Data Modeling: your data isn't going to model itself w/ Anna Abramova
- 0.5
- 1
- 1.25
- 1.5
- 1.75
- 2
Speaker 1: This is Catalog& Cocktails, presented by data.world.
Tim Gaspar: Hello, everyone. Welcome to Catalog & Cocktails, presented by data. world. We're coming to you live from Austin, Texas. It's an honest, no BS, non- salesy conversation about enterprise data management with tasty beverages in hand. I'm Tim Gasper, longtime data nerd, customer guy, product guy, joined by Juan.
Juan Sequeda: Hey, everybody. I'm Juan Sequeda. I'm the scientist guy here at data. world. And as always, it's a pleasure, middle of the week, end of the day, to go take a break, have a drink, chat about data. And today, we're going to have an awesome conversation because it's one of my favorite topics, very deep in just my upbringing of data, which is modeling and about knowledge. And today, I am really happy that we have Anna Abramova from SqlDBM. You must be following her on LinkedIn because she's really pushing all data modeling on everybody's radar. So, Anna, how are you doing? I think you're on mute, Anna.
Anna Abramova: Sorry about that. I'm doing phenomenal, Juan. How are you?
Juan Sequeda: We're doing great. And we're really glad to have you here. We've been seeing all the SqlDBM and data modeling, so it's just great to have this conversation.
Tim Gaspar: You're always chiming in on great topics, starting great conversations. And now, we get to hang out and have a cocktail together.
Juan Sequeda: I love to say that I'm surprised that you're not on some fancy boat or something, so.
Anna Abramova: I should have. Sometimes, only on weekends.
Juan Sequeda: All right. Well, let's start off. So, what are we drinking? What are we toasting for? You go first.
Anna Abramova: Yeah. I know you guys already have your drinks. So, I have a question. Maybe I went overboard. You tell me. I have a question. Have you ever seen a vending machine with champagne?
Tim Gaspar: A vending machine with champagne? I don't think so.
Anna Abramova: Right? Me neither.
Juan Sequeda: No.
Anna Abramova: But I saw one. I know vending machines are so normal and common, but I saw a vending machine with champagne. It was a couple of weeks ago already. It was during Christmas break or something like that. And so, I went to the vending machine, and I got the champagne bottle. And then I didn't have a reason or an occasion because it's like a tiny... So, this is the one. It's very tiny, mini champagne bottle out of a vending machine. And I hadn't have a reason. It's too small for a party or a present. So, I thought, " Okay, today's the occasion."
Juan Sequeda: That's a unique story. Where was this? Where was this?
Anna Abramova: This was in San Diego, in one of the very fancy brunch spots in Little Italy. We have this really nice, little neighborhood. And yeah, they give you a token, like this metal, piece of metal. It's not cheap. Don't get me wrong, but then you buy the coin. You go to the machine. Put the coin into the machine, and then it gives you champagne, like this tiny, little bottle. So, that's what I'm drinking. I'm going to add a splash of... I'm going to just open it right now.
Tim Gaspar: Yeah, open it.
Anna Abramova: I'm opening it and adding a splash of... Hopefully, I don't get myself wet.
Tim Gaspar: Watch the.
Anna Abramova: Living on the edge. You're living on the risky side. All right, looks like we're good. Yeah, I'm just making myself a mimosa, a good, old mimosa.
Tim Gaspar: That's perfect.
Juan Sequeda: We're having really nice traditional drinks. So, you're having a mimosa today from champagne coming from a vending machine. We're just having a good, old classic, just whiskey soda, angel's envy today.
Tim Gaspar: Go angel's envy.
Juan Sequeda: This is great. So, you got your drink prepared now, so we can cheers.
Anna Abramova: Working on that, yep.
Tim Gaspar: Just a moment.
Anna Abramova: Yep, got the mimosa ready.
Juan Sequeda: Ready? Cheers. Cheers. Cheers. Oh, wait, hold on. What are we cheering for?
Tim Gaspar: Oh, what are we cheering for?
Anna Abramova: Oh, oh. To living on the edge, risking it all, loving life, building businesses and being happy.
Tim Gaspar: Cool.
Anna Abramova: Is that enough?
Tim Gaspar: That's perfect.
Juan Sequeda: Perfect. I can't talk top that.
Tim Gaspar: Cheers.
Juan Sequeda: Cheers to that. All right, so we got our funny warmup question of the day, which is if you could model your home after one famous building or monument, what would it be and why?
Anna Abramova: Ooh, totally would be non- practical, but the Eiffel Tower. The mini version of Eiffel Tower, I would live in that.
Tim Gaspar: That sounds cool.
Juan Sequeda: That's a really good answer because I was not coming up with a good answer, but Paris is one of my favorite cities. So, I'm going to steal your answer for that because I think... Whenever I go to Paris, I always take half a day off. And wherever I am, I'm just going to go walk to the Eiffel Tower, just go look at it. I love staring at it. I'm going to steal your answer.
Tim Gaspar: That's so interesting, right? What would I choose? You know what? I don't know that I would ever actually want to live in it, but I've always been super interested in those shipping container houses, and you stack them all together and stuff like that. So, I don't know, shipping container houses are like-
Juan Sequeda: The question was a famous building or monument.
Tim Gaspar: Oh, yeah, whatever.
Juan Sequeda: Well, you're right.
Anna Abramova: As long it works.
Juan Sequeda: All right, we'll-
Anna Abramova: They're actually super tiny, the shipping containers. They're very tiny. You need a lot.
Tim Gaspar: Yeah.
Juan Sequeda: Well, there's a lot of little, also these bars now, container bars and stuff.
Tim Gaspar: Yeah, exactly.
Juan Sequeda: Anyway, so let's kick it off. All right, Anna, honest, no BS. What's the deal with data modeling? Why are we seeing now this resurgence of it? Start with that.
Anna Abramova: All right, let's get into it. I hear actually a lot of people... I just had a conversation yesterday. In the conversation, we called it the renaissance of data modeling. That's, I guess, the wave we are riding at SqlDBM. And honestly, I don't think... Yes, it is definitely happening again, but I know it never went completely away. But hey, I'm in data modeling business literally. And yeah, definitely, it's never gone away. It's here and it's here more than ever. I think, well, at least from what I'm seeing, because I hear the phrase" data modeling" daily, 57 trillion times, every email.
Tim Gaspar: You're always picking up... Right, right.
Anna Abramova: Every email, 50% of Slack messages, they're about that.
Juan Sequeda: So, I am curious to get a little bit of history of SqlDBM. If I look at the modern data stack, we've had so many conversations about modern data stack and all different tools. Data modeling isn't one of them. And I'll be honest, you see all the old school legacy players when it comes to modeling tools, and I believe that SqlDBM is the only modern data modeling tool.
Tim Gaspar: Yeah. I mean, you hear about data modeling a little bit in the context of things like DBT and that kind of thing, but it's things like Fivetran and Snowflake. It's just not enough a part of the conversation for that.
Juan Sequeda: So, what's the history behind it? How did SqlDBM come up? I mean, for me, that is the tool that I-
Anna Abramova: You're right. Yeah, I'm glad you're noticing that too because we have that... It's like a problem, a champagne problem, if you would. We're the only player, so we don't deserve... You need two or more to deserve a category. So, no one puts us in a category. We recently became a Google Cloud partner supporting them for AlloyDB for Postgre. And they were like, " Hey, guys, so which category should we put you in? Are you observability or governance? Where do you want to be?" And same with Snowflake. They're also... I think they put us into data integration just because no one identifies modeling as a separate category, unfortunately. I'm working on this, okay? My big goal, we will have a data modeling category because it's a very important piece of the modern data stack. If you go look at customer stories, you would hear, if you ask them about modeling, they would say, " Oh, yes, we chose Snowflake. We chose Fivetran." And then if you ask them more, they'll be, " Oh, yeah, we're using SqlDBM for data modeling." Or you ask them, " Do you do data modeling?" A lot of them would say yes. It's just kind of a hidden thing that happens. No one talks about it, but we didn't invent data modeling. It's been around. Literally, all we are doing is we're more of a fresh approach with the cloud, choices for cloud data warehouses like Snowflake, the game changer. You want the best of the breed and then you want to surround it with other cloud solutions. So, we're just happened to be the only one who does it. There are good traditional tools. Don't get me wrong. They're just not cloud. They're not part of that modern story per se. Yeah.
Tim Gaspar: You'd say one of the biggest differences of SqlDBM in terms of a modern approach to modeling is a cloud- based approach, that kind of thing, or are there other things that you would say? Why a modern approach to modeling?
Anna Abramova: Why a modern approach to modeling? The reason I think here... Well, I'd tell you this. The way we're building the tool, yes, it's a developer tool. Data modeling is a very complex topic. Going back, that's probably why you don't hear a lot about this. I mean, there's a lot of domain knowledge required to speak about database structure design. Okay, boring. People are like, " Okay, I'm out." So, what we're doing, which I believe we're doing differently, is yes, it's a developer tool, but we're building with a developer, architect, modeler, engineer in mind, but also with a businessperson in mind, with the consumer layer. Because the siloed version of data modeling is just this one team that sits in there, I don't know, silo and works on data models and then just sends files to the rest of the team. I don't think that's what's happening right now. Every company that comes to us, they're like, " Well, we're trying to bring the IT and business together. We need a communication tool. We need help with communicating our models. Hence, we want to just send a link. We don't want to send files. Help." And no one wants to show them a very complex, convoluted tool with primary key, foreign key index, if the businessperson looks at it and they're like, " I don't want to touch this."
Tim Gaspar: Yeah. It's easy to be uninviting. I don't want to be a part of this. How do you make them work?
Juan Sequeda: This is an important point when we talk about being modern. I traditionally will say, " Oh, modern means, yeah, it's my honest, no BS of the modern data stack, whatever it is, it's in the cloud. Yes, that's important. And second, it has a fancy UI." All the same thing.
Anna Abramova: Yes, yes. Honest, yes.
Juan Sequeda: And if we look at the modeling tools, I think it fits those two things. But you said something very key here, which is part of it being modern. It's not just any more for the technical audience of, " Here's your table and your primary keys and stuff." Modern also means we need to be able to combine this audience of the businesspeople, the business users who understand how the business works and capture that knowledge as models and be able to go work. So, a modern tool is going to be capturing those two audiences. And I think that's something that is really lacking right now. And we do this in the whiteboard, hopefully enough, but we can't keep it at the whiteboard. I mean, the first step is do modeling on the whiteboard because a lot of people don't even do that. But you got to take it to the next level and you want to be able to bring all these different personas together. I think that's how we should start thinking about modern, is we combine all these different personas who need to be working together.
Tim Gaspar: I like that view. So, why is modeling now becoming such a hot topic? Why now? I know you've been pushing for it, and I've been pushing for it. You've been pushing for it, Anna, but why is it becoming such a hot topic now?
Anna Abramova: Well, one of the theories is that it got forgotten when the big migration happened. Everyone is going to the cloud in terms of the storage. The enterprise data warehouse happens in the warehouse, lakehouse, whatever it is, data swamp for some. Yeah, we just noticed it had a little slow time. And then once companies and people in the industry realized, hey, we're dealing with much more data that we thought, like there's more tables than your memory can retain, the modern, again, enterprise level data landscape, we're talking about a lot of data assets, a lot of tables, schemas, columns. It's just too much to look at. And if you do this, you can survive without modeling. From what I've learned, you can survive. You'll be fine. It gets you a couple of years after, months or years after the fact when you start seeing, okay, problems with performance, costs. And that's where you involve a consultant and you're like, " So, what happened? What's happening?" Then the consultant's like, " Well, where's your data model? Let us look at the model and well, which model." The model-
Tim Gaspar: Yeah, we weren't prioritizing that, or what are you talking about? What's a data model? I like Ken Graziano's comment here that in the Hadoop days, in those SQL days, things got forgotten. And I really resonate with that because I think that, as an industry, we really went more into... I think of two Ps, pipelines and prep. And that was like, we were dumping everything into the lake and we're going to build pipelines and we're going to do a lot of prep. And you got all the Alteryx's and all that stuff. But then it all came full circle, to your point, Anna. That we weren't modeling the data and then we have to pay those. Eventually, those debts come due.
Juan Sequeda: I'm curious then, what are the trends that you are seeing right now in the market when it comes to data modeling? Are people asking? It's like, yes, we need that data modeling till we finally need it, or are you also seeing people's like, nah, I don't need it? Like nah, that's-
Tim Gaspar: If people see it as nice to have.
Juan Sequeda: Is it a nice to have, or are they realizing it's a necessary thing to have?
Anna Abramova: Well, I see it all. For some, it's nice to have and they're like-
Juan Sequeda: Let's break this down. Let's break it down.
Anna Abramova: Yeah, break it down. Okay.
Juan Sequeda: I want to go to the different categories that you're seeing the people of the market.
Anna Abramova: If we talk about market as overall, there are three main categories. Even we're defining for who are we going after, who are we trying to serve, who are we trying to help? There's startups, smaller companies. They're like 10- people company. They have a couple customers and they're like, " Hey, we're..." Maybe they're digital native. Maybe they're an analytics company. So, their product is dependent on data that they have in store and provide. And so, they come to us and they're like, " Hey, we just need a little bit of help. We need a data modeling tool to support. We're just building our foundation. We need a good modeling tool, scalable to support the future of our data architecture. Right now, we only have half a modeler. We have a little bit in our Snowflake, but we know we'll grow." So, it's like for the future, they come to us. They're, " We need the basics, the minimums, but we need an online version. We need something collaborative ideally, if you integrate with the rest of our tech stack." So, it's like the very smart people, their roles could be different, data engineer, modeler, architect, head of data. Really depends. But they realize, they know from experience of all of those debts that they would have the problem down the line. So, they're trying to build things the right way from the get- go. We do see a lot of that. And the only trouble with them is, well, they don't want to spend a fortune on it. This is why Snowflake is a really good choice for them as their solution for their data warehouse because they pay as you go. So, they don't have to start paying a lot for Snowflake and then they expect the same from us. The only problem, data modeling tool, it's hard to introduce the same cost structure. So, we're per user. It's different from Snowflake. I wish it was as easy to build them on consumption, but we love startups. We were a startup ourselves one day. Maybe we still are, I don't know. It depends on which book you compare us against. So, yeah, that's one category. Sorry, if it was long but-
Juan Sequeda: No, no, this is great.
Anna Abramova: And that's my-
Juan Sequeda: Very true, very concrete. The one, the digital native smaller companies. All right.
Anna Abramova: Yeah.
Juan Sequeda: What's the next one?
Anna Abramova: Well, then you got the medium size, small and medium size companies. They don't have to be digital native. They could be automotive, insurance, financial, medical, healthcare, pharma, media, streaming. You name the industry. That's another thing. People come to us. They say, " Hey, what industry are you guys serving?" There's no one particular industry. It's very industry agnostic. And I think, overall, data industry, our set of tools, our enabled solution is vendor based. We're all very industry agnostic because every industry is solving the same problem. Would it be car company? Would it be coffee shop? The Starbucks, the Teslas of... I don't know, whatever the company is, they're dealing with the same type of troubles, if they're dealing with large amounts of customer data. So, yeah, we're industry agnostic. There, it's a little bit more mature of a situation. They probably got data modelers and data architects, a couple of them. It's maybe 10 to 20 architects. They have already data engineering department build out. They know where they are. Either they're fixing the mistakes of the past and they're like, " Hey, we just realized we missed a tool when we build this whole thing. Can you help us out please, as soon as possible?" And so, that type. Or maybe they're migrating and they're rebuilding the whole infrastructure architecture and data modeling is one of the pieces they identified they need. So, we see that a lot. Yeah, they-
Juan Sequeda: I like what you just said there. This group is fixing the mistakes of the past.
Anna Abramova: Yeah. We see that a lot. We see that a lot. Well, I think we're good. I love working in this fast- paced environment. And people tell us we're good at listening to the market and anticipating trends. A lot of our functionality that we release is based on what we find, the problems we find people have. They're like, " Well, we need to reverse engineer our existing database structure and visually show it. But even though in Snowflake it's the best practice to define our primary keys and foreign keys, we didn't do it. So, can you still help us visually show which table relates to which?" So, that's one of the examples why we introduced the feature to help them fix it after the fact.
Juan Sequeda: Interesting. So, in a way, it's like you're reverse engineering some sort of the semantics. It's almost like a lineage. These things are to be connected right here and you didn't do it, but you should have done it. And adding these connections, these foreign keys, primary keys actually means stuff. And basically, that's just the mistakes that we did and we're going to need your help to fix it. That's the second group. Is there a third group?
Anna Abramova: Third group, the established enterprises, very large organizations, again industry agnostic, any type of industry, and they probably have... The other day, I had a 900 people IT organization, 150 people data team, and they're just migrating off of their traditional tooling onto cloud native and so, that's where... And they know, they already have. So, it's like they already have all of the pieces of the process established. They just need to replace the tooling to fit the future, the on- prem, the downloadable solutions, change to the cloud ones. That's part of this process.
Juan Sequeda: Yes. So, this is a really nice categorization of these three. I'm going to go summarize this here. The one is the digital native. I'm just starting now. I want to make sure I start here correctly, building the right things from the ground up. The second one is they're already building on the cloud, but they didn't do it right from the beginning. And now, they've realized, " Oh, shoot, we should have done this."
Anna Abramova: Oops, yeah.
Juan Sequeda: Right? And that's probably, they were the folks who were the first ones in the cloud and doing Hadoop and NoSQL, all that stuff. And then you have the third which are the legacy players saying, " Hey, we need to move to the cloud. We know how to go do data modeling. We already know how to do big warehouse stuff. We need to migrate to Snowflake. And by the way, I got these legacy modeling tools too. We want to go move that, use the modern tools for that." I think that's really interesting, this categorization. I really like this. The smaller digital native, the" oops, let's fix it," let's-
Anna Abramova: Let's do this again.
Juan Sequeda: Yeah. And then the established players who are like, " I'm old, but I'm young. I want to be young."
Anna Abramova: Yeah. And you see the level. I like the summary, and you see the level of knowledge and experience progressing. Obviously, the established enterprises, they have much more resources. They would have a lot of previous knowledge pretty much on everything. So, they know what they're doing, they know. And as they're bringing more people into the team and training them, they're doing it the right way. So, there's access to that education, not so much outside in the industry. But internally within organization, they have ways of passing on the knowledge. And I really like witnessing that. You don't see that so much in the younger and smaller companies, less mature in terms of their data journey, but obviously, they're learning. They have to go outside to the market because the inhouse experience is not always there.
Juan Sequeda: And I think going to that second group, the" oops, let's fix it," here, we're seeing Ronald giving this comment, " DevOps, forget about data modeling. They wonder why their project goes off the rails." And I think it's that. It's like, " Oh, I need the observability stuff because things are breaking." And yeah, well, things are breaking because you didn't think about how things should have been modeled and moved things around and stuff. So, I think if you start investing in the modeling and the knowledge from the beginning, you're really preparing yourself for a lot of this stuff in the future. So, I think this is why I'm a big proponent of data modeling and knowledge really sets you that foundation for resilience and not just like, " Oh, here's this quick thing and here's an answer."
Anna Abramova: But it's hard. Yeah, but it's hard with the ROI. Everything in the market is about ROI. And so, yes, just because you know, okay, this is the right way to build things, your management is going to come to you and say, " Well, Juan, this is very costly. What's the ROI?" And this has been the hardest thing for us in go- to- market of a customer comes in and says, " Well, I need to pitch this internally. Can you help me? Do you have a white paper on an ROI of data modeling?" And I don't. There's nothing in the... I don't know, maybe someone already created. But last time I checked, there's no research papers on how much money a data modeling practice can save you. I wish someone went there and done some research and put it online. I know it's much easier for other vendors to do this. Like Fivetran, amazing tool, again very modern cloud, nice UI, works fantastic with Snowflake and they are... I study them and I know one of their value propositions is saying, " Well, by installing Fivetran, you don't have to hire a couple of positions. It replaces an engineering job. And so, this is your savings at the very minimum." And then this is the base bare balance. There's much more to it in scalability and repeatability and growing. But I can't say that data modeling replaces a job or a position, or I can't put a money amount on it.
Tim Gaspar: I feel like this is a very keen observation, and I think this is, honestly, a call to action for the whole data industry that... I mean, anybody you talk to, I'm glad that this is finally becoming reality, everyone you talk to now who knows things about data says, of course, data modeling is very important.
Anna Abramova: Of course, no questions asked.
Tim Gaspar: But we don't have the ROI study or the TCO study or whatever that says, " Oh, companies that do good data modeling make on average 300% more" or something like that.
Juan Sequeda: So, I'm going to take this as an opportunity for two things. One is I'm going to plug a talk I'm going to be giving this weekend at Data Day Texas titled Show me the money, where I'm literally going to be not talking about data modeling specifically, but just in data.
Anna Abramova: But I love the name. Yeah.
Juan Sequeda: It's show me the money about this stuff. So, I'll be excited. By the way, if you're going to Data Day Texas in Austin this Saturday, if you use the code, Juan Sequeda, my name, you get a 20% discount. So, for all the folks in Austin or coming to Austin, please use that 20% off. But second, totally, I get the point with the ROI. And for me, the episode we had last week with Jane was about we need to have more metaphors. We got in the metaphors to go explain things, like a lot of storytelling. And I think, for me, the metaphor around data modeling is like you're building a house and you're like, " I'm not going to get an architect and go draw this out. I'm just going to do whatever."
Anna Abramova: Yeah, I'm going to build.
Juan Sequeda: What do you mean? What do you mean? You're not going to go architect this? You're just going to go build it? You would never ever go construct-
Tim Gaspar: Just give me some wood.
Juan Sequeda: Just give me wood, give me bricks and whatever. I'm just going to go build this. And I think we just need to get to that storytelling around that stuff. And so, let's assume that I architect some things and then somebody says, " You know what? I don't want that door there anymore or that wall. I'm going to go tear it down, put the other things." It's like, " Wait, there's implications if I'm going to go do this." You would not build something and say, " You know what? Tear that down today. Put that other stuff." It's like you would not do that in construction. I think you need to be able to... I think those analogies and metaphors should be-
Anna Abramova: And to add on to that, if it's a small enough house, let's say it's a one story with two rooms and a kitchen, you may build it without the architect. You'll be like, " Okay, I'm fine." But if you need to grow that, or if you're building a bigger thing, that's when it seems like, " Okay, if it's a small thing, well, just give me some wood. I'll put it together." If it's a larger project for many more years and that many other internal teams are going to be the consumers, the standby readers of that information you created, that's when you get in trouble. When it becomes a larger landscape and scale, which you want it to become large, if you're growing, and choose me.
Juan Sequeda: So, the argument I always tell people is, it's about efficiency and resilience. And you just said it yourself; I'm going to build a small thing right now. I need to do it really fast. I don't have time to invest, and it's like-
Anna Abramova: But I get that. Yeah, some-
Juan Sequeda: And there are some things.
Anna Abramova: Yeah. Sometimes, that's all you... Yeah.
Juan Sequeda: Yeah. I mean, probably, there are moments that you have to have an ad hoc report right now because something happened and we'll just get it done. But the resilience part is, I want to be able to build in, invest such that if I put one, I want one plus one to equal three. So, I think to truly enable you, you should do that, but it's more of a future thinking and-
Anna Abramova: The quick report or quick fixes are more of a reactive. And when you're in early stage and you just need something get done, we're all been there, yes, you do that. Planning and just proactive planning and strategy is a different... This is what helps you to grow, when you're thinking ahead, not just thinking what is the problem right now I'm solving. You're thinking, " If I don't do something today, what problems am I going to have in a year? Let's work on that."
Juan Sequeda: Now, the issue is that a lot... I mean, you said it yourself right now. It's like people are incentivized more for the reactive quick. So, if we go back to the three categories that you said, maybe that first one is not... They see it, but they're that first ... Or they're like, " I get it, but I got to do this fast stuff."
Anna Abramova: I get it, but my job description is I'm responsible for three million other things and data modeling, yeah, later.
Juan Sequeda: Well, then it's the second. Is it then the second one or the second and third one that they can tell that story? That second and third category of-
Anna Abramova: Probably the third, the established enterprises with the mature data practices, the ones that successfully migrated to the cloud and have been running on it with the best practices, they would. Yeah, they're probably in the best position to tell that story of the cost benefit analysis. Yeah.
Tim Gaspar: That's interesting. Just before we move off of this value topic around data modeling, what do you find is the biggest trigger for, I think, especially the medium size cohort and the large enterprise cohort? Because I think the smaller digital natives, it's more like they know. They already have like, " Oh, hey, of course, you build the house with the architecture." But I think that especially for medium and enterprise- sized companies, what do you think is the biggest trigger around value that they're seeing around why they're investing in data modeling? Is it performance? Is it things are too slow, and that's the big trigger and the big value of how do we make this faster? Is it more people? People are wasting time and if we could save them time? What is the biggest value triggers that you're seeing around data modeling?
Anna Abramova: That's such a good question. I never asked anyone from those type of companies. I would want to find... Yeah, it's like what is the life- changing event? What changed life before and after that you went... The question we ask on demos a lot is, why are we talking? Why are we on this demo? Why are you looking at this tool? What happened internally? I guess, we just don't go deep enough in it. I know one example recently, again without company names or industry specifics, it was pretty much, " Hey, I joined this company. I'm responsible for this and this, the data team, big data, Snowflake and the previous team disappeared. And with them, the job security part, which is the knowledge part when you're the only one who knows what was built." And they're like, " Well, and there's no documentation whatsoever and we need..." And then, I guess, data model serves the documentation part a little bit to the data warehousing design. And so, for that case, it's just one, right? I'm not speaking of the industry, just one specific memory I have. And I remember, my teammate telling me about this. So, it's like, " Well, I have a problem with my job to do my job now because I don't know what was before. So, I desperately need a tool right now to just show me the map. Please, I'm lost in the forest. They hired me, brought me on the helicopter to the middle of this forest, and I really need to get out. Please, someone give me the map."
Tim Gaspar: I love that.
Juan Sequeda: That's a great... Again, back to analogies and story, that's a-
Tim Gaspar: And send it to our knowledge.
Juan Sequeda: Yeah, I think that's a great story there. As a community, we need to be able to start packaging these things up and be able to say, " These are the ROI stories." Or I mean, convince ourselves too that these are the reasons why to invest this stuff. And then work also with our colleagues outside of the data tech world and our business colleagues and saying, " We believe this is important and I'm not making this up. It's like I'm actually getting these calls from people." Again, going back to the house example, I need to make a change to this house. I don't have a plan to it. So, then what happens? I'm like just put that-
Anna Abramova: How would you know which wall is okay to break.
Juan Sequeda: At the end of the day, you reverse engineer things that happens. Well, if you had the map right there, life would have been better.
Anna Abramova: Yeah, yeah.
Tim Gaspar: Yeah. I like the analogy of a map because I think it resonates, and it creates this broader metaphor. Because one thing that we see on the catalog side is that we see people wanting better modeling, we see people wanting better lineage, and we see people wanting better glossaries or documentation. And even though those three things are a little different from each other, they all intersect with I need a map.
Anna Abramova: Right. In a broader sense, to me, data... I might be wrong; tell me. A data model is part of your data governance, part of your data catalog, and part of your data governance strategy. Apart from architects and modelers and engineers on that, remember when I said about the business side, for me, for us, a lot of times, it will be the data governance team that becomes the secondary party that's very interested because their processes really depend on what happened before. So, yeah, I see data model is a key part of an overall data catalog. Ideally, they should be connected and it's a key part of the overall strategy, data governance strategy.
Juan Sequeda: I fully agree and I think I'll tell it from... Our experience is people want to go catalog. I don't know what data I have, what tables and columns I have, but I also want to be able to extract what that stuff actually means, and the modeling actually gives me that semantics. That means like, " Oh, this table exists for this reason and so forth." And I think I have the feeling that we're now getting this pendulum, goes back and forth over five, 10 years, goes to one side, goes back to the other. We're coming from this world of NoSQL. It's not only SQL. There's NoSQL. We don't need schemas. It was schemaless, right? I mean, that's the whole point of schemaless. We want to move fast and efficient. And now, it's like, " Yeah, well, I just dumped a bunch of this shit into this lake. I don't know what this freaking means." And then they're like, "I don't know what it means." Well, guess what? You didn't have the model. So, I think-
Tim Gaspar: It doesn't even have a schema, so you can't even look back.
Juan Sequeda: Exactly. We're going to go move back. And I think now, what we got to be careful is, then we're going to have people saying, " Well, we got to model everything." And then we're like, "Well, no."
Anna Abramova: Yeah, that's another-
Juan Sequeda: You're in the pearl of the ocean.
Anna Abramova: Yeah. Life is not black and white. And so, that, I agree. It's too much because the relational modeling, you could go very, very deep of physical model, logical model, conceptual. It's very important. I get it.
Tim Gaspar: You can't even build the application ... model, right?
Anna Abramova: But if you're a startup and you want to do everything by the book, yeah, that's not the recommendation. You probably don't have time to think through every layer of it, and you model every single thing. Yes, I guess, this balance between do it but don't overdo it. Come back to it, document more. Yeah, something like that.
Tim Gaspar: Balance.
Juan Sequeda: This is a good segue to this other topic about education. How are you seeing, or even what are your recommendations for people to start learning this?
Anna Abramova: Oh, God, that's a big question. A lot of people learn this on the job, again, just from what people are telling me because it's not like... Maybe you're lucky and you took a database management class back in the day when you were getting education. And there was one class that mentioned database structures and designs and what primary keys, foreign keys are, and then you forgot about it, if you're lucky. Maybe you didn't have that class. So, a lot of people learn this on the job. That's why the industry education is extremely important. I would say there's a lot. I'm myself learning a lot every day. I mean, I have books and books on... This is, who is this? Oh, my friend, our friend, Joan Matt.
Tim Gaspar: The theories? Nice, Joan Matt.
Anna Abramova: Yeah. So, we're writing. Serge Gershkovich from SqlDBM, he's writing his book on data modeling in Snowflake. Ken Graziano has documented it all a lot, his blog articles, hundreds of webinars and talks and books also. So, you see bits and pieces of the industry bringing it together and saying, " Okay, this is not an official topic. You're probably not going to go get a master's in databases or anything like that, but this is an important piece of knowledge that we're missing." And you see bits and pieces from even technology vendors, which I think we're doing a good job in the industry trying to do educational sessions. I see we're switching. It's no longer webinars and sales pitches. This is now educational sessions, how to 1, 2, 3, masterclasses, workshops. I see that a lot. I mean, frankly, that's something we are doing ourselves. 2023 is a big educational route. Serge got this book that he's working on. I'm less of an educator. I'm more of a communicator, but I know we have a lot of great minds here in the industry, consulting companies, system integrators, vendors. We can do this. But yeah, there's not much. Even internally, I keep saying, our team internally, if we nailed data modeling education internally, we can nail it externally. Because even bringing people on and them getting familiar with SqlDBM, some, they're very talented and smart and we hunt for the best out of the best. But it's a rare piece of knowledge to find in the market.
Tim Gaspar: Yeah. It all comes back to champagne. If you can drink your own champagne, then you can sell it too.
Anna Abramova: Yes, yes. So, I guess, what-
Juan Sequeda: So, are you seeing, or do you predict that we'll have the role of the data modeler or whatever you want to go call it, this will become a more prevalent role? Or will this just be like, I also do data modeling, or will data modeling be their main focus?
Tim Gaspar: Yeah. Is it a role or a skill?
Anna Abramova: Well, I'm not the best person to give an opinion, but if you ask me, I might be biased, wrong. I think it's becoming more of a skill. Because again, if we're working on breaking the silos, then everyone needs to know a little bit more about data modeling, the basics of it, so that everyone is on the same page. So, hopefully, it becomes more of a skill that's translated from IT over to the business side and having the right tools that are business friendly. Why does everyone know Excel? Tell me. Because Excel is just so friendly for any type of a user.
Tim Gaspar: Yeah, I was thinking about this the other day, and somebody said something very similar to what you just said. And I was thinking to myself, I was like, " Is Excel really friendly?" Well, somebody sent me an Excel spreadsheet one time and I was like, "I don't know how to deal with this," and I figured it out.
Anna Abramova: But at least you know the bare minimum. I struggle with Excel. It's not my favorite tool, indeed. Yes, it's not as friendly.
Tim Gaspar: Yes, the minimum threshold of skill that you can have.
Anna Abramova: Yeah. But I guess, the barrier for entry is very low. Excel held the barrier for entry for some basic... I don't know, if it's Excel analytics or working with small datasets. The barrier for entry is very low. In data modeling, you know that... Yeah.
Juan Sequeda: You said it yourself, you don't get education. I mean, if you're lucky, if you take a computer science degree and if you took a database course, you probably took one or two lectures around data modeling there. Right? I'm seeing Ken here again, his commentary, he's taught a course for a master's in BI class that teaches data vault modeling 10 years ago. He's been doing it. That's an anomaly. That's true. Those types of situations are courses in university settings. It's not that common. And if we look at today, oh, master courses and stuff around becoming a data scientist and data engineering and stuff, data modeling isn't one of those things. But then at the same time, we're complaining why this thing breaks and stuff. Well, broke, put the models around.
Anna Abramova: Right. And I'll be honest with you, data modeling is not the sexiest topic out there. It's not the sexiest job. Like, " Oh, I'm a database modeler." It's like, unfortunately, we-
Juan Sequeda: How do we make it cool? How do we make it cool? How do we? Because we're still forming the foundation. I mean, you want to create this as a category.
Tim Gaspar: You have to call it a data supermodel.
Anna Abramova: I think it is cool.
Juan Sequeda: That was a bad joke.
Tim Gaspar: I'm sorry. Bad joke, bad joke.
Anna Abramova: I think it is cool because knowledge is sexy. Data modeling is knowledge. So, here, I said it. That's why I was going with... I got busy and forgot, but I was going with the hashtag, make data modeling sexy. So, that was the model of SqlDBM in 2022. And I think we got some success with it, but the problem is, again, the barrier for entry. If you go out to networking event or speak with, I don't know, someone from the industry or not, and they ask me, " Oh, Anna, what do you do?" And I'll usually look at them and I'm like, " Well, how familiar are you with cloud data warehouses, or have you heard of database modeling and design?" At that moment, you could see the regret in people's eyes. They're like, " Why did I ask this?" Because they got themselves in a situation where I just said 10 words, they only understood one of them. And they're like, " Well..." And that's why it's not sexy because there's a lot of the main knowledge required and basics to be on the same level to sustain a conversation. And so, to me, that's a problem. That's why it's not sexy. It's not sexy because it's complex. It's too smart. I don't know.
Juan Sequeda: Again, Ken is great, giving us great comments here, " Like a tall building, you need a good foundation to stand the test of time. Data modeling is needed for that foundation in the data world." I, 1000%, agree with this and I think this is kind of the-
Anna Abramova: I'm going to take a screenshot of that.
Juan Sequeda: This is the mindset that we need to have, and it goes back into being efficient, being resilient. If your goal is to build just a really small two- bedroom house of wood, then you don't need to go build the most foundation.
Anna Abramova: No, you're fine.
Juan Sequeda: Yeah. If you're thinking about being around for a long time, you want to be the giant and-
Anna Abramova: The resilience, as you were saying.
Juan Sequeda: Resilience, what I talked about. There's this balance between efficiency and resilience. And I think it's not one or the other. It's finding a balance. There's some things we need to be very fast about. And I think data modeling, investing in data modeling is about building that resilient foundation. And sadly, we're not incentivized to be resilient. My example always is the Suez Canal is very, very efficient, but one boat goes a little bit and an entire economy can go kaput.
Anna Abramova: My favorite meme, yes.
Juan Sequeda: Well, look, Anna, I told you we're going here so fast on time. We can keep talking about this. This is my favorite topic about data modeling, but I think it's time to go to our lightning round, which is presented by data. world. And I'm going to kick it off. First question.
Anna Abramova: Go for it.
Juan Sequeda: All right. So, I'm a big proponent of this role that I call the knowledge scientist or the knowledge engineer, which is this translator between the data people and the businesspeople. Do you see this becoming a role, a title?
Anna Abramova: Yes. That's a good... And it's very rare to meet in the wild for now.
Juan Sequeda: All right, let's work together to make this not rare.
Anna Abramova: Yeah, let's do it. I'm in. I'm in. It sounds like a kick- ass role.
Tim Gaspar: All right. A very sexy role, I think.
Juan Sequeda: We'll make it a sexy role.
Anna Abramova: That's a sexy role.
Juan Sequeda: Yeah. All right, you go, Tim.
Tim Gaspar: All right, next. Should every data engineer learn data modeling?
Anna Abramova: I'm biased. Absolutely, yes.
Juan Sequeda: I would say so.
Tim Gaspar: If you're a data engineer who's listening, how well do you think you know data modeling? Give it some thought.
Anna Abramova: Yeah. And then tell them but they're like, " Well, I'm willing to learn. Where do I go?" That's what we need to help out.
Juan Sequeda: That's another thing that needs to be fixed. This is the honest, no BS conversation. This is we need to get the community who's listening. It's like, we need to have more of this education and more of the... This is all community that we're doing here. And I'm really glad that you guys are getting a book out. I mean, we need more of these modern books.
Anna Abramova: Yeah, we're building academy also of our own. I mean, obviously, it's for tool onboarding, but the hope is to be able to cover more general topics. Forget the tool, just please learn the basics. We'll help. But it's not easy. We're still a software tool and we want to stick to that niche, because that's how we can best support. But a little bit on the educational side, hopefully. Yeah, hopefully, we'll start that.
Juan Sequeda: Well, then that leads us to our next question, which is, can we make data modeling easy?
Anna Abramova: Yes, we can. We can. It's a way which we can make it easy. We can make it cool. We can make it sexy. We can make it lightweight in terms of tooling. We can make it more automated, more collaborative. Yes, absolutely, we can, we should, we're doing it.
Juan Sequeda: That's another topic we didn't touch about, collaboration, being agile data modeling. That's another topic.
Anna Abramova: That's a lot of conversation.
Tim Gaspar: Yeah, modeling is often thought of as a little bit more of a, I create my model.
Juan Sequeda: Model, a top- down, just me. I'm the one who knows.
Tim Gaspar: And then I'm going to print it out on a really big piece of picture and put it on the wall. That's cool to do. Yeah. But how do you make it more collaborative? All right, so last question for you, lightning round. Today, data modeling is often more strongly connected to the world of the data warehouse. I think, just conceptually, people tend to connect the two a lot. Do you see streaming or other modalities becoming a really major focus of modeling as we go forward?
Anna Abramova: Yeah. Why not? Why not? With time.
Tim Gaspar: Yes, with time, lots to figure out there.
Anna Abramova: Yes. Conceptually, it sounds fantastic. Practically, I need some research.
Tim Gaspar: I love it.
Juan Sequeda: Well, I mean, there's already a big uphill journey to be taken just on cloud data warehouses. Let's get that one first.
Anna Abramova: Yeah. One at a time.
Tim Gaspar: Get the warehouse bit.
Anna Abramova: One at a time.
Juan Sequeda: All right. Well, take away time. TTT, Tim, take us away with your takeaways.
Tim Gaspar: All right. Anna, you said that really, yourselves at SqlDBMS, and also the broader industry, the data industry, we really see ourselves going through what feels like a renaissance of data modeling. And the reality is it's never gone away. It's certainly never gone away for your company because you've been talking about data modeling every day. But one of the challenges is that, in the modern data stack and the way that we think about the modern data community, it's not really a category, right? And so, it stinks to be so category centric. But when you have these labels, then it starts to be easy to say, " Oh, well, we have this box or we don't have that box." Maybe you need that box. Maybe you need some data modeling. So, it needs to become a data category. And that was one of the missions that you mentioned that you're really focused on. And really, data modeling has been like... Why is it now a renaissance? That means before it was the dark ages, right? So, why was there a dark age? You talked a little bit about the data swamp, the data lake. Just there's this trend that moved away from data modeling being the focus, but then all this data accumulated. And we got to figure it all out and enter modern data modeling. So, we asked you what is modern modeling? And you mentioned that, well, first of all, modeling is complicated. It tends to be more technical. And so, modern modeling really is thinking about, first of all, what is modern data tooling? It's usually the cloud. It's a better user experience. Sometimes, it's more affordable. So, these are some of the things that are oriented around modern tooling. But also, you mentioned that we need to bridge the developer, engineer or technical person and the businessperson. And that's an important part of modern modeling, which I thought was very important. And then you talked about three key groups that are really leaning into modeling, data modeling and modern data modeling. You mentioned the smaller digital native companies. They might be analytics companies. They might be companies where data is a really important part of their business value that they provide. And these folks, they want to build something scalable. They want to build something smart. They usually have smart people, and they probably don't have enough modeling people. They can't throw 10 architects at something, right? And so, they want to take the smart approach, a technology and automated oriented approach and modeling early and often is a way that they can achieve that scalability. The next group is these medium- sized companies, industry usually specific companies. And we all decided that they're the" oops, let's fix the sins of the past" companies. So, they went down a path. They probably weren't as digital native or aren't a digital native. And they're realizing that they need to adopt these scalable, better modeling practices to keep up with their digital native peers and to, in general, just be efficient, be effective. And then finally, are these established enterprises. And they may have been doing data modeling and maybe it's already a part of what they do, but they're looking to continue to lean into that, do that more scalably, do that more effectively. So, you have these three nice cohorts here of different shapes and sizes trying to address this. So, I think that's a nice way to break it down. What about you, Juan? What are your big takeaways?
Juan Sequeda: A couple more things. One, my favorite topic right now, ROI, show me the money. It's hard with data modeling, because it's the right thing to do, but it is costly. So, we struggle around explaining what is the ROI by data modeling. I think we need more stories and analogies around this stuff. We need to tell better stories. We were talking about how the architect and the plan you're talking about. It's like I get dropped in the middle of the forest. I need to go do my job. Show me the map. I'm lost about this. We need this map. And if you think about it, if you're doing something small, following that the house analogy, if you're doing just a small house, then yeah, you probably don't need it. But if you're doing a larger project, which is going to grow, that's where you really need that strong foundation with modeling comes in. If you're doing small, you're just being reactive. It's different if you're thinking big and you're being proactive because it's going to help you to grow. But at the same time, we need to be careful about what is that balance. You don't want to go and model everything. This pendulum goes back and forth. So, I think, finally, the big picture here of ROI is balancing this efficiency and resilience. So, this is something that draws the incentives. And then finally, it's education. Many people learn data modeling on the job. And unfortunately, it's really hard to go learn out there. There's not enough. You don't learn this. And if you're taking computer courses, there's not many modeling courses around there. There's not many books. I think there's probably old school books that frankly probably you can't even buy them wherever.
Tim Gaspar: The Data Warehouse Toolkit by Kimball and people like that.
Juan Sequeda: Yeah, exactly like that. We need more modern books around that. Really excited that Serge from SqlDBM is writing a book like that. We need more of that stuff. Interesting that you've seen the vendors move from webinars to education masterclasses. I think that's a great approach around that too. And finally, we need to make data modeling sexy. Maybe this title around knowledge, the knowledge engineer and all the scientists. That may be a way to do it.
Anna Abramova: The translator, yeah.
Juan Sequeda: The translator role. How did we do? Anything we missed on takeaways?
Anna Abramova: You did a fantastic job. This is like an article, a piece of art in itself. So, thank you. I thought I can't communicate-
Juan Sequeda: ... behind everything right here.
Anna Abramova: ...the story, but sounds like this is a perfect story that they needed to be told. So, thank you for=.
Juan Sequeda: Well, we're just repeating what you said, so thank you for saying that.
Anna Abramova: Did I? Was I there?
Tim Gaspar: Thank you. And all the while drinking a mimosa, right?
Juan Sequeda: Yeah, there we go. All right, so we're going to throw it back to you, three questions. What's your advice about data, about life? Second, who should we invite next? And third, what resources do you follow?
Anna Abramova: My advice, I don't want to be obvious, so I'm not going to say the obvious thing that, hopefully, everyone got out of this conversation. You need to do the non- sexy thing. I say advice, keep learning, keep educating yourself. Any job, any topic, any time, any point in your career or life, keep learning. Well, the second one was?
Juan Sequeda: Who should we invite next?
Anna Abramova: Who should invite next? There's lots of... Someone, are you going to invite them or is he-
Juan Sequeda: We reach out. We reach out to folks. I mean-
Anna Abramova: I'll just-
Juan Sequeda: If you know them, then you should foster the introductions.
Anna Abramova: I just have three really cool female leaders in data, Veronika Durgin from Saks. She's a Snowflake superhero. I don't know if you had her. Probably not.
Juan Sequeda: She is. I'm already talking, and we are scheduling her. She will be a guest.
Anna Abramova: Yes, please. Because she's amazing at that storytelling. And I think, what is she, VP of data, I'm not sure. But she's amazing at having that technical knowledge and background and then communicating the story in business terms. So, please, yes. Yes, fantastic. Or any other, yeah. Snowflake superheroes are usually good at creating content. And what was the third question, sorry?
Juan Sequeda: What resources do you follow, people.
Anna Abramova: Resources.
Juan Sequeda: Or blogs, conferences. Where are you going, I mean?
Anna Abramova: Ooh, ooh, yes. I follow, I go everywhere where I can. Obviously, oh, going to Snowflake Summit in June, Vegas. I'll probably see you guys there.
Juan Sequeda: Yep.
Anna Abramova: Oh, we are going to this in Switzerland, skiing and data. This is in March with Leading Edge IT, somewhere in Alps. Sounds fantastic in terms of skiing and learning. But resources I follow, I started listening to your guys' podcast, amazing for any level. Internal education is important, so I recommended some of the pieces of the podcast to some of the team members. Love the stuff.
Juan Sequeda: Thank you.
Anna Abramova: Thank you.
Juan Sequeda: Appreciate that. Great and review us, please.
Anna Abramova: Oh, I will, I will. Books, yeah, as I said, I'm reading not always full book. Maybe you just start a book. Maybe you watch a couple of videos. Also, talk to people. People are the largest and best knowledge containers out there, and people are amazing. They can answer questions, tell you stories, put metaphors, and sometimes, hold your hand. So, yeah, my biggest resource, people around me, smart people around me.
Juan Sequeda: Love that. Well, Anna, before we say goodbye, just quick reminders. Again, this Saturday, I'll be at Data Day Texas, a 20% discount code using my name, Juan Sequeda. I'll be giving that talk, Show me the money. We're going to be there. A lot of our former guests will be there. So, Zhamak Dehghani is going to be there. Joe Reis with Matt Housley. We have, I think Dave McComb, Chad Sanderson, so many guests. It's going to be a fantastic event. So, if you're in Austin or coming to Austin, use that code, Juan Sequeda. Next week, we have Malcolm Hawker from Profisee. We're going to be chatting about, is MDM dead? It's going to be a good one. It'll be very controversial.
Anna Abramova: Very provocative.
Juan Sequeda: Anna, thank you so much. And as always, thanks data. world. Let's do this every Wednesday, have cocktails and chat with cool people. Anna, thank you. Cheers.
Tim Gaspar: Cheers.
Anna Abramova: Thank you. Cheers again.
Speaker 1: This is Catalog& Cocktails. A special thanks to data.world for supporting the show, Karli Burghoff for producing, Jon Loyens and Bryon Jacob for the show music. And thank you to the entire Catalog& Cocktails family. Don't forget to subscribe, rate and review wherever you listen to your podcast.
DESCRIPTION
Data modeling isn’t new. So why is it still a problem?
Maybe the problem isn’t data modeling itself, but rather there is no modern solution for companies, or the incentives are not well understood. It’s a learn-as-you-go type of thing, but that’s where the trouble lies.
Do you hire one data engineer and have them do everything? Do we encourage training in data modeling? OR… do we throw in the towel and keep doing things as they’ve always been done?
Join Tim, Juan, and special guest Anna Abramova from SqlDBM to answer these burning questions on this week’s episode of Catalog & Cocktails.
Today's Host
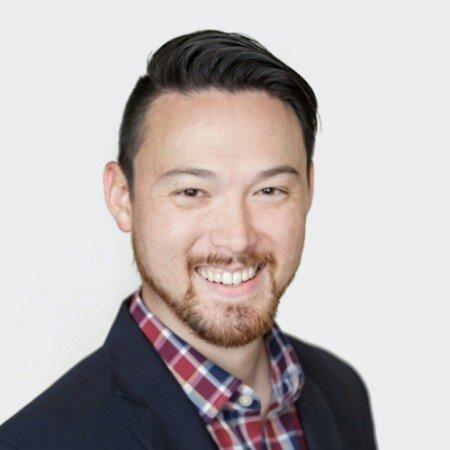
Tim Gasper
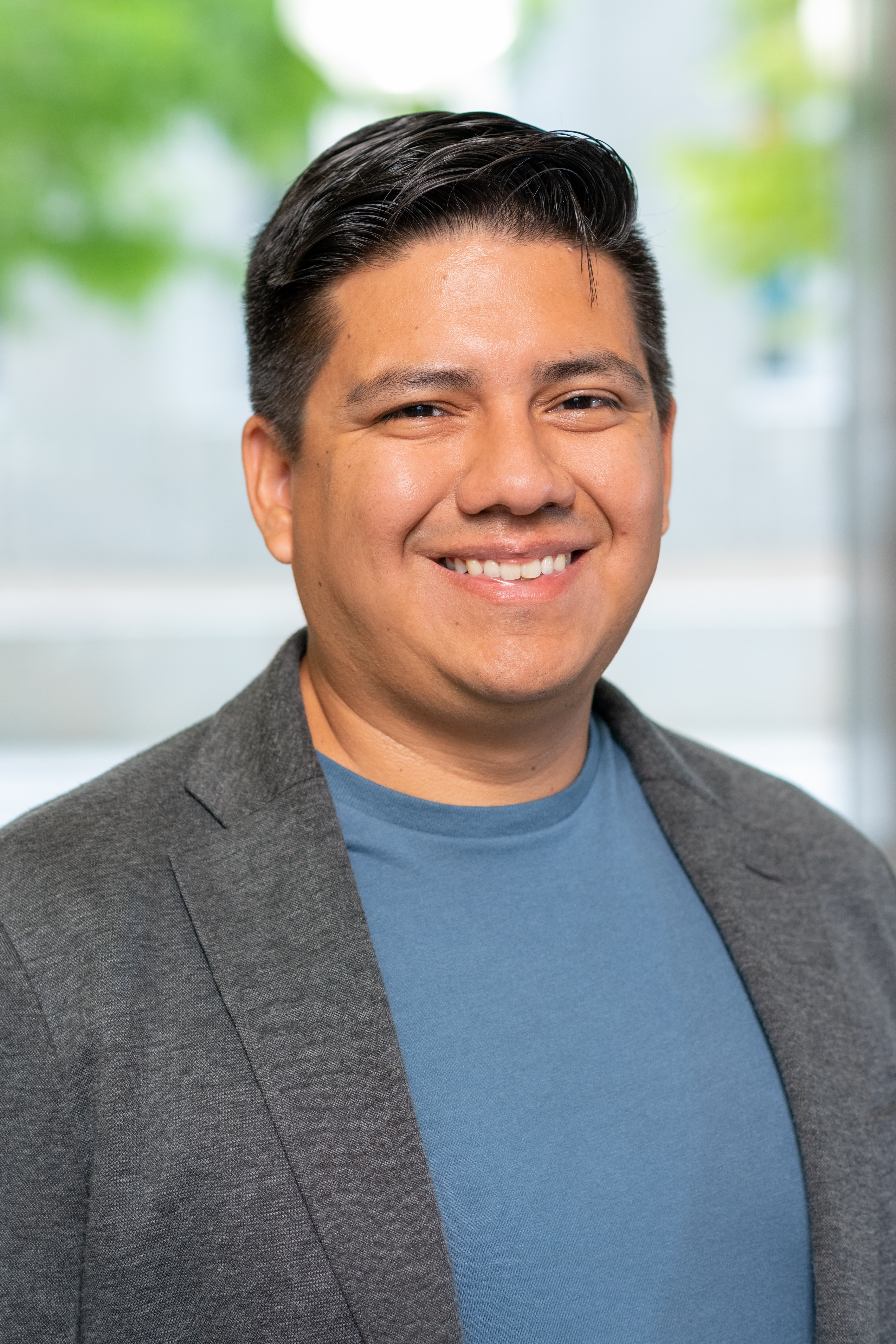
Juan Sequeda
Today's Guests
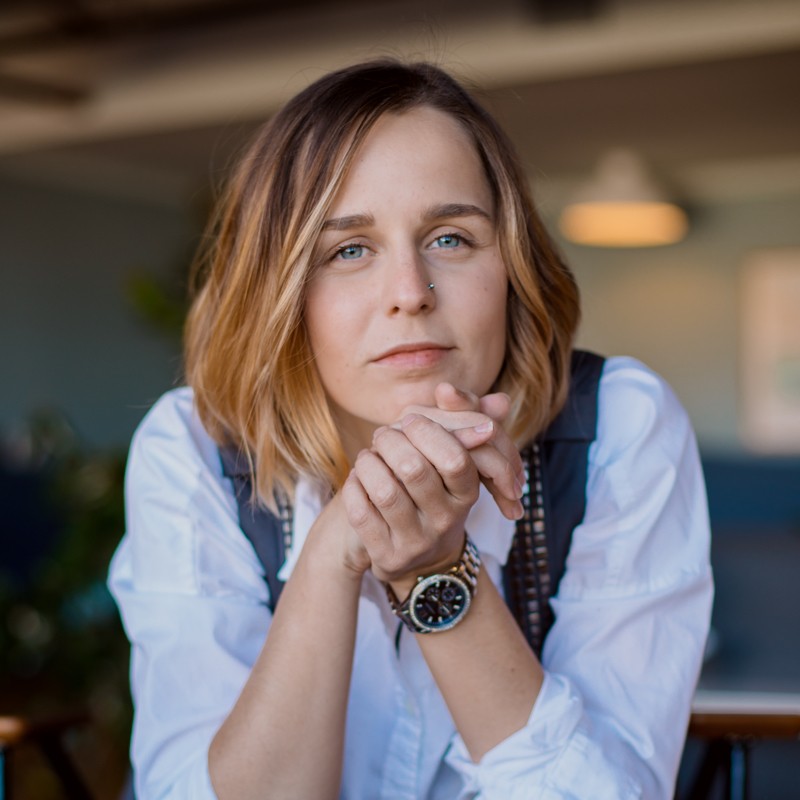