions or tens of billions of records, how to make those systems scale and stay accurate, how to make them real time so you don't have to do a batch reload. How'd you like that? You have 10 billion records loaded. You don't want to load 10 new records. You're like, " We'll just reboil the ocean." Well, that is not green. So, as you get to larger scales and you can't all do it in memory, your techniques have to change. But what the history has been is it's been pretty expensive, million basically minimum to get into really decent entity resolution. You can easily spend 5, 10 million, or more. The question is, what about everybody else? So part of what I've been trying to do is create something like the smallest little nonprofits can use for chump change and still have not just the elite. It's not just for the elite. Everybody can do it.
Juan Sequeda: This is an interesting point that entity resolution is for the elite. To get it right, you have to invest a lot of money as we're talking here. Otherwise, you just don't do it or you do a crappy job about that.
Jeff Jonas: Yeah, yeah. If you want to buy entity resolution, it's for the elite. If you want to work on it yourself, I mean, I know organizations with 50 people, 100 people. I know some organizations with hundreds of people that are working on entity resolution. It is that hard. It's crazy.
Juan Sequeda: From a basic perspective, you see people doing stuff in SQL and doing some fuzzy match. I mean that's basic stuff and maybe that gets some stuff there. If you're a small organization, you only got 50 people, you still got customers, maybe that's the best you can do because you can't afford... It's a problem, but not a big enough problem that's going to warrant more investment on that. But then it seems that at some point, the stuff that you can do yourself just breaks and then there's this gap. There's either you just live in this really bad world until you really need to invest a shit ton of money to go do this correctly. There's this big gap right there. That's how I've been seeing.
Jeff Jonas: Yeah, that's true. If you want to get something to 70% competitive and accurate, you could do that for a million bucks. You want it to be like 80% towards a highly competitive product, you might spend$ 5 million. If you want to get 90%, it's not quite world class, not type leading, but you want to get the 90%, spend $ 30 million literally. It's crazy, but what's going to happen, it's going to get harder and harder for organizations to compete without higher quality entity resolution. Between the false positives and the false negatives where you're tapping on the wrong shoulder or looking stupid to your customers. I show up at a hotel. I go to check in. Hey, we didn't have your loyalty club. So, I tell them my name. They go, " Which one of these is you?" They named three people. They're all me. I have three. I'm in their club three times and they can't even fix it. It's crazy.
Juan Sequeda: You're not making a pretty picture of the world. I mean you're saying that we're screwed.
Jeff Jonas: Well, but the truth is that a lot of decisions are being made and there's a lot of waste and a lot of bad decisions being made because people aren't counting their entities right and it deserves some invest. They should either be investing in it, buy or build. Do you think there's anybody out there that's still building their own spell check, grammar checker? I mean they got their data scientists and they are fascinated with the art of doing grammar and spell checking themselves, or do you think they're just using a library? That's what I'm trying to do. Are you really kidding me?
Juan Sequeda: That's a great analogy right there.
Tim Gasper: Go ahead, Juan. Yeah.
Juan Sequeda: Are we at the point of commoditizing entity resolution that can be the equivalent of a spell checker that you just called?
Jeff Jonas: Yeah, that's what we're doing.
Juan Sequeda: But again, so what has been the missing piece? What are the principles that should be able to apply over and over again? Otherwise, it seems like everything just going to be a particular project for every single scenario that is not scalable to replicate.
Jeff Jonas: Well, I don't know. I built five different generations and I sold the fifth gen and then started a sixth gen, but I told when I was going to build it for them, I was like, "I need $ 50 million to build it." They're like, "$ 50 million?" I go, " Well, yeah, but I'm going to build a sixth generation and it's going to cost $ 50 million anyway, because it's that hard." But I don't know, you've got to see a lot of use cases to figure out how to build something that's generalized. Because it's real easy to really get really narrow around a certain entity with a certain set of features and study just that, but it took decades and a lot of different use cases to think about it. This has a little bit to do with it. I did a blog post a long time ago called Hell With Rules. The entity resolution space, a lot of times they're like, " Okay, if the names are this close, that's this score. If the address are this close, it's this score." Then they're like, " What about phone numbers and Twitter handles and Telegram handles and blah, blah, blah, blah, blah?" Oh, well if this and that are true and this and that are true, you get these growing combinatorial lists of if these things are true and those things are false. The amount of details and rules that you get just grows and grows and grows. I want know if this lady had a financial company. She goes, " Hey, I just want to impress you. I know what you are. We have 10,000 rules." I thought to myself, "10, 000 rules?" She's bragging at me about 10,000 rules. So, to trivialize all of this, it took me a few months to come back at her with the difference between rules and principles. So, under Senzing, we built a principle based system, but it basically goes like this. Your kid's throwing rocks at cars. They're like, " Hey, don't throw rocks at cars." But the next day, they're throwing sticks at buses or rocks at buses. You're like, " Okay, don't throw rocks and sticks at buses." The next day, they're throwing them at SUVs. Your kid's 35- year- old throwing iPads the same way. How many rules do you need if you can't get a principal going?
Tim Gasper: Is that part of the magic here? Think of it from a principal standpoint. How do you even do that? Is that applying more of a semantic understanding?
Jeff Jonas: Well, there's two parts. One is principles. I'll give you an example of a really simple principle as if somebody's identifier like a passport ID, Social Security number, or in a car, the number or license plate number or a router, serial number, IP address. But if the name is pretty close and the identifier's the same, you're probably good to go. Okay, that's a principle. You don't have to name all the identifiers in the world. Socials, Mac addresses. Okay? So that's the principle. But then Pirates of the Caribbean number one where the lady's on the ship and she looks at the captain and the captain goes, " We're going to make her walk the plank." She goes, " I invoke rules of parlay." The evil captain goes, " Actually, those are guidelines." If you don't want AI, because we have a real time learning thing, but if you don't want it to go crazy, put some tape on the stop sign. So, it thinks it says 55. Even though it doesn't say 55, it just goes crazy. You have to operate good guidelines. So, we have a principle, simplest one. Hey, if it's an ID number and a name's pretty close, call it good to go. But the guideline would be unless that ID number's oversubscribed. If you have 50 people that has the same passport, like 123123123, that one's not any good. Okay? So you get to a self- tuning system. The trick in all of this though is you've made a billion decisions and now you get record billion and one. A record billion and one, you learned that the passport number of 123123 is no good in that moment, you have to say, " Now that I learned that, had I known that in the beginning over the billion records I've seen, should I've made any decisions differently." Any smart system should be able to change its mind about the past, real- time learning. By the way, do you ever do this? You're listening to somebody chatting and you go, " I know what they mean." Then they say a few more things and you go, " Oh, now, I know what they meant." That. If you want to do real- time streaming learning systems like what we're doing in entity resolution, that means every record you get, now that I learned this, I learned something about the past. Oh, those two people aren't the same. That's a junior and senior, but it wasn't known until this new observation. That's the hard part by the way. Anyway, I'm blah, blah, blah.
Tim Gasper: No, I mean, that's interesting. It's a different frame of mind and sometimes it's not always a step forward, right? Because you come up with a new principle and you say, " Oh, wait a second. This is a different way than I should have been thinking about this." But maybe your past heuristic was actually better at being a predictor. Now you've got more work to do before you can get back to where you were. So, it seems like a hard problem on two fronts at least.
Jeff Jonas: What we are seeing is that I just did a blog post about this recently because it just made me crazy, man. It just made me crazy. They wrote what I would call small data set, and then they want to tinker a little. I said, " No, just keep loading more data, because it can change its mind about the past." More data remedies tinkering. By the way, the kinds of tink you're doing, it might look really good in your small data set with 10 million records, but as you really scale up to your billions of records, those things you're tinkering on, you're not even going to want those later. It's just more evidence points, but it's like how do you integrate new observations into what you know? It's like a puzzle piece, man. A new puzzle piece shows up and you're trying to figure out where it belongs in the puzzle. Just like when you put a puzzle together at home, sometimes that one piece allows you to realize these two pieces or these two chunks are connected. That's the mental model I have about what's happening inside of... I'm going to take it up one level, a context engine. Context, meaning understanding something by taking into account the things around it. You see the word bad in the sentence, you look at the words around it. Data doesn't sing unless it's in context. It doesn't tell you really what it is unless you can find the related pieces. Entity resolution is the first form of that.
Juan Sequeda: Oh, man, this episode has so many awesome quotes. Data doesn't sing unless it's in context.
Jeff Jonas: I just made that up, by the way. Should I drop a little TM on there?
Juan Sequeda: Yeah. Yeah. I mean, I will be quoting you on this one. I mean we're going to get a bunch of T- shirts out with quotes like this. That's definitely going to be on that one. All right. You've got my brain here thinking. So, couple of things. One is the lady who said they have 10, 000 rules, how many principles are there?
Jeff Jonas: Here's my goal and it's all imperfect because that's the world we live in. My goal was that the number of principles would fit on a screen, a single screen. You could look at them all at once, not font size six, and that the set of principles work, whether it's people data, company data, vessels, planes, routers, cars, same set of principles. It'll work in English, it'll work in Arabic, Mandarin, no changes. That was the vision I started out with. How I did it, by the way, when I thought about this new invention, I'll call it this new method. It's radically different method. By the way, I originally called this project G2, not for gen two, genus. It's a different species. If you look at my first five generations, you can see how it grew up. You'd be like, " Oh, look what it did to the schema." Well, that was nicely normalized that and made that a type value pair. Cool. You could see it grow up this new thing. It's just radical. What I did though is I made columns. I had companies then cars and routers. I made examples of all the features of each, people, names and addresses and phone numbers and date of births, cars, make, model, VIN, color. So, I listed the common features for these. What we've been doing in entity resolution is you think about each domain uniquely. You look up and underneath that column and go, " What combinations of things should I drive these things together?" Then you look over at cars. You go, " Okay, good. VINs are always pretty good, make and model. Those never change over our lifetime." What I did is I thought about it horizontally. I said, " What is it about us as humans that..." We can do entity resolution in new domains all the time. I said, " What is it about a social that makes it like a VIN? What's a VIN? How's that make it like a Mac address on our router?" It led me to behaviors, just a few behaviors. So, you can pick a new entity type and you can just pick new features and you assign the features one of three behaviors, and you're done.
Juan Sequeda: Okay, but there has to be a number here.
Jeff Jonas: I've got like 26 or 28 principles. By the way, now and then, we add another one or change one. But when we do, it's good for everybody. It's really weird. I can fix it, make it match vessels better for the Singaporean Maritime project. It makes it better for somebody doing corporate supply chain data with corporate data, with corporate hierarchies. That's how I know I'm on the right track. Okay.
Juan Sequeda: Let me get a little bit philosophical here, because I completely agree with you. What we're talking right now is for me, this is a demonstration that why computer science is actually a science, because what we're seeing here is that you're observing a phenomena here of computation. If I look at this thing that I go down here, it's always this. I mean, VIN, make, model, year, name, address, all these things. But then what you observe here is there's a higher level abstraction which applies to all these things. Then after seeing so many times over and over again, you realize, " Oh, this is not applicable. This thing that I just observed is applicable to cars, to peoples, to vessels, to routers and so forth." I think something that we learn in computer science, for me, actually, computer science is all about understanding the levels of abstractions. You figure out what level of abstractions you're going to be in. Maybe you also become a compiler. You like to focus and I want to move between one level of abstraction to another. So, for me, everything you're saying is this is the phenomenon of computing. We are understanding the different levels of abstraction and we're solving a problem. If you had a higher level of abstraction, the problem actually gets a little bit easier. Now the thing is have that insight to be able to realize, " Oh, we should look at this problem at a higher level abstraction." Hence my question, at a lower level abstraction, we're talking about 10, 000 rules. At a higher level abstraction, we're talking about... Well, you're saying 26.
Jeff Jonas: Principles or something.
Juan Sequeda: Which in a way, there's some a rule here because you're saying identity can be considered by X and Y. X here can mean name and address and X can be make and Y can be model and so forth.
Jeff Jonas: Another way to think about this is sometimes you go to solve the problem, but a way to get it more abstract is to say, " What kind of thing can solve problems like this?" Instead of solving that, you're saying that's an example of a classic problem. Can I build something that can solve things like that?
Juan Sequeda: Again, this is computer science. You got to want to reduce a problem to something else. This is why this is so fascinating.
Jeff Jonas: By the way, about catalogs. A thing about catalogs, one of the ways I think about what we do and what we do in entity resolution is we have a little database, but it's a special index, but it's like a card catalog at the library. You're not taking all the data from everywhere in the enterprise. You're taking the subject title author off the library cards and you're putting it in a card catalog. That card catalog, you're rubber banding together the cards that relate to the same entity. Now you have a way to get a 360 degree view without having to move all the data. By the way, how you keep maps at the library versus DVDs versus magazines versus books, each of those aisles can have different structures. But at the card catalog is where it all comes together. I get my inspiration, by the way, did you know that there's a single part of the brain... If I said there's an artist who drives a motorcycle that has passed away not long ago, whose name was a symbol, you just did entity resolution to Prince. In your head, you may have located a picture or the Purple Rain album cover. Your hippocampus did that. The hippocampus is the library card catalog of the brain. I like to hang out with hippocampus researchers because I get the inspiration for my work on my engine because I'm really building a hippocampus. Wait, that's a secret.
Tim Gasper: I love that. That's the cross- functional knowledge going across disciplines where you get the new aha moments.
Jeff Jonas: Yeah, I'm a very curious cat. So, when I hang out with people, I track some down and I'm like, " Can I come hang out with you?" If you are a brain researcher and you sit next to me on a flight across the country, you poor thing, because I want to know about the details of that, especially that hippocampus.
Tim Gasper: I have a feeling that that would be a very interesting conversation to observe. You mentioned about catalogs and the context. How does metadata tie into entity resolution and how does that become important? Is it important to do things like catalog your rules and things like that, or catalog your principles or is that a little unnecessary? Really what you care about is more the end result of all of that?
Jeff Jonas: Yeah, it's a little bit unnecessary to catalog. Maybe something that sits in one place and it's very short. You could edge it onto a piece of paper, a small print. But if you think about a bank account that gets opened, because a lot of our customers are doing transaction monitoring or fraud or sanction screening or something. You wouldn't pass an entity resolution engine, all the particulars about the money moving here and there. Those are interesting for the rules and alerting. But the one type of metadata would just be, what's the name, address, and account on the phone and what's the identifiers of the entity on the other end. That's the metadata. That's the metadata you'd pass to an entity resolution engine is the features that you need to be able to assemble something and index it. But then of course, like the library, it has the Dewey decimal number. It has the pointer back to the actual transaction of the actual account. By the way, this is maybe an important. It's not obvious to maybe everybody that might be on listening is you have data that your observations, that are collected in lots of different piles and different forms. There's video from the parking lot and there's people onboarding in the loyalty club. But in the other end of the enterprise is decisioning systems that help focus human attention, driving it to showing a human or a feedback loop to a user or reporting. But in between is where the entity resolution happens. Entity resolution is you're taking your observation space and it's like lots of different puzzle pieces. You're bringing context to it. It's a form of assembly. It's context construction. So, it comes out of entity resolution as an entity resolved graph. Then you send that to decisioning systems. Good news, bad news, sell it or shoot it. That's downstream from entity resolution.
Juan Sequeda: So I'm still at the principles. I'm hearing everything you're saying. I'm like, " Okay, you've observed so many things throughout all your different generations of entity resolution systems you've built and you've identified these principles." I'm hearing this. I'm like, " Okay, there is some what I would call an upper ontology, some semantics that is applicable across so many different domains where you're representing things as, I mean, high level obstruction identities and stuff like that." You have a thing that can be identified and so forth. Then you have rules associated to that.
Jeff Jonas: Well, let me say a few things. I love this because it's a conversation like this that helps me think about when I say some words, how do they really land? At the front, I'll just use an entity engine, let's say somewhat like ours. It said you get some message in you. It's got a tag on it. This is his name. Here's the name. Maybe there's an AKA, address, label. It's a label value label. Value label value. Some data sources, the address is a bag of words, like a banking swift message is a bag of words. It's just a bag. Other address is 1234 or address one, two, city, state, zip. So, different sources have different assemblies of those, but ultimately, those are tagged as addresses. What entity engine needs is it just needs to know that's an address and that's a name. It should just take care of that and do the right thing with it. Now, but one organization might have some features that weren't conceived of. Maybe somebody's doing a project in South Asia or in Malaysia. Now they need an Asian citizenship ID as a field. Well, they should be able to introduce that. They should be able to introduce a new field without having to restructure a database and everything.
Juan Sequeda: So there's still work that needs to be done about mapping, the guessing systems to the principles you have.
Jeff Jonas: Not the principles. You don't map the principles.
Juan Sequeda: Not to the principles.
Jeff Jonas: No, no, I'm going to clear it up really easy.
Juan Sequeda: All right, clear it up.
Jeff Jonas: For every entity, there's a list of features, name, address, phone, cars, make, model, color, whatever. Okay. What you do in a principle based engine like Senzing is for each feature, you just tell it with just three principles. I'll tell you what they are, man. I'll take all the mystery out of this. The number one principle or I'll call it behavior, the way you do principles is you map the features to three behaviors. One of them is frequency. Remember these are guidelines like Pirates of the Caribbean, okay? So it is not like what is it? It's like generally. Generally, frequency is generally when you see this value, does it relate to one entity, a few, or a lot? Date of birth. When you see a date of birth, does that value generally relate to one person, just a few, or a lot? A lot. So, you give a frequency mini. A home address, frequency few. If it's in your email or cell phone or a social security number, a frequency one. Again, it's just generally. That's one of the behaviors. So, jump to vessels. Vessels have what's called an IMO number. It's stamped on the hall, man. It's like a VIN number on a car. Frequency one, if you see an IMO number, it's probably that vessel. If you find 50 vessels that are all 0, 0, 0, 0, 0, 0, it means that one isn't so good. The second behavior's exclusivity. When you see this feature, do any of this tend to have more than one of these or just one of these? A car that has a make or a model, how many makes or models can a car have? Have you ever seen a car that's two models? No. So, it's exclusive. A social security number, a person generally has one, but not a credit card number. So, a credit card number is a behaviors of frequency one, but not exclusive. But date of birth, you're only supposed to have one other than my bad daddy story. The third behavior is stability. Over the course of the life of the entity, it tends to say the same. A credit card number doesn't, because somebody steals your credit card number, you got to cancel that little sucker and get another one. But your social security number does. So, a social security number is a frequency one, exclusive, because you should have one and it tends to stay stable. So, my rules, I call them principles. My principles aren't about the names of the features. It's only about the behaviors. The principles are, if things have identifiers but have nothing else that disagrees, now you can use the same set of principles. I remember I dreamt this up in my head with this exercise and my number two, been working with me for years. I went off to Singapore to work on a project, started putting vessels in there. Remember the day he called. He goes, " You're not going to believe this. We're doing really good matching on vessels and we didn't have to change any rules." I was like, " Okay, fine. Don't fire me."
Juan Sequeda: I was getting this wrong. I truly thought that you were doing things more... I have a higher level abstraction of the features to some description of what an identifier could be for multiple entities. You're looking at this more from the distribution of the data, right?
Jeff Jonas: Yeah, that's exactly what it is. Then in real time, I mean real time, thousands of transactions a second, we have a statistics table on the distribution. Not how many times have we seen a date, but how many entities have that date? Not how many times have we seen the phone number, how many entities have that phone number? Then that's at absolute real time where we keep those counts for every feature. Then as it's ingesting, if you have something that's a frequency one, but it's behaving like a frequency, fewer frequency, many, it just self- corrects it. Not just corrects it when it sees it, but the future, it goes back in time and goes, " Oh, well, I know that." George Foreman named five of his boys George and one of his daughters Georgetta or Georgina or something. You don't know that going in. But over time as you get more data, you discover these glitches in the universe or where the guideline's not true in this case. Then you want to be able to just change your mind about the past. You don't have to reload.
Tim Gasper: Interesting. When you talk about these principles, one of the things that I wonder about the state of art of entity resolution is how much can work out of the box? How much needs tailoring to your business or to your own policies and things like that? How much of it is domain specific? I need to understand insurance or health-
Jeff Jonas: It doesn't matter.
Tim Gasper: ...domains, things like that.
Jeff Jonas: It doesn't matter.
Tim Gasper: How does that spread look?
Jeff Jonas: It's so different now. If you asked me 10 years ago before this latest sixth gen different species that we've been working on, I would say at least 80% of the people that open us out of the box don't have to change a single switch. I'm talking about voter registration, modernization to healthcare, this and that and fraud over here. We just had a great project. One of our partners, Code for America, used our engine for a project to the Clean Slate Law in Utah. There's a law. There's certain kinds of felonies. They get rid of those. So, they're not felons anymore. So, they can vote and get access to better jobs. It's a small little resolution. You're probably all running memory, 80%, man. I'll just run the same without any tinkering. If you do need to tinker, see, this was a big lesson by the way. Back in the day, my fifth generation engine, it would be like this. You would say to me, " Is it configurable?" I'd brag. Here's what I would do. I would open up the cockpit on the 747 or the Dream Liner, whatever, and I would show you all the buttons. I'd go, " Marvel at the configurability." You know what I'm telling you? I'm telling you the manual's 2,000 pages. You need 2, 000 hours in the co- pilot seat and that you can't commoditize something if something that's complicated. That's been the state of the union. The principles piece is a way that generalizes until you can do different missions with different features. Now we've been specializing in people and company data, but we've had others do other entity techs, but it can be a little more work. But anyway, that's the vision is you shouldn't have to tinker it. If you do need to tinker, I'd be surprised if anybody had to flip more than five switches. I mean maybe if you have to tinker, it's maybe two. Usually, they're sorry. Later, they'll come back and go, " Wow, you should've just left the default."
Tim Gasper: I think that's exciting. This is a sign of usability and maturation takes an interesting path where things get more complicated and then at some point, ideally, they get simpler. That's cool to see this finally taking and hitting that inflection point.
Jeff Jonas: Back a year ago or two, I'd be like, " All you got to do is put it in your mouth and chew." Then I realized people want it easier than that. Now I tell my team, I'm like, " I need it dissolved under their tongue. You hear me?" They just put it under their tongue and it dissolved. They don't even need to chew. That turns out where a lot of the work is now is just how to make it easier and less and less work. On the mapping side, if you have to tell people they've got to parse and figure out the first name from the last name and it's one bag of words and now they have to split the name, that's a lot of extra work. So, we have a machine learned algorithm that's been trained on 850 million names. No, you give it a bag of words. It not only knows first name and last name, it knows an Arabic name. If it's an Arabic name, it knows Ben and Haja aren't part of the name. By the way, if you're going to match a Chinese name versus a name from Ukraine or Russia, you'd better use an entirely different algorithm, man. So, you guys know the culture of the name and then matching the culture. You spent tens of millions doing good name matching if you want to be a global company. It's crazy.
Tim Gasper: Just before we go to our lightning round here, ChatGPT and a lot of this stuff that's going on here. Exciting stuff. Super clever. It's like the smartest, dumbest thing you've ever seen, right? Just curious about what do you think about that craze and is there any relationship to entity resolution now or in the future?
Jeff Jonas: It might not surprise you, but it turns out ChatGPT and things like that are going to need to be able to have counted entities. I've seen two people now go ask ChatGPT to give them a bio. It sends them to school in places they've never been. They got a PhD in Oxford. It turns out they're going to need entity resolution. They're going to need a resolved entity graph to get to their next level.
Juan Sequeda: So it's not there. It needs it.
Jeff Jonas: It's everywhere. When you put entity resolution glasses on and look around, it's in AML, KYC, insider thread, CRM vendor, supply chain, nonprofits. Just everywhere.
Juan Sequeda: This is a good segue into our lightning round question because I got a couple things here lined up. So, all right. Let's move to the lightning round, which is presented by data. world. I'll go first. In the next 10 years, will we have democratized entity resolution?
Jeff Jonas: It's done.
Juan Sequeda: Oh, it's done. Not yet. Okay.
Jeff Jonas: It's done. I'm telling you it's done.
Juan Sequeda: We don't need 10 years.
Jeff Jonas: No, I mean more can be done, but I'm just telling you that's done. I get people asking my roadmap, I'm like, " You don't use half the stuff we already do." My Swiss Army knife has got so many things in it. No, done. Next.
Tim Gasper: That's exciting. Hey, second lightning round question. This actually harkens back to our previous episode where we talked about master data management. Is MDM dead?
Jeff Jonas: No, but it's caused so many bruises and limps and hobbles that it causes a lot of angst when people think that they might have the next 3 million project that goes two years and then gets canceled. But MDM's really about getting your entities better managed. It's a little bit of a falsity to say there's a single version of truth, but being able to properly count your entities to know how they represent each other through your observation spaces is as essential as ever.
Juan Sequeda: All right, next question. So, you mentioned data scientists, they don't build spell checkers. They grab a library. Will entity resolution just become like that, a simple pluggable library?
Jeff Jonas: Done. We literally shoved our 50 million investment to six gen into a DLL or a shared object in Linux where most of our customers do this. Done.
Tim Gasper: That's incredible. I'm getting very excited now. All right. Fourth and final lightning round question, are we trending toward entity resolution? Not the fact that you can have a library because obviously that makes things very easy. But the art of entity resolution, are we trending towards it becoming harder as time passes?
Jeff Jonas: The trending will be there's a lot of really great data scientists that are spending time on something where they could spend their time on something that's actually more interesting and more valuable. For example, the risk scoring models that come after entity resolution to make higher quality decisions. So, the trend's going to be a change of allocation about where you put your smart people. I'll call it the mundane task. Who would have funk that it's so hard to match Beth to Elizabeth? Really?
Tim Gasper: I mean it's going to be a hard problem. As data gets more complex, et cetera, that's going to continue to make it hard. But if smart people are building great libraries that are democratized that allow people to do this stuff and people can spend their time on better, more valuable things.
Jeff Jonas: You know what? It's about outsourcing entropy. It's the second law of thermodynamics. The universe is trying to break itself into little pieces, spread out, and cool off. We use the food we eat and teams to fight entropy. That's what we're doing. You do not want to have your own team. Do you have somebody at your company writing your own credit card settlement? No, you would use Stripe. You outsource that entropy. Why would you be using your energy to fight that? That's what I'm trying to do in entity resolution. We're going to slay your entropy for you.
Juan Sequeda: This has been a phenomenal conversation. All I'm just thinking is I just want to sit. I think Tim and I were just back channeling. I just want to sit in the bar and just have a drink with you.
Jeff Jonas: You guys haven't had a single drink.
Juan Sequeda: I started with this glass full.
Jeff Jonas: Oh, hey, that was pretty good.
Tim Gasper: I already wasted all of mine.
Jeff Jonas: Really? How did I miss it? Okay, well, I'm impressed.
Juan Sequeda: With that, let's see how much we impressed you because it's takeaway time. So, Tim, TTT, Tim takes us away with takeaways. We've been taking notes while we were having our drink here about everything we discussed.
Tim Gasper: All right. Yes. Tim's takeaways. So, we started off with, " What is entity resolution?" You mentioned it's counting your entities and don't overthink it. It's really about looking at those entities, counting them. When we look at all these different things like linking, duplicating, matching, fuzzy matching, mastering, a lot of these things are actually ultimately talking about the same thing, figuring out how things are related to each other and counting them. We got into what's the state of entity resolution today. You really gave us a little history lesson that things have evolved and changed. They've obviously gotten a lot better. You've been at the forefront of a lot of innovation as things have gone from gen one to gen two to gen three and so on, but the past approach has always been expensive, complicated. The results are iffy. It's hard to do yourself. The biggest companies are spending big dollars trying to solve these problems so that they can move the needle a little bit and get just a little bit better. As you get to larger scales, more real time, you can't do it all in memory. The approach has to change and it gets pretty complicated. Entity resolution therefore has been for the elite. You mentioned, " Wouldn't it be great if it was free or open or more accessible?" The more common people and companies could take advantage and spend less time trying to solve this problem. That feels like a really good approach and a good principle here. It gets harder and harder for companies to compete as they get more of this duplicate data, complex data. The truth is that a lot of bad decisions have been made because we're not counting our entities. Juan, what about you? What were your takeaways?
Juan Sequeda: So several here. So, one, Jeff, you have been seeing this for so long. So, you have to really see all the use cases to know how to go build something that can work as a general platform. That's what you've been able to go do. I love this. Oh, I got 10, 000 rules. It's like, " Really? Is that something you should be proud of?" Another interesting here commentary is any smart systems should learn about the past and make changes based on that past for the future. So, that's a very important thing. How do you integrate the new objects as they go? It's like a puzzle piece, right? Oh, I think these two things go together, but another puzzle piece will come in and you'll realize, " Oh, they don't go together and so forth." So data doesn't sing unless it's in context. That is a quote I'm going to put on a T- shirt and I love that. This is all imperfect because we live in an imperfect world that we just have to accept that. The principles, if there is the main takeaway, it's about the principles. Don't throw rocks at cars versus don't throw stuff at other stuff. You want to be able to have those principles and what you've been able to observe over your career is you brought this down to 26 principles. I really like that there are really behaviors of the data that you're observing here. Talk about the three principles, frequency. Generally, when you see this value, does it relate to one entity, a few, or a lot? Second, exclusivity. Does an entity have one of it or many of these things? Stability, does it tend to stay the same? This is an amazing aha moment for me and actually looking at this as behaviors of the data. In the past, you would have all these configurations. We're very proud of the configuration. Go into my book, look at all the knobs you can go choose. Now, today, you should not be proud of that. Actually, we should probably offer two, five max. Probably when they'll come back, they should have said, " I should have left it in default." We brought up catalogs for a second here, and this is Catalog& Cocktails. I think entity resolution needs to have a database like the card catalog because you want to keep track of all the features here that can keep and provide some extra context, because remember, data doesn't sing unless it's in context and the catalog will have that context. Then finally, ChatGPT, it's going to need entity resolution around here. Otherwise, it's a lot of great creative BS. All right. How did we do? What did we miss?
Jeff Jonas: Man, you have summarized my life in just a few minutes.
Juan Sequeda: While I drink the piña colada.
Tim Gasper: The SparkNotes of Jeff Jonas.
Juan Sequeda: There we go. All right. So, to wrap this up, Jeff, back to you. Three final questions. What's your advice about data and life? Who should we invite next and what resources do you follow? People, blogs, conferences, books, so forth.
Jeff Jonas: I'll take that backwards. On Twitter, I like to follow Terrible Maps. It's really funny. On conferences, I like the Geo Conference and just a thing about both of those is where things are and when and how they move are the highest order bits. If you're trying to do really interesting analytics, geospatial data, it is super fluid for analytics. Okay. So, funny side on the maps. Then the Geo Conference where people are obsessed in geospatial. Those would be two go- tos. Danny Hillis. Oh man, that guy. Do you know Danny Hillis?
Juan Sequeda: So I would love an introduction, Danny Hillis. I know him from the Connection Machine back in the'80s, during the early expert systems and non- parallel computers and stuff. My PhD advisor was Dan Morran, and at the same time, they were doing all these parallel rule engines. Then one of the things I find fascinating about Danny Hillis is just how he's seen the future. Jump around afterwards, he founded me Meta Web, which was free based, which is the foundation of Google's knowledge graph. This is a guy who has seen the future and built it and been there. I would love to have Danny Hillis.
Jeff Jonas: I can make that happen. By the way, do you know about his 10, 000- year clock?
Juan Sequeda: I have heard about this, but don't know the detail.
Jeff Jonas: That's his invention. This is a clock that runs for 10, 000 years without hooking up to any power. Okay, and then here's a piece of advice I've never shared, at least not public. I've shared to a few people. As you go through life, if you're creating a group of people that hate you, that don't like you, in the back halls, they say negative things about you, don't underestimate the weight of that versus creating goodwill. You want the people that have been through your life on a journey to be in the back room when no one's looking, saying generally good things about you. I say that because I've seen two cases now where people didn't put care to that. You know what? It's like having a small army with people. They're just waiting with a ship to stab you. So, anyways, yeah, that's my advice. Makes the future better.
Juan Sequeda: Very profound. I like how we started and we ended here with very profound advice.
Tim Gasper: It came back to relationships.
Jeff Jonas: Yes, relationships.
Juan Sequeda: I've seen this comment here on LinkedIn. The final takeaway just to put the stamp here. Entity resolution is a thing that hasn't been resolved. Would you agree with that?
Jeff Jonas: Yeah. Well, any entity. Yeah, probably an entity. It's still a real world entity to be entity resolution, but whatever. You're asserting something. You're asserting something true and then you're willing to change your mind about it at the entity too. Perceive a certainty, correct when necessary.
Juan Sequeda: All right. Before we say quick goodbye, just reminder, next week we have Muhammad Side, who's from Capco. We're going to be talking about metadata. Is that really a graph problem or not? With that, Jeff, this has been a pleasure. This has been a phenomenal conversation and I can't wait to meet you in person and go sit at the bar and just chat and just hear all your stories. You are a fascinating individual.
Jeff Jonas: ...
Juan Sequeda: Phenomenal storyteller.
Jeff Jonas: I prefer nutjob. Hey, thanks, guys.
Juan Sequeda: Cheers.
Tim Gasper: Thanks so much.
Speaker 1: This is Catalog& Cocktails. Special Thanks to data. world for supporting the show, Carly Bergoff for producing, John Loins and Brian Jacob for the show music. Thank you to the entire Catalog& Cocktails. Don't forget to subscribe, rate, and review wherever you listen to your podcast.
DESCRIPTION
Entity resolution.Is it a single source of truth? Is it multiple sources of truth? Is it multiple data sources that refer to the same real-world thing?
How do we make sense of it? How can we harness the value of data through entity resolution? Talk to Jeff Jonas from Senzing on this week’s episode of Catalog & Cocktails and you’ll understand.
Today's Host
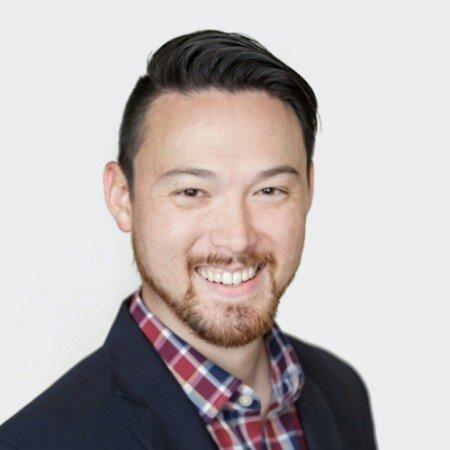
Tim Gasper
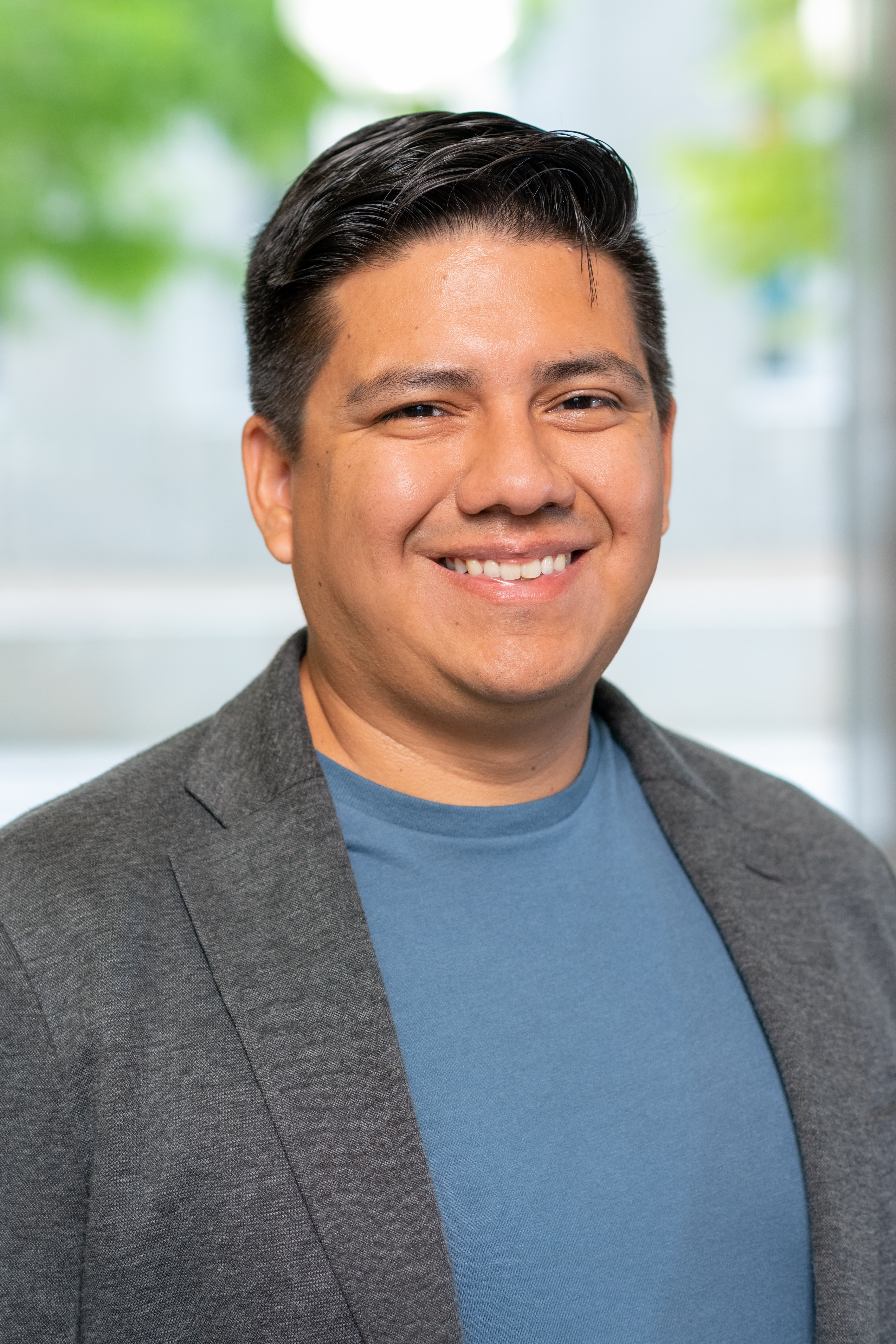
Juan Sequeda
Today's Guests
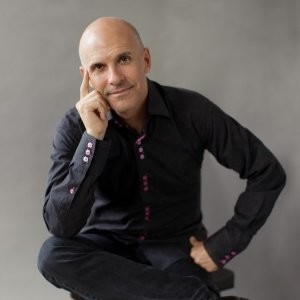