Metadata, is this a graph problem? w/ Mohammad Syed from Capco
- 0.5
- 1
- 1.25
- 1.5
- 1.75
- 2
Speaker 1: This is Catalog& Cocktails, presented by data. world.
Tim Gasper: Hello, hello, hello everyone. Welcome to Catalog& Cocktails. It's your honest, no- bs, non- salesy conversation about enterprise data management with tasty beverages in hand, presented by data. world. I'm Tim Gasper, longtime data nerd, product guy, customer guy at data. world, joined by Juan.
Juan Sequeda: Hey everybody. I'm Juan Sequeda, Principal Scientist at data. world. As always a pleasure, it is Wednesday, middle of the week towards the end of the day. Time to talk about data and one of my favorite topics, two of my topics, metadata and graphs. And for that, we're going to have a conversation today with Mohammad Syed, who is the head of data architecture and engineering at Capco. And we finally, after so long last September, a big date, 11, we finally got the chat, meet in person and chatted for many hours on this topic and we're like, " Okay, we need to have this podcast right there." So Mohammad, it is great to have you here. How are you doing?
Mohammad Syed: Yeah, pleasure to be here. Thank you.
Juan Sequeda: All right. Well, let's kick it off. Tell and toast, what are we drinking? What are we toasting for?
Mohammad Syed: So, I've got a no percent Merlot, because I'm a snob that takes my health seriously, probably. I did quit drinking, but I love the smell and taste of wine, so I stick with this stuff now. And I suppose related to that, I maybe toast to health, because I've been taking mine seriously. I hope you guys have maybe been doing a dry January or something, I know some people are. So as long as we're healthy and fit, then that's the important thing.
Tim Gasper: No, that's awesome. Catalog& Cocktails every Wednesday kind of messes up our dry January plans. But with that being said, health is really important and I'll cheers to that as well. And we got some interesting stuff here, right?
Juan Sequeda: Yeah. Well, I think we're kind of going on the other side. We got two bottles right now.
Mohammad Syed: Oh, wow. You got...
Juan Sequeda: This is an ....
Tim Gasper: And ....
Juan Sequeda: We can't pronounce this.
Tim Gasper: I don't know how to pronounce that, but it tastes good.
Juan Sequeda: But I'm going to cheer. So here, I don't know how many people know this. When we were in the midst of the pandemic and we were doing Catalog& cocktails every Wednesday, we were going to the outdoor gym. And even though we were having the cocktail, a drink, an hour later, I was at the gym. And I mean, the gym is kind of a... I enjoy working out, so I still do it. Continue to go to the gym, not only every Wednesday right now. So cheers to health.
Tim Gasper: All right. Cheers. So we have our funny question today, which is what's your go- to karaoke song?
Mohammad Syed: Honestly, I think it depends on what mood I'm in. If I'm feeling really excited, it'll be some AC/ DC, some Back in Black shout. And if I'm feeling sentimental, maybe heartbroken, it'll probably be some Backstreet Boys, maybe some'I Want It That Way' or something like that, I don't know. I'm a bit dull, I go for the classics.
Juan Sequeda: Actually, we went karaoke here as a team event a couple of weeks ago and I don't remember. Well, no, actually last time I did karaoke I was at the Gartner Conference with one of our past guests, Allison Sagraves. I even forgot what it was, but I'll tell you, I don't know what my go- to karaoke song is. I don't do it often that I have one. But I sang All The Small Things from Blink- 183. Oh, that was good.
Tim Gasper: That was good. That's catchy.
Juan Sequeda: Yeah. And then later on I did sing some Backstreet Boys. How about you?
Tim Gasper: With you Mohammad, on the classics. I would go for Bohemian Rhapsody just because I'm that guy.
Mohammad Syed: Very nice.
Juan Sequeda: Somebody has to always sing that. It's always a fun song, karaoke.
Mohammad Syed: But plus you get to go for eight minutes, right?
Tim Gasper: Yeah, exactly. You get to hang out for a while. That could be the one song and then you're done.
Juan Sequeda: Yeah. All right, well let's kick it off. Honest, no- bs. So graphs are a hot thing and I always say consciously, metadata is a graph problem. Do you agree or not? Where do you stand on this?
Mohammad Syed: Yeah, I think it's become a graph problem in the data world. We obviously spoke about this a bit in Big Data LDN. Metadata has always been a graph problem on the web as you know, because of semantic ontologies and AI and all that sort of stuff. Again, we spoke about this a bit before, but the reason being is because the web is all about discovering stuff and it's discovering stuff that you don't know is going to be there tomorrow. How can you predict webpages don't go through an architecture board, they just turn up, you've got to find a common way of finding them. And if you're developing a search engine like Google for example, you can't curate those relationships beforehand. You've got to look at how people navigate through the web and go, okay, this is how it's working. But I think in data, it's never been a graph problem before because you always knew what you wanted to do with data. So if you think about warehouses, it's like it's going in there because I know what I want to do with it, I'm going to curate it. And I kind of define what's important. We need business metadata, technical metadata. But then actually, today, some of the big banks we're working with, you don't know what you want to do with that data and you don't know what's important. You don't know what relationships matter. And obviously, what you guys are doing with data. world is creating a space where you can curate the metadata that matters and the relationships that matter. So I think it's become a graph problem because the nature of data volume, but also the kind of way we work with it. Short answer to your question, yes, it has become one.
Juan Sequeda: Well, it's interesting because I would argue it's always been, but there's some nuances here. I think it's interesting to say we just haven't seen it that way or just because the problems that were being tackled kind of traditionally didn't elude it to become a grafting. So let's brainstorm, let's talk about this. What would we call a metadata in a pre- graph world versus and how does that shift here? I mean, love to get your insights. How are you seeing this? How have you seen this?
Mohammad Syed: Yeah, so I mean, graph is a technology to enable you to manage data fundamentally. There there's a way of storing it, but why would you store it in graph versus structure? It's the nature of the data, but it's also the nature of how you want to go through it. So the reason we have different storage patterns for data is because we need to make it easy for the computer and the user to find what they're looking for, depending on how they want to navigate it. So in a pre- graph world in data, because obviously most of the stuff we did was warehousing and analytics and stuff like that. It's not a graph problem, you kind of say, but maybe you can disagree. But I've got this data, it has a meaning. I know what I want to do with it. I'm going to architect it up top, I'm going to build some models, I'm going to curate it. I know how it's supposed to be used and before I let my users get to it, I need to do that curation. I need to make sure that before I let it out there, it's documented quality, metadata, blah, blah. So people can use it and access it. But that's all based on the assumption that what matters, what you want to do with it, how's it going to work, etc. It's like when you design data models, this is the relationship, who says whoever's going to use it? So what that means in a pre- graph world is you do your design up top, everything happens through curation, it gets reviewed, you documented, etc. But you have to do lots of work before you have metadata. You can get some technical metadata, but the really rich, meaningful metadata is all done by asking questions, interviewing people, whatever. So it's a long lead time, but you know what you're working to. But I think when you move into the post- graph world, you're saying, I don't know what I'm leading to. I don't know what metadata matters. Actually, I'm going to put it out there and I'm going to let the users use the data and almost set the requirements for what metadata matters and then enable something through a catalog or whatever it is that allows other people to access it. Because metadata that matters to person A doesn't necessarily matter to person B. And there's things we're doing with banks that I can go into as an example of where we are seeing that. But that's kind of how I would see the shift at a really high level.
Tim Gasper: Interesting. I've never heard it kind of laid out this way and it's making a lot of sense. What do you think has been the biggest inflection point that has gotten us to start to move from this pre- graph world to the post- graph world? Is it the use case is getting more complicated? Is it the volume of the data getting more complicated? Kind of curious about your takes there.
Mohammad Syed: Yeah, I think it's mainly about context and use cases. So one example is I did a lot of GDPR, BCBS. And so, we did data lineage and metadata and it's sort of like the data has to meet these standards, we have to report this thing. And then working backwards through there, let's make sure all this stuff's in place. We're now working with kind of banks where somebody in Germany is going, " Hey, there's a data asset somewhere in the UK. Can I use that? Can I build that to build X, Y, Z?" And the way it kind of currently works is someone over there sends a message to go, " Hey, can I use this data?" And the person in the UK goes, "Well, I don't know if you can use it, what are you going to use it for? What are you going to do with it? How do I know? It's not my responsibility?" And so, you think of something like a fabric or a graph that allows that person in Germany to look at that person and look at that data in the UK and go, what do they use it for? Who owns it? Who interacts with it? Is it PII? What regulations apply to it? And suddenly, your metadata, can you ask the team in the UK to curate the metadata? Everybody around the world needs to make sense of that data. No, it's not a reasonable ask. But if you provide a knowledge graph layer over that, then someone in a different jurisdiction can say, " What are all the extensible problems or implications of using this?" And then it becomes an intelligent conversation. So that's an example of where we are seeing that actually you need to have a graph driven approach to metadata done through data fabric. Because you can't do it the other way. You can't predict those needs and it's not reasonable to do it in the, " Oh yeah, sure, here's some data. I don't know what you're going to do with it, it's your decision."
Juan Sequeda: No. So I think this is interesting. The use cases have gotten more complex. So if we think about this traditionally as you said, oh, we're going to go create this data, it's going to be created for this particular use case and so forth, and that's it. But then suddenly, I would think that there's two things. One, we get more use cases like now because regulations and stuff. So then the types of questions people are asking are like, " I didn't even know I needed to this today because of this new regulation I made. So it was hard to predict what are the use cases that you need to gather that metadata. I think that that's one aspect right there. The other one I'm thinking about the inflection question and it's an equal lake thing. You said, before we knew we were structuring to do what it was for, but then we started in this world of like, oh, let's just ELT. Let's just dump it to the lake. We'll just put it there. So then now, you've actually put data that has structure and then on you're like, wait, we need to put some cords around these things and need put it back there a little bit. And for that you need to have that flexibility.
Tim Gasper: There was some inherent design to building out your schemas and your pipelines and things like that in a sort of a pre- lake world. And that got a little bit thrown out to more of this lake world. And so, you actually took a step back in terms of the work you do beforehand and the documentation.
Mohammad Syed: Yeah. Well, it's interesting cause when lakes kind of turned up, they quickly turned into swamps and then everyone basically said the same thing, which is we don't have metadata and we don't have data quality. And that's absolutely true, but the problem is what is good metadata and data quality in a lake? Because if you do it in a warehouse, well, I can define that, right? Because I know why you're using the data, you put it in a lake. The whole purpose of it is it's raw, it's dumped in there because people are going to explore it. What guarantees as the data CDO office, can I actually provide you with that data if the whole purpose of it is that, I don't know what you're going to do with it. So it raises interesting questions like, what does good metadata and data quality mean realistically?
Tim Gasper: What is a good lake, right?
Mohammad Syed: Well, exactly. What's a good lake? And it puts a lot of pressure on data people because again, if you can control the access point in the use case, and as a data person, I can put some guarantees around that. But if I've got data all over the place, how can I be responsible for the data? This is a big CDO challenge.
Juan Sequeda: So in your perspective, because I'm seeing this also kind of a lot in industries like in finance and stuff, what are the use cases that you're seeing? Let's talk about finance, that can be accomplished when you have your metadata as a graph versus if it's not in a graph.
Mohammad Syed: Yeah. So one of them is we're talking about customer actually. Because the traditional kind of way of doing MDM is data, but it's also metadata because there's information about the customer, etc. And the idea that actually you can't necessarily just have one golden record. Actually, what you need to do is build a customer graph which says across our different product lines, systems, interaction points, etc, this is actually the same person. And then there's some metadata social. So that idea of, there's one bank where, I mean, there's always this statistic we pull out, this is a shameless cap code plug. But we built knowledge graphs that process 17 billion rows of customer data every time they run. And this is across all their lines, global cap markets client. And the purpose of that is to use the graph to basically pull out tables and other stuff to go, this is how you've reconcile this stuff. This could actually be the same person. And they're using that for due diligence checks, anti- money laundering, fraud, client onboarding. They're using it for product recommendations. So that thing of connecting products and customers, which you traditionally tended to do in a curated way to go, every customer can have multiple products, you can't do it like that because the bank's too complicated. New customers are coming in and opening up accounts all the time and the way they behave and their needs, etc. So even just doing something simple, I've got a sole trader who has a business relationship, but they also have a personal relationship. They've also got wealth relationship, they've also got some trustee relationship and their circumstances are changing and they're globally. That is a graph problem. You can't solve that by curating data based on known needs. You've got to use the graph to connect data and attributes. So that's one big one.
Tim Gasper: Just double clicking on that example around, like you mentioned, you started to name off product recommendations, fraud, diligence checks, and you've got these 17 billion rows of data. I mean, you kind of mentioned this I think previously, but to kind of reiterated and make sure that I'm getting this here, those are different contexts in which you want to use the data. And I assume that in those different contexts, customer may mean something different. And therefore, the best application of that data to solve the fraud use case, the best application of that data to do a product recommendation, to your point about the golden record, it isn't one size fits all. Is that kind of a good way to summarize why graph ends up kind of fitting in there?
Mohammad Syed: Exactly. So you've got data points coming from different contexts, as in sometimes from a customer journey, sometimes from a relationship, sometimes from someone signing up online. It's coming from different contexts, but also from the context of someone who's trying to sell a product versus someone who's trying to find some AML kind of issues. The definition of customer, as you say, is different. The attributes that matter are different, but then also the other relationships. So sometimes credit information's important, sometimes regulatory information's important. Sometimes things like their citizenship or residency is important. You can't predict that, you've just got to be able to make it easy to discover and share data.
Juan Sequeda: To summarize this, it's like you are connecting so many different data points, which in reality, a bunch of observations about this thing where this thing is a customer, there's different observations, but you want to be able to put a different context lens over it. So it's kind of like this, if I put a lens over this, it's this part of the graph means this thing, has this definition of a customer. But over the same observations in the graph, and I changed the lens, it's over the same graph, but it's a different lens of it. It's a different content, different definition for it that you would use it for, again, different application. So I think that's the advantage of the graph for one. Is that an accurate assessment?
Mohammad Syed: Yeah, totally. And it comes to the question of why are you doing metadata management? Because previously it was like, I've got data and I've got to make sure it's documented properly. And that's kind of what metadata management does, make sure my metadata is good. But actually now, the purpose of doing metadata management is to make it easy to discover and connect things, which is fundamentally a graph problem. So you could argue that it becomes a graph problem because the purpose and value of metadata management is different. You're not doing it for the same reason.
Juan Sequeda: This is a key point you just made. We traditionally have seen metadata as kind of a means of documentation, right?
Mohammad Syed: Yes.
Juan Sequeda: Data dictionary, all these things. I mean, we still need that. But now it's come to the point that's like, well, I need to use that so people can find things. It's discovery. You're really care about the relationships, and that's why it becomes a graph. The search aspects is a really interesting one.
Tim Gasper: It is. And I think there's a difference here between, I think just to bring catalogs into the picture for a second, there's the simple lens that people look at a catalog and they're like, " Oh, I need to find stuff." That's sort of the very simplistic definition that sometimes people use for a catalog. But I think people who think about this more understand the value of the metadata and think of that have a different lens it feels like, which is Oh, no, this is the context around which we are trying to effectively and properly use our data.
Mohammad Syed: Yeah, a 100%. I think back to when I first got into data management and looking, I've got the big dollar book, everyone's got the big dollar book. And sorry, this isn't the criticism of anybody who contributed to that book or read it, but very much rooted in that world of you read the chapter of metadata managers like, well, there's three types of metadata. There's business, there's technical, there's operational. This is what goes in there. So as a data person, I'm like, right, I'm going to define the metadata strategy because this is the proper way to do metadata. This is what it is, and you guys are going to have to conform to this. But actually, that's the wrong approach because as you say, it's about the context in which people use it and explore it. And you'll probably end up ticking those boxes, but it's not necessarily about conforming to the standard. It's about harvesting knowledge of what data means and how it's used.
Tim Gasper: Yeah. I've always struggled with it's technical metadata, it's operational metadata, it's business metadata. Sometimes it feels like you're saying when you're building a house, you can make a house out of bricks, you can make it out of wood, or you can make it out of steel. And it's like, okay, cool. I mean, I'm glad we have those categories, but is that most useful lens?
Juan Sequeda: I like this comment that we have here, is I wish I use case...
Tim Gasper: Use cases in financial services.
Juan Sequeda: I wish the use cases in financial services were only about warehouses of lake. In the world with zero physical reality data and metadata management in a large bank is about mapping the rivers and streams up the springs in the hills. Very poetic.
Mohammad Syed: Is that-
Juan Sequeda: What are your thoughts about this?
Mohammad Syed: Well, it sounds like a very poetic way of describing data lineage, isn't it?
Juan Sequeda: Thee we go. Talk about upstream and down. Let's continue. So we're talking about why metadata is a graph, and the first thing has been about connecting, integrating data, and probably in the context. Second, it's about search and discovery. Third, let's talk about this other point about creating that map and navigating the rivers and lineage and that stuff. So I'll throw it back to you.
Mohammad Syed: Well, the map isn't linear anymore. I mean, you think about what people are building with data and analytics and all sorts of solutions. They're using data from systems that was built for, I don't know, capturing transactions and now they're trying to use it to, I don't know, predict whether somebody's doing something naughty. So the lineage across the organization isn't simple anymore, it's not a simple stream. Now you've got bits kind of coming off. And so, again, you're seeing people use graph based technology to do lineage, because again, it's not about saying it goes from system A to system B, to system D. It does, but actually, those rivers and streams, to use the poetic analogy, are constantly changing. And so, you need to understand how a certain data point travels across the enterprise and gets used, which is dynamic. You can't design that. I mean, I've used Axon and IBM and all those kinds of tools where you map the lineage, fine. You can't map that with foresight, it's always changing. You need the graph based approach to discover it in real time and go, " Well, why are you guys using it from there? Shouldn't you be using it from there?" And have those intelligent conversations as opposed to this is the lineage, right? You can't forecast that. And it also comes into the implications, because let's say we talk about scorecards and regulatory reporting, it comes from system X. That's the right place. I remember Big Data LDN, the Monte Carlo, those guys, I was really impressed with the whole observability and reliability thing. Because you are understanding the implications of a dataset changing, going missing, whatever, on a 100 things downstream that again, you can't predict. So again, this becomes a graph problem between relationship between systems and environments and end users. So again, you could argue that data lineage is fundamentally a graph problem in complex organizations.
Juan Sequeda: Yeah, 100%. I think this is something that we're realizing that lineage is definitely, I mean, people want to go see the lineage in that itself is a graph when you look at it. And then we have the two traditional use cases, the impact analysis of I change this column, how was that going to effect? And you're trying to find out the paths and then, oh, if root cause analysis, there's a problem wherever, where does that come from? And then again, it's a path. So those are effectively graph problems right there. But then what's more around lineage? Because I think we're just barely scratching the surface. Well, I hear these two topics wherever, but there's what way more that we should be doing with lineage in the graph. I got some thoughts here, but I want to hear from you. What are we missing out that we should be doing more and taking advantage of the graph structure when we're looking at metadata from a lineage perspective?
Mohammad Syed: From a lineage perspective, it's interesting. I definitely think there's something about how it's used. I think if you use graph for lineage, I think you can get into questions of what data's worth and what data's valuable and where you should be investing your resources. Because again, the whole purpose of it is your understanding what data do I have a massive system dependency on an operational dependency, but also kind of value dependency on stuff, people are building. So then you can move away from this thing of, well, we are going to plan how we're going to invest in data based on, I don't know, the guys in finance use lots of data. And you're actually taking it from, we've harvested our lineage data. You could use something like solid datas or whatever, whoever it is. And we've put it into the graph structure and now we have a relationship across environments, across geographies, across systems, across business processes, across users, across tools. And then we can start having a really interesting conversation about what data practically not matters, not the data that we get the business guys in a room and they say, " Oh, customer data is really important." That's not interesting. What's interesting is there is a major business dependency on these five data assets that go through these systems that are used by these people. Let's get that right. So I think that's an interesting point for me. The risk...
Tim Gasper: I really like the way that you're positioning that and going back to what data is worth, what data is valuable, what do you plan to do with it, the use of the data. I think this connects to something that I know for example, one of our customers is doing that's very interesting is actually they're starting to catalog the decisions and the business processes. And then when you have that context, and then by the way, that's not typical, most companies are not going to that level in terms of trying to build out the metadata, and then you also have the technical metadata and things like that. Now, all of a sudden, you can start to ask questions like, " Hey, are we making decisions off this data? Are we making the most important decisions off of this data? When some problem happens, which decisions do we impact?" I mean, that's even just one lens of it, but there's a whole world bigger than what is typically looked at, right?
Juan Sequeda: Yeah. We're in this topic of the lineage, which is the map, and I think there's different kind of, again, context reviews around that map. We can get very into the details and talk about the technical stuff, and then how do I know if something is worth it or is it valuable? One way is to identify the dependencies. I like to think about this as how complex is something. I mean, if you look at it in a graph, you're like, well, here's this node, this node represents a system, a database, a table, a view, whatever. Hey, there's a lot of things that go into it. There's a lot of things that go out to it. This is a really important thing. We should know about that. Did we know about that? Who's responsible for that? Who's a steward of this? We should maybe even simplify this because there is a lot of risk around that. So identifying those things is like there's this very important set of executive reporting that happens that depends on this stuff. Let's identify that. So I think those are just kind of literally applying basic graph techniques, algorithms to identify that. I think we're just barely scratching the surface on that when it comes to the technical side. But then if we elevate a little bit more, I've been talking about we need from data literacy to business literacy, we need to go understand more the business and so forth. You see my rants on LinkedIn about show me the money and ROI and all that stuff. Well, part of it also I think is we, I need to elevate from my technical data lineage to business lineage. I want to be able to understand and incorporate in that graph, not all the technical. Going back to the technical, let's connect the business metadata, which includes, here are these business processes, here are the decisions that are being made. Here are the outcomes that occurred. Here are the people who actually took those decisions. I mean, this is all a graph, and then here's... and so forth. And now you start making the graph much more...
Mohammad Syed: Yeah, 100%. So sorry, your connection is just slightly breaking up a bit, but can you hear me? I'm coming through.
Juan Sequeda: Yeah.
Mohammad Syed: Yeah, it's interesting. Think about how that accelerates the whole process of data governance and data protection and all that sort of stuff. Instead of going round and going, " Hey, who owns this thing? Any volunteers?" No. Actually, using that graph approach, you've got a very clear set of who actually makes decisions on this data, who actually uses it. So definitely, I can see that really drives data culture because where I've seen data culture and literacy is people do things like, let's go around and lecture everybody about the importance of data. Well, that's not valuable. That's not going to change anything. So what? But actually, if you have that kind of graph of this is who uses it, who makes decisions on it, then actually you can engage with those people on the data and the context that matters to them and go, " Guys, we know this stuff's really useful to you. We know you use it. Actually, we want to put a bit more governance around this. We want to kind of understand it a bit better, that will help you, etc." So that data- driven approach, I can see it making governance a lot cheaper and a lot quicker. So instead of doing the whole, let's go around and convince everyone data matters.
Juan Sequeda: And I want to put this comment here, let's read it out loud. It says, there are graph problems throughout at different layers, resolve our language differences, absorb tribal dialects to get to a shared understanding. Second, intersystem data lineage. Third, referential lineage. How do transformations and lookups depend on each other's across the systems? Fourth, runtime lineage, how did this version of this report come to be? Which versions and values of inputs were used this time? So I think at the end of the day, we're seeing that this is a third one that we've talked about, is there's this map. And this map can get into so many details to help us ask so many more to more detailed questions, which I think we're just barely starting to realize the questions we should ask. So we've gone through three things here. The whole topic is the use cases around metadata, a graph, so integration and search and creating this map. Is there anything else you would add here? We're brainstorming here, live around this stuff.
Mohammad Syed: Yeah, I feel like those three are a lot of meat to chew on. There's a lot of fat to chew on.
Juan Sequeda: I think so. So let's talk about the outcomes, and you already talking about this. So you're like, okay, so I do metadata graph, so what? And I think you've already touched on some things that helps accelerate data governance. I mean, right now it's expensive. And then if we can make that, automate that, make it cheaper, drive data culture is another thing you said. What are the outcomes that we're able to achieve? Because we look at metadata as a graph problem, we're able to achieve those outcomes as instead of if we didn't look it as a graph problem.
Mohammad Syed: I think the biggest thing is on the value point, I think if you most remember data management governance, fundamentally this stuff came out of a negative space as in risk avoidance. Let's avoid it being wrong. Let's avoid billing the wrong customer. But when you're moving into the innovation and the value space and how do I get data utilized and discovered and get people building it, that's when the graph approach really becomes the massive enabler. I mean, that's really the big sale of data fabrics. Is it going to make it easy to innovate and connect data and this sort of stuff? So for me, it's like it starts, I think by going, how does the way we manage data translate into what people can do with it? So if I've got a pre- graph way of managing data, what I'm doing is I'm fundamentally averting risk. And so, all of my investment decisions there, if I go to the business people and go, " Hey, I need money for a governance program, why?" The risk conversation is always easy trying to sell data governance as a value conversation, very difficult. But actually, I think if you were a CDO or a CTO, you'd kind of sit with your business people, your head of analytics, your head of digital, whatever, and you go, " Okay guys, what's the product suite you want to build over the next 10 years?" You want to build these products, it's all cutting edge, just blah, blah, blah, blah. That's not going to happen if we don't move away from managing our data in a castle where it's all locked up. And towards managing our data in a way that it makes it easy for you to build those products. So for me, I really think the graph approach is connected to value from data. That for me, is the really big benefit.
Juan Sequeda: I think we just lost Mohammad here for a second, but we'll continue here. Hopefully, he will join us in a second again.
Tim Gasper: I like this focus around outcomes here. I think that's huge, and I think it kind of brings us full circle. And then Mohammad, I think we've got you back here.
Mohammad Syed: Yes. Sorry.
Juan Sequeda: No worries. This is another great observation here, is that if, and we look at governance and metadata management, historically, it came out of the BCBS 239. The financial crisis and the financial world, and it's there through the risk. And it was kind of well- defined what you needed to go do. Here, the use case, you need to keep track of these X amount of things and you're good to go for the risk. And then I think at some point we realized, well now we need to have governance, not just for finance, for these parts of the regulations, for other types of regulations, for more things. And then basically, the world is evolving so fast that we can't keep up with it as with our traditional ways that we need something to help us to be very agile about it. And the graph gives us that nimble, agile approach to go integrate data, provide the different context around that. So if you're just kind of, I think the takeaway here and kind jumping ahead into takeaways already a little bit but it's, if you just want to go deal with the basic risk stuff, then probably don't need to think about so advanced here. But if you're thinking about I need to be not just defensive with my governance, what our friend Mark Kissen always says, " Breaks in a car to slow you down. That's what you want." Then just your traditional stuff. But if you want to be able to turn more about, have data as an enablement, breaks in a car to help me drive fast, safely, you want to start thinking about it as a graph.
Mohammad Syed: So we've mentioned before Laura Madsen, right? The whole agile data governance, brilliant book, and I-
Juan Sequeda: Agile Data governance. One of my favorite books for sure, a must read.
Mohammad Syed: ...a front bookshelf. But it's really her whole thing around agile data governance. It's value driven, it's about taking yourself away from the position of I'm responsible and towards, we are 1% better than yesterday because I'm hoping you guys understand and we're curating knowledge. The underlying architecture that supports her approach to agile data governance, I think is a graph based approach to metadata. Those two things go really hand in hand.
Juan Sequeda: So agile data governance tied together with metadata as a graph.
Mohammad Syed: Me, you guys, and Laura Madsen, that's it. We're going to change the world now.
Juan Sequeda: All right, I truly really love it here. Here's another great comment we see here, let me go read it out loud. " By mapping the money value risks, you know where to focus your finite data weapons first and next. Map issues, causes and effects on top of your lineage graft to supercharge optimization as a team. I mean, the takeaway here is that there's just so much more that we can and should be doing with metadata that by just opening it up and thinking about as a graph, we have more imagination here to things to go do.
Mohammad Syed: A 100%.
Tim Gasper: How do we get started? I think a lot of people look at this conversation even. We'll use this as a microcosm and they say, " Wow, this sounds exciting. Metadata in a graph. How do I make that happen?" What are some of your recommendations to folks who may be listening trying to figure out how they start to move along in this journey?
Juan Sequeda: Especially folks who are kind of realizing, okay, I get it. I'm in this pre- graph metadata world. How would I move to be part of this post world in the graph? I mean, based on your experience.
Mohammad Syed: I think we touched on it before, which is what are you trying to do with metadata management and lineage if it's just averting risk? I would not recommend that you go to your CEO and goes, I need a million pounds to do knowledge graphs. I think not, maybe it doesn't cost that much, but you know what I mean? I think it's like, is this challenge relevant to you? So in the last week, there's a potential client we're working with, they want to clean up a data warehouse. I'm not going to tell them to build a knowledge graph that they're metadata. They're relatively small, they just want to get a better warehouse, probably running in one of the leading vendors. But then when we go out and we talk to some of the larger banks and they're going, " I'm trying to run a data analytics function and I've got a 100 different users and how do I do this?" So then we talk about right data products, lifelong ownership of data, agile teams, whatever. Some of the stuff I've read about using team topologies for data and then graph approaches comes into it because you're dealing with so much complexity. So I think there's something about stepping back and going, this is obviously really interesting kind of topic, but is the challenge relevant to you? And I think the first 15 minutes of this, when we were talking about pre versus post graph, I think it's worth sitting down and just putting that down on paper and doing some ticks and crosses going, " Do I actually have a problem?" And then I think the other thing is who's going to benefit from this? Because you can sit in a box and build a graph, but actually, graphs don't build themselves. So if I think about Google, the reason when you Google something, it shows you other stuff that's related to it. They didn't curate that. That's based on user behavior. So the graph kind of builds itself as people use the data. So where's the area of the business that people are actually going to use the data to be able to curate that knowledge where you can go to them and say, " Guys, we're going to unleash this stuff and what we want to do is work with you to build some knowledge around how you use it," etc. So I think it comes back to fundamentally, is there a business case to start talking about it? And are there people who are going to not deliver it with you? Because when I think about data governance programs, you get people to deliver it. Oh, we need you guys to give us a steward, graphs aren't like that. data. world, it's about users. Who's going to use it, who's investing dyno? So who are those people? And what I definitely say is I wouldn't start this conversation by talking about graphs. I would start this conversation by going, what are all the stuff that we can't do with data now? And how valuable would it be if we could do X, Y, Z? Imagine a world we could do this. Is that valuable? Could we do that? And I think a mistake that a lot of data people make is they go into a room thinking, this is my 15 minutes with the COO or the CEO, and I've got to get the answer now. Actually, you don't, build it up slow. Pop in, have a conversation, lay the groundwork, whatever. You don't have to convince people straight away. I think it's about starting softly and building appetite before you rock up with a solution and a suggestion, don't rock up with a solution first. No one will know...
Tim Gasper: So basically when you're selling this internally to your organization, do the opposite of what we did in this podcast. You start with value and you work your way back.
Juan Sequeda: Actually, listen to this from the back all the way.
Tim Gasper: Yeah, listen to it in reverse.
Mohammad Syed: Just listen to this in reverse, right?
Juan Sequeda: Yes, exactly.
Mohammad Syed: Like a secret tomato soup recipe or whatever you feel like.
Juan Sequeda: This has been a very thoughtful kind of conversation. And before we head to the lightning round, I do want to be very honest and no- bs here. People listening, they're like, " But wait, we know this is data. world. Are you crossing the line right now and kind of being salesy around this stuff? Because we know data rolled markets themselves as being a data catalog power by knowledge graph." And I want to be very open and explicit and honest with everybody here. The reason why I wanted to talk about this with Mohammad is that this is something that is just in my freaking gut. This is in my passion, my heart, and I genuinely believe that this is the right thing to go to. And to be very honest, that's why I'm here at data. world because we are very aligned and I continue to be here. So I don't want people who are listening to this to think about this as, oh, this is a salesy thing right now. I am here, I am coming in. I'm being extremely honest, saying that I genuinely believe that this is the right thing to go do or how to go manage data. And Mohammad, as you said, if your goal is just to go do just very traditional risk and compliance and it's it, then no, don't do this. You're going to overcomplicate. So I'm actually going to tell you don't do this stuff. But if you are really thinking about what I always call focusing on the known use cases of today, and you need to deal with the unknown use cases of tomorrow, then just knowledge graphs give you that flexible architecture that gives that flexible agile opportunities to go deal with the knowns and the unknowns. And at the end of the day, I think the Corona wrapped us up a little bit is this is new tech. Yes, it's scary. Talk about RDF and Sparkle and things news, but I mean, I think this is kind of why we've kind of been stuck lot ourselves is because people are kind of afraid of new tech in a way, but at the same time, they jump on all their bandwagons and stuff. So here's my call to action, my requests, my pleading, please open up, be more curious and look at all these graph technologies, these semantic technologies, the RDF and Sparkle and all. Because I think this genuinely, is a way how we should be managing data. And you started out Mohammad about this is the web was all about no, you couldn't control what websites you're going to. You have to decentralize this. And we've now realized that governance can't be living this ivory tower. You need to be able to have this way of managing and decentralized. There are things that need to be centralized and so forth. But you need to have this ability to be very agile and decentralized and graphs enable that. And with that, I'm going to get off my soapbox because I can keep right here. Let me pass it on to you, Mohammad, before we go to our lightning round. Any final thoughts here?
Mohammad Syed: No, I think you're right. I mean, obviously, we're focused a lot in the FS space. Most of the clients are really kind of big clients and they use all the tools you've all heard of on the Gartner thing. But I've definitely seen data. world come into some of the clients we're working with, some of the RFPs, etc. And it's because of this approach to metadata management. It's because they want to move away from curating metadata, which they can't do anymore, and they need to move to, we're trying to create a digital exchange. We're trying to create marketplace. We're trying to create monetization of our data. We can't do that by just manually curating metadata. We have to do that by building a graph that people can search and they can find meaning, and they can define what the data's worth. So I'm seeing it all the time, and I think you guys are ahead of the curve, there's no doubt about that. So keep talking about-
Juan Sequeda: I'll probably come across on this as non- salesy thing, but I want to respect our listeners who really value us for being non- salesy here. But again, it's the passion we have. This is the right thing that we do.
Tim Gasper: It stands on its own merits.
Juan Sequeda: Yes. With that lightning round, lightning round presented by, Hey guys, we've got to thank data. world who lets us do this carbon freaking whiskey. On Wednesday, we do this. So all right, I got to kick it off, lightning round. Question number one, fast- forward 10 years, is there a metadata graph at the heart of every enterprise's data platform?
Mohammad Syed: No.
Juan Sequeda: No. I love this area here. Okay, expand on that a little bit. I need to...
Mohammad Syed: For the reason I think we said, which is, I mean, enterprises can be small, medium, large. You don't need it in every single place. I can imagine in large enterprises, some people taking a more traditional approach in some areas and others saying actually, whether it's in a region or whether it's in some kind of environment where we collaborate and building stuff, we've chosen a select set of data sources that we bring into a graph because actually we want to do some interest stuff with that. So I think putting all of your... Like when people say, get all the data governed. Really, you need to see that? So I think there's different approaches for different bits, and not all of it needs to go off even in a big enterprise. So, no.
Tim Gasper: It's not going to be one size fits all.
Mohammad Syed: No.
Tim Gasper: That makes sense. I think that's very fair. And 10 years, although it feels like a long time, isn't as much time as one might think, right? All right. Second question for you, instead of yes or no, this is actually going to be multiple choice. So we talked about an integration, golden record and context kind of category of things. We talked about search, and we talked about the map and the lineage and sort of the relationships. If you had to pick one, which of those is the most important?
Juan Sequeda: Which one?
Mohammad Syed: I actually think some combination of the first two. I think the third one, I understand from the perspective of people who work in data, IT platforms that I want to know where all the data goes. That's great, and I'm not downplaying that. But the real value is in the combination of the first two. So the example of the customer that I talked about, I mean, how many millions, billions have been spent globally on trying to master customer data? And every five years, the same companies buy a new MDM tool to master. It's like, how many you guys solve this problem already, right? How much have we spent on this? So I really think that that customer thing of connecting data and searching through that and discovering your version of customer with your attributes that make sense for you, that is just super powerful.
Juan Sequeda: All right. Next question. Defensive use cases, risk protect, security. Offensive use cases, new products, new insights, new value creation. Will the offensive use case ultimately win out?
Mohammad Syed: For graphs, yeah. Because actually, I think to solve the risk use case, to be fair, graphs make it a lot easier to identify risk. What's the risk of this data? I can know that, but I can see the implications. So I think graph makes it easier to detect the impact of risk, and it makes it easier to understand the value of data and unlock the value of data. But I think there's a big change for me, which is if you want to have the graph conversation, I think it's easy to talk about manual approaches when you're talking about risk. Because like, okay, there's a fine, let's avoid it. I think going and saying, let's build a knowledge graph to avoid a fine, it's like, do we need to do that? But I think if you have a value focused conversation around graph, you're suddenly saying, actually, there's a lot of valuable stuff we could build there. And there's all these products at the moment that you're not going to be able to build and you want to do a digital defi exchange, you need graph metadata. So I think it's a value driven conversation, which is good. So as data people, we want to have value conversations. We don't have risk all the time.
Tim Gasper: Well, in your comment there about risk just made me start to have a bunch of thoughts around the interplay between graphs on one side, and then policies, processes, workflows on the other side. But that that's going to have to be a topic for another day because I think that there's a whole interesting thing to explore there. All right. Last lightning round question for you. Do you need to have a graph expert or be one to get started with metadata and graphs?
Mohammad Syed: Depends on what you mean by get started. I think to get started on laying the building the appetite, which I think is where you start. I don't think you need the graph expert. I know this is the no- salesy show, but I think you need someone more like me, quite frankly, right? So sorry, apologies. But I think you need someone who could just talk and you could be someone in the business. Maybe a business sponsor, maybe somebody on the business who gets it, who can advocate for you. I always think that's how you get started. But if you mean get started as in developing stuff, then you need to get a graph expert in to explain to you what's the challenges. And also how much can off in one go realistically, how much data sources and challenges do you actually want to take? Because there'll be a question, eventually somebody will come and say, what are we doing with this? And if you've bitten off more than you can chew, you're going to be in trouble. So I definitely take some technical advice.
Tim Gasper: No, I think that's some really good advice. And every once in a while even we see folks who will say, " Oh yeah, we want to put everything in the graph.' It's kind of to your point about I want to govern all the data, and it's like, woo. So you want to go straight for that? Well, why don't we focus on the use case and what's needed for the use case? So I think that's valuable advice. And it sounds like you can, if get started means more the business angle, like, " Hey, let me build excitement, sponsorship, figure out the right value in use case," it sounds like you don't necessarily need to be a graph expert. If you want to take things to the next level, you really want to kick off some development and things like that, then having that skillset set becomes really important.
Mohammad Syed: Well, we'll keep markets on standby. All right.
Juan Sequeda: All all right. We got T time. Tim, take us away with takeaways.
Tim Gasper: All right. I think one of the biggest things that we started with here was around the fact that metadata, well, maybe didn't always used to be a graph problem. I'm sure you can argue that graph could have been interesting back a while ago, that it's now becoming a graph problem. There's sort of this pre graph world and this post graph kind of world where now it is very valuable and things have shifted a little bit over time. Metadata on the web always was a graph, and now we're starting to look at how it can apply to our own data infrastructure, data architectures. The web is really about discovery. And similarly, in our own environments, this helps not just with the discovery, but also trying to create more from the data. Previously in the pre- graph world, you had the data and you had a specific use for that data, specific meaning of that data. Usually, things were in a much more structured environment and based on that, a set of assumptions you kind of designed for certain use cases. And so, things were a lot clearer. You're designing upfront, kind of curating upfront. Usually, you're pairing it with certain workflows and documentation. So metadata was a little bit more cut and dry, a little more structured in that environment. But then once you started to get into this situation where you don't necessarily know what metadata matters, really, the users in different use cases are setting the parameters for the requirements, not the designer of the data pipeline and the applications upstream. And that metadata that matters to person A or use case A may not matter to person or use case B. Now, all of a sudden, enter graph and now graph can have a really big impact here. And this inflection point happened where you've got sort of different context, different use cases, you've got different regulations coming in, and those add additional kind of context on top of this, you've got different sort of structured versus unstructured data lakes versus warehouses, lake houses. All of this environment creates this multidimensional matrix that we have to navigate and hey, there's a technology that helps us with that. It's called a graph. And so, I think this set up a really good kind of context for why maybe graph didn't have its moment in the world of metadata management before, but now knowledge graphs, metadata, and graph is really having a bigger impact. And Juan, what about you? What were your big takeaways?
Juan Sequeda: So first of all, I love how we got to these three very concrete use cases we could take away. So what are the use cases of having metadata in the graph? Number one, it's about integration and context. We can't to have consider anymore having the golden record of a customer. You need to have that customer graph because there's just so many relationships. This is our dynamic, circumstances changes. You need to be able to have that support, being very dynamic and deal with the different contexts. You can build a graph that pulls in all the observations together and have different lenses, different contexts around that. So that's number one. It's connecting, integrating data and providing different context. Second, it's about search. So before, metadata has just been focusing on documenting, but now we're seeing that metadata is being used for search and discovery and that effectively is a craft problem. It's about relationships. I mean, look at Google, you Google and you search, you get your knowledge panel, which is a graph and everything they Google's knowledge graph. And third, it's about really creating that map. And the issue is that the map isn't linear anymore. Again, you don't just have the simple stream of data, the simple stream that goes into the data warehouse is done. It's like, again, things are very dynamic. Things start changing it. People go, branches all over the place. It's not just system A to B to C. It gets very much more complex. And that graph helps you navigate that map, right? I can understand now, is my data worth something? What is it? I can check the check usage of it, understand the dependencies and figure out, help me go plan things. I have a very detailed map, so I love those three things that we came up to. And then talk about the outcomes. Basically, so what? Show me the money. This next part is how you should start the conversation with the sponsors is biggest thing is value. So if you're focusing on metadata, this is for dealing with risk, then you probably don't need the graph. But you are going to start talking about value, starting going, doing valuable things with your data, generating those new products. That's where the graph is going to come in. And definitely it does help to accelerate your governance. It's helps to accelerate your risk. And I'd like how you said it, agile data governance and metadata in a graph. That's a dangerous and perfect combination you need to have. And I think third helps you to drive that data culture that you need to go do. And finally, how to start? Well, question is what are you going to do with your metadata? I mean this is the thing. If you're just focused on your traditional known use case of risk, maybe you don't need that. But if you're trying to run a data analytics function that has hundreds of users and you're generating data products, that's something is going to lend itself to a graph. Who's going to benefit from this? Who has this? Who's putting skin in the game here? At the end of the day, this knowledge and these connections don't happen automatically. Somebody teams need to be part of it. They need to go build and add to the graph. So who's going to be part of that? And then finally, don't start a conversation with your executive sponsors with technical things about graph, right? Start with this last part of the podcast and then go the verse. You do. Anything we missed? Anything to add?
Mohammad Syed: No, I think that's it. I think what's really exciting is that we often talk about data in a risk avoidance conversation. I think the graph puts it squarely in the value space. And that's where I want to be. I don't want to be talking about how data's going to avoid it. I don't want to talk about how data's going to help you build cool stuff. And I think that that's where the knowledge graph comes in. That's really exciting.
Juan Sequeda: Awesome. All right. We'll throw it back to you to wrap up three questions. One, what's your advice about data, about life? Second, who should we invite next? And third, what resources do you follow?
Mohammad Syed: Okay. I mean, we covered quite a few data advice. Maybe I'll go down the life advice stuff. I don't know, maybe I'll be a life coach. I try and stay kind of organized with my time and there's a thing about habits of effective people. I'm sure you've read the book, about your sphere of influence and how if you allow yourself to just get sucked into stuff that you can't control, you're going to be all over the place. You've got to defend your square. So recently, there's a CDO I'm kind of working with, who's really frustrated, basically doesn't know what his job is. And we've spent the last two weeks going, this is what you can do in this business. Given how it is and helping him present to his COO to go, " This is what I think my job is. This is what I want to responsible for." So I think on a personal level, I think your space, what are you responsible for? Well, what's the value you are going to add? Where are you not in competition with others? And how can you brand yourself as that deliverer of value without making yourself responsible for all the noise? And that was my personal advice to people and danger-
Juan Sequeda: Know your space. I love that.
Mohammad Syed: And know your space.
Juan Sequeda: Great advice. Who should we invite next?
Mohammad Syed: Actually, I think on that... So almost, there's two people I kind of for quickly thought of. So there's a guy, Sam Sharma, he was having drinks with us in London the other day. And the reason I mentioned him is because his podcast is all just CDO people. It's not consultants like me, it's all people who are actually kind of doing the job. It's not people who talk rubbish like me on the podcasts, these are people who do the job. I think he'd probably have some really interesting insights about the struggles people are facing and whether people have tried to have these conversations. And because again, sometimes as a consultant, I can come in and kind of say all this nice stuff, but your world is the reality of your realm. So I think that's interesting. There was also a chap called Eric Broda spoken to a couple of times and he put out some interesting stuff about using team topologies for data. And so we had some really interesting conversations about moving away from thinking of the data organization as BAU, like head of governance, head of engineering and thinking of your data model as team topologies, which is how do I set up teams to go build some stuff? So I think that's a really interesting thing that he and I were talking about collaborating on, which is data op models, which are all about delivery as opposed to... So I think that is an interesting topic to get into him.
Juan Sequeda: That's great. I've been talking to Samir and I think we're going to figure out how to get him on the podcast too. So, yes. And then Eric Broda, I've been seeing a lot of his content. I'm really excited you brought him up. I think we definitely should have him on the podcast. So Eric, listen, we're we'll be reaching out. So finally, last question. What resources do you follow?
Mohammad Syed: I mean, I listen to you guys, so...
Juan Sequeda: Thank you.
Mohammad Syed: I do go to conferences, but if I'm honest, a lot of conferences just like vendor speak and it reminds me of that stuff I used to work in previous organizations where people get their 15 minutes to present their projects. Great, so what? I think books and honestly, people, I think the most valuable lessons I've learned is just meeting people and listen to them. And it's not formal presentations like it's graphs in real life. It's the power of network, the real value. Let's get out and talk to people, build a graph of your life.
Juan Sequeda: Your build your graph of people. There you go. Well, before we say goodbye, just a reminder, next week we have Brian T. O'Neil, who is the Founder and Principal of Designing for Analytics. I love following him on LinkedIn. He talks all these things about user experience when it comes to data products. And we're going to be talking about his biggest pain, which is adoption. Why is it so hard to have adoption for the data products that are being generated? So I'm really excited for that conversation next week. And with that, Mohammad, thank you so much for just having this awesome conversation about the two topics that I'm so passionate about in life. Man, there's many more topics that I'm passionate about, metadata and graphs. Thank you so much. Cheers.
Tim Gasper: Cheers.
Speaker 1: This is Catalog& Cocktails. A special thanks to data. world for supporting the show. Karli Burghoff for producing, John Williams and Brian Jacob for the show music. And thank you to the entire Catalog& Cocktails fan base. Don't forget to subscribe, rate and review wherever you listen to your podcast.
DESCRIPTION
Metadata management has been a topic for a while now. Lately, the industry is pushing that metadata is a knowledge graph problem. What does metadata in a pre and post graph world look like?
Join Juan Sequeda and Tim Gasper with special guest Mohammad Syed, Head of Data Architecture & Engineering at Capco to chat about metadata and knowledge graphs.
Today's Host
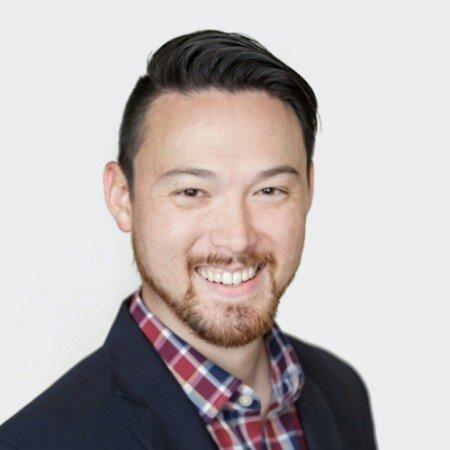
Tim Gasper
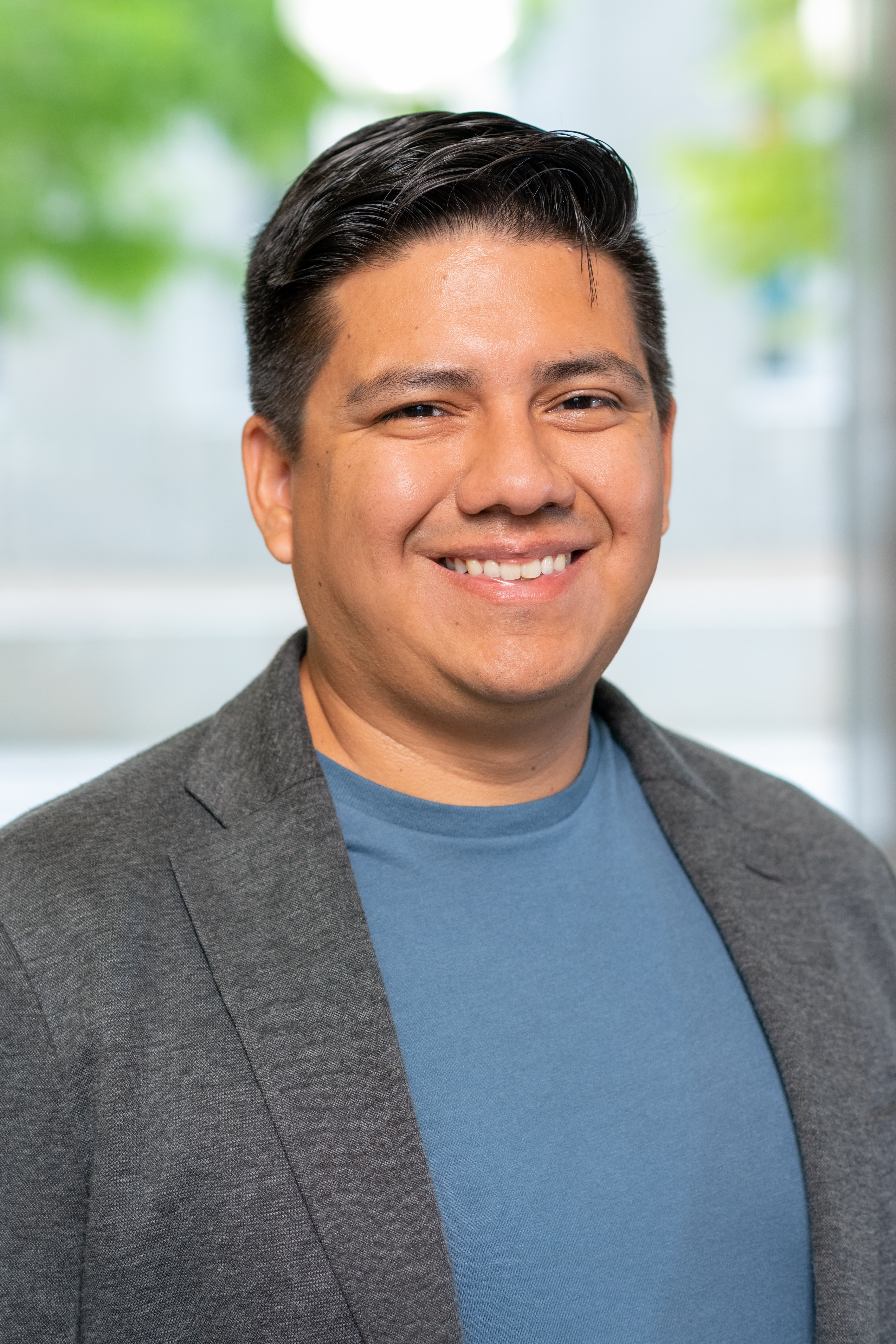
Juan Sequeda
Today's Guests
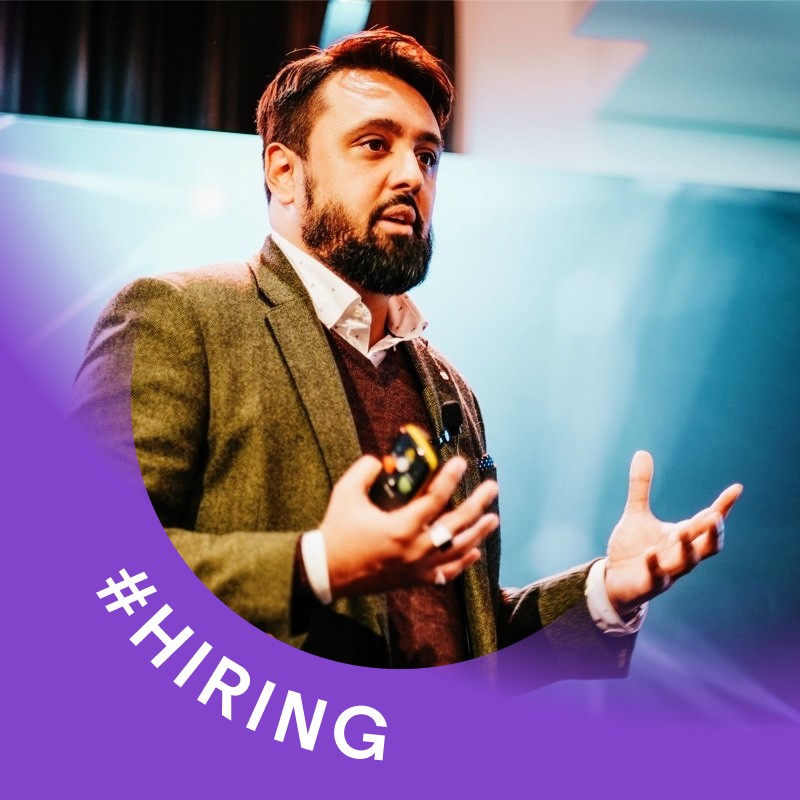