AI: No one wants your models. w/ Andrew Eye
- 0.5
- 1
- 1.25
- 1.5
- 1.75
- 2
Speaker 1: This is Catalog& Cocktails, presented by data. world.
Tim Gasper: Hello everyone. Welcome. It's time for Catalog& Cocktails. Your honest no BS, non- salesy conversation about enterprise data management. Catalog& Cocktails is presented by data. world, at the data catalog for leveraging agile data governance to give power to people and data. We're coming to you live from Austin, Texas. I'm Tim Gasper, a longtime data nerd and product guy at data. world, joined by Juan.
Juan Sequeda: Hey everybody, I'm Juan Sequeda, Principal Scientist at data. world. And as always, it's a pleasure to be able to go chat about data, and today we're going to chat about a topic that it's not the one that we chat a lot about, but it's one that we need to chat more about. Our guest is Andrew Eye, who is the CEO of ClosedLoop. Andrew, how are you doing?
Andrew Eye: Hey Juan, hey Tim. Thanks for having me. Doing great.
Juan Sequeda: Awesome. Well, I'm really excited because on our previous conversations, I just love your honest no BS takes on AI, and we're going to get into a lot of just honest no BS- ness, right now, but let's kick it off first. What are we drinking? What are we toasting for today? Andrew, kick us off.
Andrew Eye: Well, we're here with you guys in Austin, Texas. So what else would I be drinking other than a Lone Star? Yeah, we're doing it right.
Tim Gasper: Appreciate you representing the National Beer of Texas.
Juan Sequeda: Yeah, which I realize I'm not having any Texas beer, which I should, but I'm having a Firestone Walker 805, which I don't know what it is, but we'll find out in a second. How about you Tim?
Tim Gasper: I am drinking a Texas beer here with a Celis White, which is sort of a nice light beer. Cheers. Excited to have you on the show.
Juan Sequeda: Cheers for AI. All right, so let's dive into this. I think one of the things that we talked about early on, got me really excited; honest no BS, why no one wants your models, your AI models.
Andrew Eye: Yeah, it's funny Juan, you and I were chatting about this, but there's this kind of underlying idea in the field of artificial intelligence that he who has the most data wins. So you see a lot of folks in the industry like," Oh, our model is trained on 50 billion people or 60 million cat videos," or whatever it might be. And the problem with that is, sometimes that's really helpful. So for instance, if you're trying to look at MRI scans to replace radiologists with robot doctors, there's really only two kinds of MRI machines. There's like two big vendors that do almost all the MRI scans in the world. So all the images look exactly the same, effectively. So getting a big collection of all the images, allows you to learn the patterns in those images, and everybody in the world is producing the same kind of images from the same kind of machines. So in that case, it's actually really valuable to have all that data, train a model, and then reuse that model everywhere. So sometimes, he who has the most data wins, but more often, the case is that whatever predictive model is created, whatever AI machine learning model is created, presupposes certain inputs. If I don't have the exact same MRI image or if I don't have the exact same data coming into this model that the model expects, then the model has no use to me. So when you look at most applications of artificial intelligence and machine learning, what you really want to do is take advantage of all the data that you have. You want to take advantage of data coming from your website, you want to take advantage of phone call records that you have, or whatever it might be, for your application. The truth is, your footprint of the data that you have and getting all of the available signal out of all of the data that you have, I like to say everybody's a snowflake. Everyone is unique in their data footprint, and so if you want to get all of the predictive signal, all of the machine learning AI goodness, out of all the data you have, you have to build your own model. And so we always talk about whenever I see people," Oh, our models are trained on X billion people." I'm like," Great, none of those people are my people, none of those people are my customers. None of those people are my patients. None of those people are my members, and so you just learned patterns about a bunch of people, using data that I don't have, on a bunch of people that I don't see." So nobody wants your models. It means custom predictive models trained on my data, my people, my interactions, that's really what I need if I want to get all that predictive juice.
Juan Sequeda: So it seems to be that people who are going off and saying," Oh, we got the ultimate model," that's really BS. I mean you do have an ultimate model, but it's not the model I need, so I don't care.
Andrew Eye: That's right. One problem is, you're not looking at my data, so your model expects inputs A, B, and C. I'll give you an example from our world. So we work in the healthcare field, some customers have medical claims data, some customers have electronic medical records, some have social determinants of health data. If I want to build the best predictive model for a given customer, I need to be able to use all those different data sources. If I try to build a model that works for everybody, I've got to go with the lowest common denominator, only the data that everybody has. So what you end up with, is if you really want to build the most accurate, most explainable, most useful model, you've got to build custom, and you got to be able to do it cost effectively for each individual customer. But yeah," I've got the world's best algorithm and you should fire your data scientist," that's BS.
Tim Gasper: This is interesting. So what do you recommend to organizations out there as they're trying to come up with an AI strategy, and think about, how do I know if I can get a model off the shelf or use an as a service type solution, that kind of has an API and I just plug in one end and out the other end comes what I want, versus, you need to build your own and sort of the spectrum in between.
Andrew Eye: So I guess another aspect of this," I've got the perfect model for you," really important question to ask is," Exactly what is this model predicting?" So again-
Juan Sequeda: It's not the data, what is trained on, but what is it trained to go do?
Andrew Eye: Exactly. It's funny because sometimes what sounds like the same use case or the same prediction, is actually very nuanced. So I'll give you another example; we work only in the healthcare space, so I'll use an example from healthcare. Folks are really interested in predicting unplanned hospitalizations, who's going to end up so sick that they get admitted to the hospital? They show up at the hospital and they end up getting admitted. What they'll do with that prediction is try to drive a preventative program that keeps people from getting so sick that they end up admitted to the hospital. That's in many cases, called chronic care management. But the problem is, the strategies employed in those chronic care management programs are different from customer to customer. As an example, some customers say," I want to predict unplanned hospitalizations," but what they really mean is," I want to predict anybody who's going to show up at the ER," whether they get admitted or they don't. Other customers say," No, for us, if you show up at the ER and you're not admitted, that's one flavor of problem, and we want to make sure we get you connected to a primary care physician. But if you're sick enough to get admitted, that's a different kind of problem; we want to be preventative before you get there. Now, if you didn't get into the nuance of exactly what is this model predicting, you might grab something off the shelf and use it for a purpose that wasn't intended. So you end up being highly accurate at predicting the wrong thing. So the first question to ask is," What is it that this model that you're trying to sell me, predicts? And is it exactly tied to my strategy?" Because I've got to know. I'm trying to predict the future so that I can change it. I want to know what's going to happen before it happens so that I can intervene and do something different before that thing happens. So I've got to be sure that we're aligned on exactly what you're predicting and does it match what I'm trying to do to change it? Does that make sense?
Juan Sequeda: Completely, and I think this aligns the theme that we always have here Juan; the data first and a shift to this knowledge first world. So for me, the data first world is what you just said," Give me more data, give me more data, I need more data. Go solve the problem." And you're saying accurately is like," No, having more data doesn't mean that you have a better model." The knowledge first, what we call," People first, context first, relationships first." And what you're describing right now is saying," Wait, I need to understand," understand meaning. What are you trying to go do? And these nuances make the big fricking difference even. I mean even the topic of," Oh, it's admitting to a hospital," whatever, we need to get into the details of that because then at a high level, we may be on the same page, but when we get into the details, we're completely off. And this is understanding, what is the questions you're trying to understand? Who cares about this? What is the context? I think this is that knowledge first kind of mindset we need to go have. All right, I love this. We're all on the same page around these things. Now, the question is, do we have to go build everything then? I mean, you're painting a picture that basically everybody is kind of selling you BS that you have to go build it on your own, but I mean, we don't have to go build... What is the balance here?
Andrew Eye: Yeah, so there's this kind of question of build versus buy in the field of AI and machine learning. There are vendors out there that are everybody. I mean, just go to any trade show in any industry and tell me... It's like AI bingo. Walk around and see how many times you see the terms AI, machine learning, predictive analytics on everybody's booth or everybody's kind of sales slicks. So should you buy something that is a pre- trained model or should you build something that's custom? Certainly I'm advocating for pre- trained models are often trained on people that aren't yours, using data you don't have. So I tend to think, pre- trained models often not the way to go unless your data inputs look exactly like the data inputs for those models. And as I said, Radiology's a good example where I don't think anybody should be going out and trying to build their own custom cancer detection image analysis models. That's something I wouldn't advise. But when you're talking about-
Juan Sequeda: That particular use case, that is a solve problem. Would you say that there's enough pre-trained models for these things that you-
Andrew Eye: Yeah, there's people who specialize-
Juan Sequeda: inaudible buy that off the shelf.
Andrew Eye: Yeah, so there's companies that specialize. Again, the way to think about this is like," What exactly are you predicting, and is that exactly what I'm trying to predict?" The second question is," What data does your model expect, and is that exactly and only the same data that I have?" So when we're talking about I'm going to read radiology reports and I'm going to spot cancer, there are companies that just do that, and the radiology reports that are coming in look the same every time. Great place to buy off the shelf AI. And you're probably not just getting ai, you're probably getting workflow tools and a full system built around a single use case. Now, let's kind of talk about when I want to predict something like churn or when I want to predict something that uses multimodal data. So I don't just have one type of data; I've got medical claims, EMR, admission, discharge, and transfer fees. These are all things specific to healthcare, but you get the idea. If I've got six or seven different types of data, and I look to those people who are trying to sell me pre- trained models and I say," Can you use this data and this data and this data that I have? And they say, No, my model doesn't take that kind of data, might be a good time to try and build custom. Now, if I'm going to build custom, what's my starting point? Do I start with hiring a PhD Data Scientist, and hoping that he doesn't quit or she doesn't quit, and go to Facebook? That's tough, but maybe I want to hire some people and then what tools do I give them? Do I just give them PyTorch and some Jupyter Notebooks and a bunch of open source stuff and say," Have at it?" Turns out the problem's a lot bigger than a handful of people with PhDs can build. And so sometimes I use this analogy, whenever there's a vacuum in the field of technology, whenever there aren't good commercial tools available, we hire technologists, programmers, to build stuff for us. So think back to the beginning of the web. When the web started, every company hired a bunch of HTML programmers and JavaScript programmers, and we all built our websites from scratch, and they were paying to maintain, and we built our own internal apps. Then fast forward a few years later, and this company called Automattic comes out with this product called WordPress, and it turns out that maybe I shouldn't be like hacking HTML and JavaScript all the time, because that's a solved problem and I can buy a content management system, and now I'm just updating text and pictures. So before there's WordPress, you have to build it yourself, but once there's WordPress, you'd be a fool to try and compete with the R& D budget of a company that has a full commercial tool, in the same way. Now today in artificial intelligence machine learning, if you're going to build, you want to be thinking about what are the tools that I should use? And we here closely, we firmly believe that Vertical AI is really the key to this, because you've got to understand the data and the problems of the particular vertical. We're in healthcare. If you want to build predictive models in oil and gas, or FinTech, I am not the solution for you, but if you want to build predictive models in healthcare, we've got not only that end- to- end machine learning platform that helps you build, deploy predictive models, but we come to the table already knowing the problems you're interested in, which means we have model templates, we already know how to handle your data. We're firm believers; we built our company on this idea of applying Vertical AI, where you've got this combination of domain expertise, data expertise in a particular vertical, combined with tools. So for us, it's a little bit of both. Should you build or should you buy? For us, we say," Look, you can build with us or you can build on top of us." If you've got internal data scientists, we'll help them go faster. If you don't, then we can provide not only a platform, but the expert, the data science expertise to help you run it.
Tim Gasper: I think Juan and I, we've talked quite a bit in general, not on the show, but more broadly about what you're saying, the value of verticalized AI and understanding the data sources, understanding the use cases, understanding the industry, and that can really influence your approach to AI, and also the tooling that you use. One of the things that I have a little trouble reconciling, and it might just be because I'm not super steeped in the AI market as I used to be a few years ago, is that there is quite a bit of horizontal tooling and infrastructure around AI. You've got like AWS and Google and everybody's got their SageMaker and things like that. You've got the data science platforms, the data COOs and the DataRobots and things like that. How do you think about or reconcile more of these horizontal data science and AI- oriented solutions versus more verticalized solutions? What's the right tool for the job when it comes to this kind of stuff?
Juan Sequeda: Actually help us navigate that tool space. I mean, when you go through the expo halls of these places, you're going to see all these types of tools. Without having to name vendors, what are the different categories that you're seeing around that? So our listeners, you can help navigate them.
Andrew Eye: So I'll speak to our industry, but I think it's probably representative of other industries where there are vertical solutions, there are horizontal solutions. So the way we think about this is, in the healthcare space, there's two types of vendors that are in this AI and machine learning space. There are horizontal vendors who sell pre- trained models, and basically their value prop is," You don't need data scientists internal to your company, you should fire them all because I own all the intellectual property and I've got all the world's best models." The challenge that they have is," How do I adapt those models to each customer's unique data? And," Oh, what if I don't have the model that that customer wants? Or what if the end point that I predicted is actually slightly different than the thing that they wanted?" And so you get this maintenance problem. More importantly, if you're trying to sell that solution and there's even one data scientist there, you're effectively saying," You should fire that person and hire my firm instead." Turns out that's a really bad sales motion. So vertical focus, what we see in the healthcare space is, most of those folks are trying to sell you the world's best algorithm. We don't sell algorithms, we sell a machine that helps you build algorithms. So there's those vendors that are vertically oriented. Then you've got the horizontal folks, the DataRobots, the Dataikus, et cetera. Those are big companies that are going to do very well in filling in the gaps where there isn't a vertical solution. I'll give you another tangible example. In the healthcare space, why didn't Oracle, or Salesforce, or NetSuite, or some other horizontally oriented database driven application, why didn't they win the electronic medical records business? Now, Oracle's bought one of the biggest vendors in the EMR space in Cerner, but why didn't Oracle win against Cerner in the beginning? Healthcare has a particular record of buying healthcare specific solutions, because it's highly regulated, because there's so much domain expertise required, and there are other industries like that. So I think when we think about us versus the horizontally oriented vendors, I'm going to lose in an oil and gas pitch to DataRobot every time, and I'm going to win every time in a healthcare pitch against DataRobot. Because the horizontal vendors show up and say," What's ICD 10?" Which is the coding standard for all medical... When you don't have that level of domain knowledge, you can't even get started, and you end up paying to educate your vendor. You've got to teach them your language before you can even get started. And so we think, particularly in verticals that are large enough, vertical AI wins. There's a great article on this from way back in 2017 maybe. There's an article from Jerry Chen at Greylock Ventures called The New Moats. And I always point people to this, and Jerry basically talked about AI back in 2017, as this new emerging category of software. He calls it a system of intelligence. He specifically calls out vertical AI where there's deep expertise in the data and problems, the basically domain expertise of a given vertical. That was his prophecy as to kind of what was going to win, and we've been kind of pushing that article for five years now.
Juan Sequeda: Wait, but even in the vertical space, there's two parts. There's the vertical that is selling, the perfect AI model does everything, and that's the one that you're calling BS on. But there's also the vertical that says," We give you the tools, the engine, the machine, so that you can go train your model, giving your data, but with all the vertical expertise," that's the one that is the valuable, if I understood correctly.
Andrew Eye: Well, that's our position inaudible that's our approach. So clearly we're biased, but yes, our approach is rather than telling you," I'm going to give you a specific pre- trained model," I'm not going to sell you the idea that I've got an algorithm that's perfect for you, rather, I'm going to build a custom predictive model for you. But what differentiates us is, I then hand you the keys whenever you're ready. And I say," Listen, if you want to iterate on this model, you want to build the next model yourself, I'm going to give you the building blocks and the tools, to build those models yourself." For folks who are in the AI space, you'll be familiar with this idea of features. So features are just... You go from raw data to these derivative variables that you believe are going to be predictive of a given outcome. 80% of the time data scientists spend is on building features. It's going from raw data to these variables they think will be predictive. That ends up being a lot of labor. It also ends up that everybody's reinventing the same wheel within a given industry. I'll give you a tangible example from the healthcare space. If you ever look at medical claims data, which is my favorite thing to do, but I'm guessing you guys don't do all the time, you'll see things like this. You'll see Andrew got admitted to the hospital yesterday, then he was admitted on Monday, he was discharged on Tuesday, he got admitted again later on Tuesday, then he was discharged again three days later, and got admitted again. And you're thinking," Gosh, how is Andrew getting admitted to the hospital, discharged, and showing right back up on the same day? Is this the klutziest guy in the world?" And the answer is, that's actually all one admission. It's not a discharge, it's actually a transfer, but it shows up in the data looking like a discharge. Now if you're a brilliant data scientist, but you don't come from a healthcare background, you're not going to know that.
Juan Sequeda: That's the knowledge right there.
Andrew Eye: That's exactly it. And not only are you not going to know it, you're going to spend about a week writing the sequel code to merge those things. Now, if you've got a great vertically oriented partner, and I don't mean this to be a sales pitch, but this is where we add value, is not only are we giving you... Now there's a field called enterprise feature stores where you can define variables, collaborate on variables. This is becoming a part of the AI infrastructure. We provide an enterprise feature store to our customers, but we fill it up with a bunch of pre- computed features. So when I tell you," Here's the number of prior admissions," I've already built in the logic about this problem of discharge and readmission, which is actually all one admission. And that is the productization of all that domain expertise.
Juan Sequeda: Now, this is exactly the point of knowledge, where you have that expertise. And then you have the common sense knowledge, but knowledge is very specific to the industry. So you're making this great point here how we need to have the vertical aligned with the knowledge here.
Andrew Eye: Yeah, I mean here's another example for you on just because this is another favorite one. So there's a concept in AI and machine learning called feature drift, which is," Hey, I trained a model six months ago, and it had such and such ROC AUC, as point 0. 87 ROC AUC; it's super accurate." Then all of a sudden I start to notice that my model is less accurate than it used to be, and what ends up happening is that the data that you had originally, and you trained your model on, you start to see new patterns in the data that you're receiving, that make it different from what you saw in the patterns previously. Here's a great example from healthcare. In healthcare, you have drug codes, and you might build a feature that says," Is Andrew taking a Statin?" And so you'll have scores for all of your patients saying," Are they on a Statin? Yes or no?" And you'll use different drug codes to figure out whether or not they're on Statins. The problem is the definition of Statins changes. New drugs are released to the market all the time. When a new statin hits the market, there's a new drug code. Your data that you trained on, didn't have that drug code because it never existed. How are you going to handle the maintenance of recognizing when those new drugs hit the market, and how are you going to know when to retrain your model such that you're taking advantage of all those new drugs that are being prescribed correctly? And again, you're not worried about drug codes if you're in FinTech, but if you're in healthcare, this is a problem everybody's got. Again, it's just another illustration of why vertical AI is so important.
Tim Gasper: No, this is fascinating. One extension of this line of thinking around vertical AI that I have for you, I'm curious is, healthcare, obviously, both before this and through this conversation, it feels like it is a very relevant place for vertical AI. Where are some places, other industries, other use cases where you see sort of vertical AI being really impactful versus more interestingly perhaps? Are there certain verticals or use cases where actually vertical AI doesn't quite make sense, like it doesn't have a good fit for some reason?
Andrew Eye: Yeah, I wonder about this. Obviously, we spend more time thinking about our swim lane, but I'll tell you some other examples that we've seen. I think retail is another area where vertical AI makes a ton of sense. So if you think about the types of data, you're going to see purchase data, you're going to see website traffic, you're going to see marketing data. And to the extent that that data looks pretty much the same, that might be a good application of vertical AI. The challenge is, in healthcare, you've got this underlying kind of common standard. People think healthcare data is messy, but the truth is, there are coding standards; ICD 10, drug codes, lab codes that standardize the data somewhat in our industry, make it really particularly useful for vertical AI. Some areas where I think vertical AI doesn't apply, the counterexample I always use is hedge funds. If I'm a hedge fund and I'm trying to predict things, I'm going to do some crazy stuff. I mean, there's classic examples of this, where hedge funds we're using aerial photography of parking lots and how crowded the parking lots were, to predict retail sales for Walmart for Christmas. I don't think that's a standardized data stream that every FinTech company's going to be thinking about. So the more you're in that long tail of creativity and your problem is unique to you, that's where those horizontal tools make a ton of sense.
Juan Sequeda: You said something super interesting, which is like if you're doing this crazy things and being very creative, that is where the vertical AI doesn't come in. This is actually a bit ironic, or maybe not. I mean you're saying," Hey, I want AI to be really smart and tell me things, but hey, if you're area you need to be very creative, then that AI is not going to go work." Like that vertical, very specific AI. So it's kind of interesting to see-
Andrew Eye: I think the point is, and I want to be clear here, what I'm not saying is that our customers that are building on top of our platform, and a vertical specific solution aren't creative. The point is, are you trying to reinvent the wheel or not? If every other company like you, has got to figure out how to handle drug codes and updates to drug codes all the time, maybe don't spend your time building that yourself, if you can buy it off the shelf from a partner. So I'm spreading the cost of solving that problem over hundreds of customers, where you're trying to compete with my R& D budget with only your one internal customer. So what I would say is, it's not that it's not about creative or not, it's about, is the problem you're trying to solve, reinventing the wheel of everybody else in your industry? If it is, then look around and see if there isn't some vertical solution that maybe can help you get there faster, and focus your creativity on the next level problem; building with those kind of building blocks to solve more problems. Here's another analogy for you. Every company in the world needs a CRM, right? Anybody who sells anything needs Salesforce or something like it. Should I go build a CRM myself?
Juan Sequeda: No. Well, you do technically start with the spreadsheet if you're really small.
Andrew Eye: Yeah, right, if you're small, but the point is, I shouldn't go compete with Salesforce. My sales process is nuanced, it's different, it's not the same as everybody else. I'm going to need to grab Salesforce, but I should focus my energy on customizing Salesforce to fit my business process. I'm going to need a Rev Ops person internally. I'm going to need somebody who knows how to configure and run Salesforce, but that doesn't mean I should try to go build Salesforce myself. Same thing here. If you've got internal resources, get them these higher level tools so that they don't have to focus on the minutia of calculating admissions correctly. They can start with that problem, solve, and implement faster. It's all about ROI.
Tim Gasper: No, that makes a ton of sense. Juan, as you've been talking through a few of these examples, one other thing that strikes me and I kind of look in your direction, Juan, because I'm curious if you had this observation as you went through these examples as well, that in these examples there's a little bit of a semantic contract that exists. Like, when you're looking at retail, there's this concept of a click and a customer and a purchase. In healthcare, you've got these codes. When you're looking at parking lots and cars, there's no kind of semantic contract in general, let alone in that industry, sort of around a ratio of parking lot density or something. So the more semantics are very unusual or not well understood, or not well sort of communicated across the industry, probably makes it harder for verticalized AI to kind of inaudible.
Juan Sequeda: Even between those two levels that you said like, oh, in retail we have these standards of expecting there's a click and all that stuff, that's like the first part you need to go have. Have an agreement within the industry that," Oh, we talk about these concepts," but then there's, take it to the next level, is like," Is there an agreement, kind of very specific, which you have in healthcare with codes?" We don't have that in... I mean, healthcare is great that it has that. I think other industries try to go standardize these things-
Tim Gasper: I see where you're going.
Juan Sequeda: They try to go standardize these models, inaudible, these models, but healthcare is the one that's really taking it to the next level, because guess why? Regulatory purposes-
Tim Gasper: So there's a stronger contract in healthcare, whereas actually marketing and retail might actually be a weaker contract. What is a click? We actually might disagree on what that means.
Juan Sequeda: Oh yeah, what is a real life-
Andrew Eye: I mean part of this just becomes philosophy, but I think this is really tangible. If I'm sitting there trying to figure out what type of tools should I use and should I build or buy? And if I'm going to build, what tools should I start with? It's this simple. We already talked about how to decide if you build or buy; if you buy pre- trained models or not. But let's say you're going to build, how do you decide what tools to start with? I would say look for vertical tools first. If you're in any industry and any vertical AI vendor exists, that's the place to start because they're going to know more about your problems, your data, et cetera. But the other question you asked me was, where will horizontal AI win? And the answer is, wherever there isn't vertical AI, and there's lots of places where there isn't vertical AI because the market isn't big enough. Maybe somebody wants to apply AI to selling deer feeders. But I'm guessing that that market isn't big enough to warrant a vertical AI specific player, who only focuses on deer feeders. So that long tail of all these other problems... Look, I think DataRobot, Dataiku, these are awesome, huge, going to be big companies. They're just not going to win in healthcare. So I think the answer is, if you're trying to make this decision on who to look at, look for vertical vendors, if they exist. You should build on top of those. If I have one customer who decides that parking lot data is really important for their problem, they can add that to what we already give them, but they don't have to reinvent everything we built.
Juan Sequeda: This is very tangible kind of a advice and I really appreciate that. I want to sub clarification here is that, go look at the vertical, but be careful and be honest, and no BS about the weight. It's not everything. Don't take it all for a granted and go drink that Koolaid. It could be very critical about that, and understand those nuances. So I think that's a really important one. Now, another thing we wanted to go talk about, because time flies here. This is an awesome conversation, is we talked about this before, was, there's all these really important problems in healthcare we're trying to go deal with, and there's all these really smart people working on it, but a lot of the AI research and application today, is not in this area of healthcare stuff that it can really impact and change the world. I mean, honest no BS here, is yeah, kind of fricking annoying that we have all these great minds doing all this AI stuff, so we can go click something on fricking Instagram or whatever. What's your take on this? I mean, that's a frustration I have. What do you feel?
Andrew Eye: Yeah, Juan, you and I have talked about this before and it is a personal point of passion for me. It's so clear AI is the transformative technology of our time. When I back up five years ago and we were starting ClosedLoop, I always look at... Whenever I'm starting a company, I'm looking for what are the major technology trends that are going on, and what's that wave that I can ride? Five years ago, there were three trends that looked promising; cryptocurrency and kind of blockchain. You had augmented reality and computer vision, and you had artificial intelligence and machine learning. Of those three, you tell me which one is impacting most people's daily lives, now, five years later. AI has been that breakout technology of the last five years and even longer, but the number one place that you see it is in ad targeting. The number one place you see it is, the ads are so good on your Amazon Alexa sitting in your kitchen, or on your phone when you're scrolling through Facebook, or wherever you are, the ads are so good that you believe Facebook must be spying on you. They must be listening to my phone because I just said something five minutes ago and here's the ad. That's how good the technology is, that we believe that they must be cheating and lying and be listening into us all the time, because the ads are so good. So that's how powerful this technology is. But yeah, I'm pissed, like no BS, right? I'm mad and we all should be. That's all that our generation decided to use this technology for, was to give us better targeted ads? What's the opportunity? How about-
Tim Gasper: Our doctor should be as good as Facebook is, right?
Andrew Eye: How about that, right? Yeah. This is why people get excited to come work here, and to work in the healthcare industry as a whole. My time in the healthcare industry has been amazing because people care. If you want to be mission driven, this is the industry to be in. I mean, just look at the pandemic and what people went through to try and help one another. So you've got this amazing industry where people are really trying to, and what are they trying to help? They're trying to help reduce human suffering. They're trying to help figure out... In the Facebook world, in the marketing world, there's only one question that Facebook is interested in. What will you click on? The whole social network is just a way to collect information to target ads, and the only question Facebook really cares about, and I'm not picking on just Facebook, but the advertising industry only cares about one question; it's attention, it's clicks. So that's their predictive model. What will you click on? In healthcare, there are two fundamental questions; what's wrong with me? When you go to your doctor, you only want to know two things, what's wrong with me and how can you make me better? That's diagnosis and treatment. Those are the two underlying questions in healthcare. Which would you rather work on, clicks or saving lives and preventing suffering with artificial intelligence? So I think we can do better as a generation.
Juan Sequeda: This is a very powerful statement you just said. Do you want to work on what will you click on, or do you want to work on what's wrong with me, and how can you make you feel better? That's an extremely powerful statement. I hope everybody listening here is actually kind of digging a little bit inside their soul and figuring it out. Ask yourself that question.
Andrew Eye: It's addictive, man. Once you get into this field, my background wasn't always in healthcare, but I had a personal experience with a child who went through some challenges. When you go through that diagnostic odyssey as a parent, and you realize nobody's using my data here. Oh my gosh, this technology is possible and nobody's using it. But the good news is, you tell me how good this data is, how good these technologies are. Fast forward into the future, whether it's five years from now, 10 years from now, pick your time horizon. Will you go to a doctor who doesn't use this technology to better diagnose and treat you? I think we're all going to choose our doctors, not just our doctors. We're going to choose our hospitals. Because this technology, it's not a question of if it's only a question of when. Facebook level intelligence is coming to healthcare. The only question is when. And I think that consumers are going to drive that because they're going to demand that they go to places where it's not AI versus their doctor. It's their AI super- powered doctor, who helps make better decisions, better recommendations, based on this field of technology.
Juan Sequeda: Before we start wrapping up and go to our lightning round, how do we get people to start thinking more about this? I mean, this is a very powerful message. I mean, is this more education we have to do, kind of grassroots from schools or I mean campaigning? I don't know. What are your thoughts? How do we get people more into the healthcare field or let's say outside of the click field to apply it to somewhere else, where they can really impact lives?
Andrew Eye: I'm so encouraged because there's a whole generation of people who are coming out of college, even high school, just so much more socially aware and with a genuine passion for making a difference. I think that's the good news. That spirit of, I want to do something that matters, is already there. I think we need more folks going into STEM programs. There's a big topic of bias and fairness around AI. One of the things we know is if you want to have unbiased, fair predictions, you need a diversity of talent. So here at ClosedLoop, diversity, equity, inclusion, belonging, these are not topics that are just nice to have, these are existential threats. If I don't build a diverse team, I'm going to fall into the trap of people that have come before us and build models that are inherently biased. So to your question, what do we need more of? We need more people taking an interest in this. We need more education for people coming from diverse backgrounds, to build up the talent pool with a more diverse talent pool.
Tim Gasper: Great answer.
Juan Sequeda: No, this is very powerful. I love that-
Tim Gasper: A lot of work to do.
Juan Sequeda: I love how we ended up ended with this really powerful message. But time flies. I love how we've gone through so much stuff, but it's time to go for our lightning round, which is taped, presented by data. world, the data catalog for your successful cloud migration. And I'm going to kick it off first. So first question, we didn't talk about this. This is a topic very passionate to me, is graph technology making a big impact in AI and vertical AI?
Andrew Eye: Yeah. Super interesting. We get this question from folks who are a little further along. I think the answer's going to be yes. I think right now, is that the first thing you need to do, if you're in healthcare in our vertical? Maybe not. If you're in marketing, I bet this is way more important because the interrelationships, and who am I looking to target? I could see it being way more applicable. But I think graph technology we're super bullish on in general.
Tim Gasper: That makes sense. Second question, as vertical AI continues to grow and gain traction, are we going to see the number of ML and AI engineers in the industry, actually start to shrink?
Andrew Eye: I think the answer is no. I think the answer is how many of them should you need? So go back to the Salesforce analogy. There's no fewer people building database driven applications, workflow tools than there were before. But you don't need to employ as many of them in your company, because you're not trying to reinvent Salesforce. So I think the number of ML engineers is likely to continue to grow. How many you need in your organization should not go up at the same rate. You should have fewer people who are able to leverage higher level tools. Again, like the website analogy, I don't need an army of 50 web engineers if my starting point is WordPress.
Juan Sequeda: All right, next lightning round question. How much of this problem is, I need AI versus I actually have a data integration problem?
Andrew Eye: It's interesting because sometimes we get this from customers like," Oh gosh, my data's so messy, I need to get everything cleaned up, and I can't even think about ML or AI until I do X." My response is always," Well, what's in your data warehouse already?" One of the great things about artificial intelligence and machine learning is it can get over the problems of messy data. So as an example from our field, if I have someone who's not coded as diabetic, but I see a prescription for insulin, ML and AI, figure out that that person probably actually has diabetes. They look a lot like the person who's coded as diabetic. And so even if your data's messy, you might be ready for AI and machine learning depending on the problem. And I always say," Don't let perfection be the enemy of the good." Can you actually get benefit out of machine learning and artificial intelligence right now, in spite of the fact that your data's incomplete or messy? The answer isn't always yes, but you shouldn't assume that you've got to have perfect data. This argument of garbage in garbage out, is overstated. The idea that you can't start until your data is cleaned up and perfect. Your data will never be cleaned up and perfect. It's probably good enough to start with, the example I just gave, but you should be thinking about that. If you don't have an AI or ML strategy now, you are behind. This is a board level conversation; CEOs, CXOs are asking," What's our AI strategy?" Because they learned this during the internet boom. People who are late to the internet, they don't want to be late to AI.
Tim Gasper: That's interesting. I feel like there's a whole topic that I wish we had time to explore, we don't right now around... because everybody inaudible the diagram and at the end of the maturity is like" AI, we're going to achieve a critical." And it's like," Wait a second, maybe AI is part of the journey."
Juan Sequeda: Like you said, the garbage and garbage out is a bit over inflated. So that's interesting. This is a good topic for-
Tim Gasper: Last question. Fast forward 10 years from now, you actually kind of alluded to this earlier in the show, right? 10 years from now, will AI actually be the primary evaluator when I go to the doctor, or are we still too far away from that?
Andrew Eye: I don't think for general diagnostics, that you're going to walk in and... There is no replacing your doctor, your physician for diagnosis. We should be able to supercharge your doctor with tools that say," Did you think about this?" So the physical interaction, like hearing your demeanor, hearing your breathing, those things will never be replaced. Just being able to have a conversation and a relationship with you, so you'll tell me the information that I need to make a proper decision. That never goes away. What AI's good at is, I've looked at 10 years worth of medical records from before I ever talked to you. And by the way, hopefully we get to the point where I'm not just looking at your medical records, I'm looking at your family's medical history. A physician doesn't have time in 15 minutes, to digest all of that information. And so hopefully what we do is AI's job is to look at all that information and surface a couple of ideas that prompt the physician to have a more informed conversation for you. That's where I think we get in 10 years.
Juan Sequeda: This reminds me of a friend of mine call out for... His name is Bart van Leeuwen, on Twitter, he's called Semanticfire and he's a fireman in Amsterdam. And we was talking about this one day with him. He's like," I'm a fireman, I'm a professional. There's a building in fire. We'll sit in front of it. I'll figure out what... Have my strategies. I don't want an AI tell me what to go do. What I do want is that AI on the shoulder, that's just tapping me saying, FYI this, FYI that," and I'm like," Thank you. Got it." That's input, and now make my decision because I'm the professional here who's going to go in into that building that's on fire. I'm the one who's going to get that surgery.
Andrew Eye: 100%. Assisted intelligence, augmented intelligence. Let's swap out the A for whatever you want.
Juan Sequeda: This has been a so awesome conversation. We've gone so many parts. Tim, T- T- T, Tim Takes us away with Takeaways. You go first.
Tim Gasper: Oh my gosh. So the title of this whole thing was No one Wants Your Model. And we started off with that as the no BS question, and you really helped bring light to why some of the... There's an overstated perspective on sort of like, oh, a pre- trained model that you're just going to plug and run with for a large number of use cases. There's this idea that those who have the most data win." I ran my model on 50 million cat videos and therefore it's the best damn cat video model ever." Well, if your data's super uniform and you know can train your own or you can use your model against exactly the same data, then maybe actually that does work well. And there's certain use cases where that can make sense, in vertical situations or non vertical situations. However, most of the time, the data isn't consistent and the model is trained on data that's different than your own data, and the knowledge or the use cases are different. And we really need to make sure that we all line it up, and you may need to build your own model. So everyone you said is kind of a snowflake and you really need to have the right model for the job. And that vertical AI solutions, particularly ones that are not just leveraging sort of pre- train models, but are actually helping you develop models, can really make a big difference. Exactly what is this model predicting? What is it trained to do? All that nuance. It's really important to know about that model so you can make a good decision on whether or not, something off the shelf, a pre- train model's going to help you or should you really be developing your own, which in many use cases, is actually the better approach. You mentioned do you have to build or buy AI? You kind of said sometimes it feels like we're playing AI bingo. When you're at a trade show, you look around at all the different trade show booths and things like that. It can be confusing, difficult to navigate. Well, pre-trained models are not always the way to go. Pre-trained models were built by people that aren't interested in data that you don't have. So be smart about the use cases. And in many cases, building your own model can make a lot of sense, especially in the industry of healthcare. What about you, Juan?
Juan Sequeda: So we're talking about tool landscapes. I love how we do the vertical and the horizontal. So the vertical ones, the people are pitching you," You don't need a data scientist. I got all the best models." It's like, yeah, be careful with that stuff, because you're going to have a lot of the issues, like," Can you put your own data into that? How do you maintain that stuff?" But you really need to think about it from the vertical perspective is, not just am I selling you the model, but we really want to be able to have that machine that where you can go train your own data with that. But you also have the horizontal side, like the DataRobots and Dataikus, of the world where they're going to help you fill in the gaps. So I think there's going to be this balance. You said to yourself, start with the vertical approach if there is one, but then also got to be very careful and understand what they can and can't do. So we went into the vertical AI and question is, where does that make sense? My takeaway is if people are reinventing the wheel over and over again, that's where vertical AI will come in. In places like healthcare, you've always had these standards, which is very helpful. In retail, it seems like it's an area that could be very helpful too, but they lack a lot of these standards. So that's something to be careful of. Other areas like hedge funds may do crazy things; maybe a vertical AI right there won't work. Finally, we wrapped up with the, where are we today? AI today is mostly used for ads, and this very existential question," What do you want to work on?"" I will work on how do I find more clicks or work on how am I going to find out what's wrong with me and what can make me feel better?" I think that was a beautiful way to go close this. And then this next generation is really hungry about making an impact, changing the world, and we need to have diverse people to be focused on these problems about fairness of data, and otherwise, is an existential threat. How did we do on our takeaways? Anything else we missed?
Andrew Eye: Man, that was amazing.
Juan Sequeda: That was you.
Andrew Eye: I didn't realize how diligent of note takers you guys are or else just like you had these incredible memories. That was phenomenal. I know you guys have a lot of data junkies in the audience and folks who are interested in these types of technologies, so we'd love to hear from them. And if this is stuff that inspires you as well, we're growing quickly, so we'd love to hear from you.
Juan Sequeda: Thank you. To wrap up, three questions back to you. What's your advice about data, our life? So who should we invite? And third, what are the resources that you follow? People, podcasts, conferences, whatever.
Andrew Eye: Yeah. Oh gosh. First question was what's my advice?
Juan Sequeda: What's your advice?
Andrew Eye: Like I said, I think what we're hearing from customers is that AI has become a board level conversation, and so if your boss isn't asking about this yet, they're going to be soon. My advice is don't miss the second internet, and so start thinking about how should this be applied within your own organizations? Who should you invite next? Well gosh, we're biased. We love the healthcare field, and again, I'd love to see you guys kind of focusing on stuff that has a real big impact and meaning. Eric Topol is an amazing speaker, and wrote the book, many books on the topic. So I think Eric's an amazing potential guest for you guys. What resources? Oh gosh, there's This Week In AI, This Week In Machine Learning. TwiML is a great resource. Then all of Eric Topol stuff is just amazing. All of his books on healthcare AI specifically.
Juan Sequeda: Andrew, thank you so much for this fascinating discussion. A truly honest, no BS discussion and very inspirational. Are you out there working on AI? Are you helping to get more clicks? Are you helping to go save lives? Think about it. Cheers.
Andrew Eye: Thanks guys. Cheers.
Tim Gasper: Cheers.
Speaker 1: This is Catalog& Cocktails. A special thanks to data. world for supporting the show, Karli Burghoff, for producing, Jon Loyens and Brian Jacob for the show music, and thank you to entire Catalog& Cocktails fan page. Don't forget to subscribe, rate, and review wherever you listen to your podcasts.
DESCRIPTION
You can’t buy a predictive model off the shelf. And if you COULD… would you? It’s the old “build versus buy” debate and with data in a constant state of change, building and deploying AI is more challenging than ever.
Join Tim, Juan, and Andrew Eye, CEO at ClosedLoop.ai, to discuss the challenges of AI deployment, AIOps, and maintaining models as data changes.
Today's Host
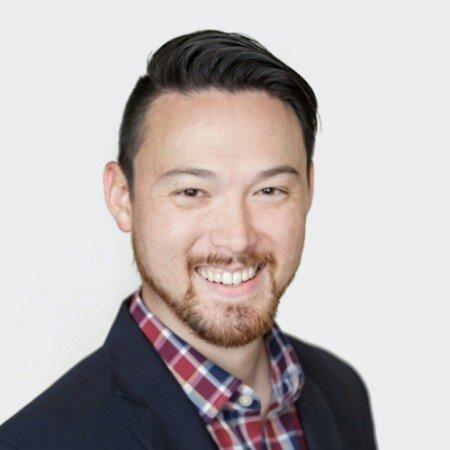
Tim Gasper
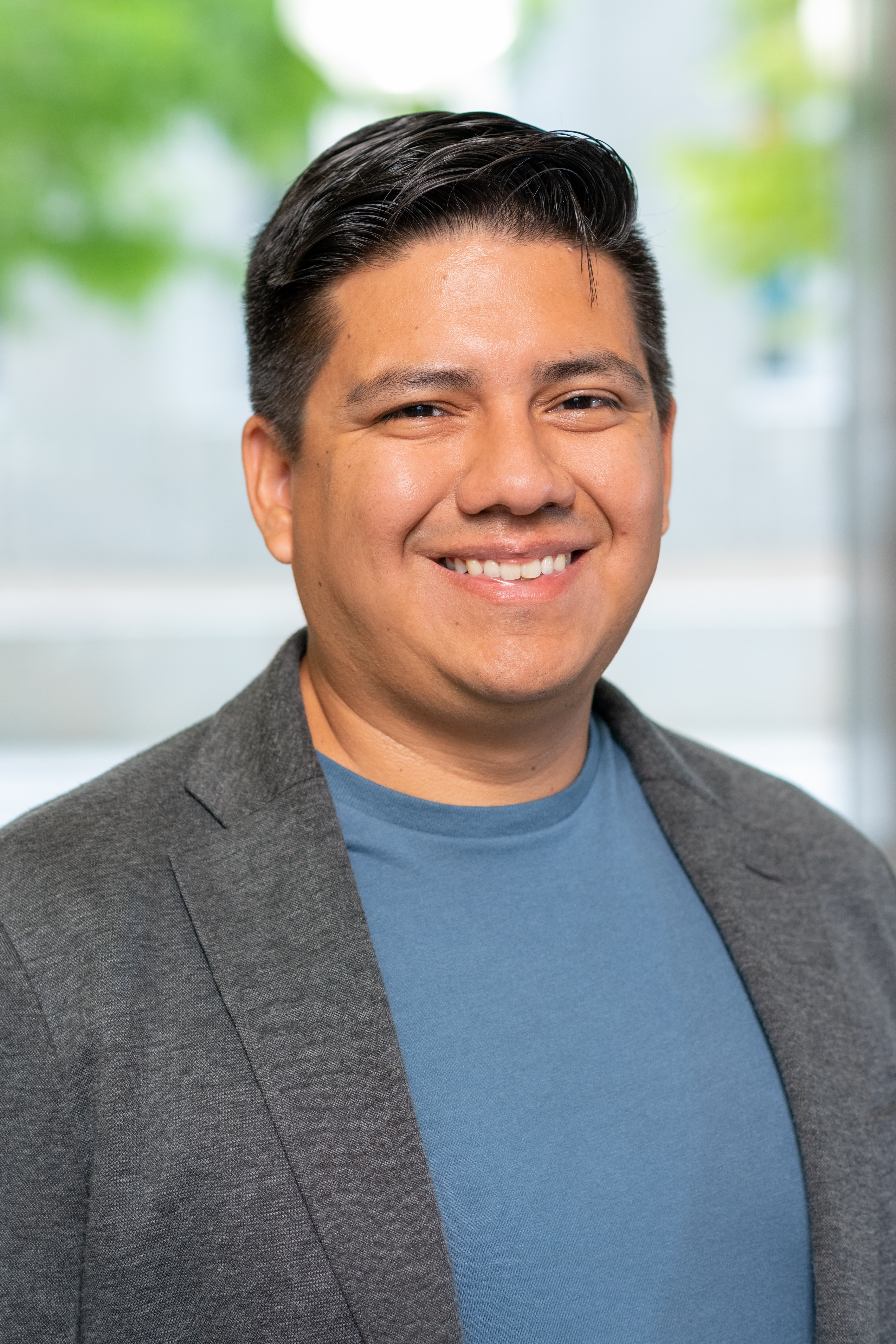
Juan Sequeda
Today's Guests
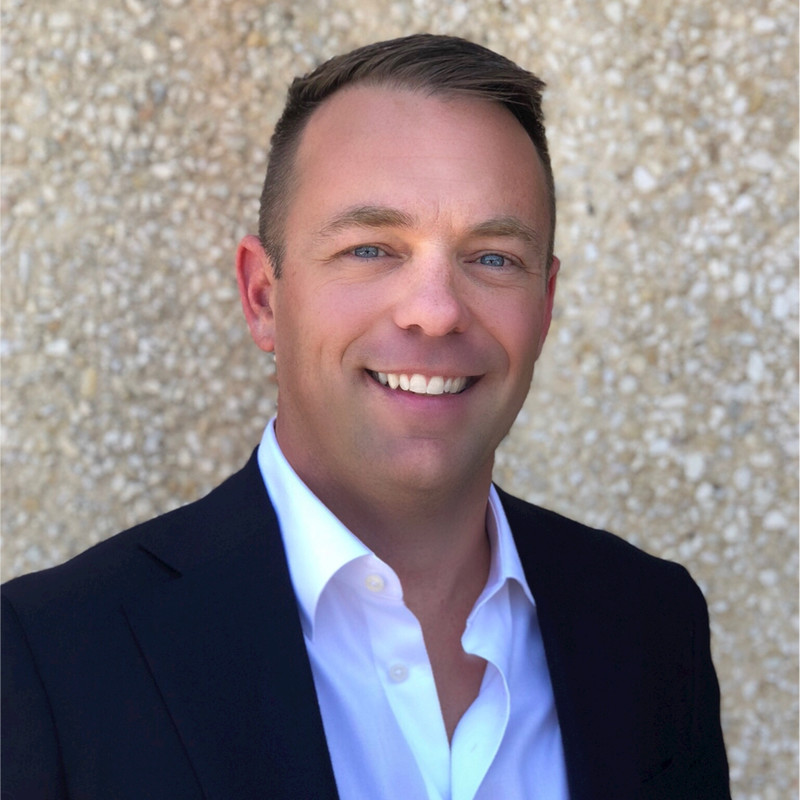