Put the Business in charge of their own data w/ Gabi Steele and Leah Weiss of Preql
- 0.5
- 1
- 1.25
- 1.5
- 1.75
- 2
Speaker 1: This is Catalog& Cocktails. Presented by data. world.
Juan Sequeda: Hello, everybody. Welcome. It's Catalog& Cocktails presented by data. world. We are coming from you live, from Austin, Texas. I'm here. But where the heck is Tim? If you're watching us right now, you're seeing that there's an empty screen right there where Tim should be and I understand that he got locked out of the office right now. But hey, we always do this live at 4: 00 PM so if Tim's not here, the show must go on. I'm Juan Sequeda, principal scientist at data. world and it's always a pleasure to have Catalog& Cocktails. It's Wednesday, middle of the week towards the end of the day and we are that honest no BS non salesy data podcast and I'm kind of trying to make some space and get Tim to get here, but I don't know. Tim will join us in any second right now. But for now, let me go introduce you to our guests today. Our guests, Gabi Steele and Leah Weiss, who are co- founders at Preql. Gabi, Leah, how are you all doing?
Gabi Steele: We're good.
Leah Weiss: It's good to be here.
Juan Sequeda: I don't know where the heck Tim is, but I think this is the first. I think we said this is like a hundred fourth episode and I think it's the first one that we're doing it without Tim. I kind of feel a little bit guilty right now and he's still locked outside of the conference room. But anyways, Tim, are you listening to us at least? You should be a live listener right now. But anyways, let's kick it off. What are you drinking and what are you toasting for?
Gabi Steele: Yeah, we're excited to be, I think Tim rushed away to get a drink actually and that's what has delayed us today. But Leah, what are you drinking?
Leah Weiss: Okay, let me get prepared here. I shared with this crew earlier, it's not very exciting, but I do have COVID so I've lost my sense of taste and smell. I'm going to do a little live experiment. I have a sour beer.
Juan Sequeda: Are you a sour fan or you're like trying to...
Leah Weiss: I love sours.
Gabi Steele: She is.
Leah Weiss: I'm thinking that I'll be able to detect it, but it's unclear.
Juan Sequeda: Right, so a live experiment right now. Go taste it and see what it tastes like.
Gabi Steele: Yeah. What is it just bubbles? Or are we getting something?
Leah Weiss: I'm getting a little sour. I mean that's probably as much as I should drink, but yeah.
Gabi Steele: At least you tried it, value? Yeah.
Juan Sequeda: I believe that the COVID is going away. That's a...
Gabi Steele: It's a good sign. I'll check back in on it tomorrow. Yeah.
Juan Sequeda: How about you Gabi?
Gabi Steele: Now I'm feeling like I should have also done a sour beer because we've got some in the office. I'm in the office drinking peach sparkling water from our bevy machine that we love very much. But it's late in the day, not late enough to go. But I didn't know that you were doing the sour beer, Leah. I think we have some really nice... Oh, Tim. Tim.
Tim Gasper: Right on, tour. I made it.
Juan Sequeda: This is truly honest. No BS.
Gabi Steele: Was it worth it? Yeah.
Tim Gasper: This is a real show everybody.
Juan Sequeda: All right.
Gabi Steele: ....
Tim Gasper: I can't believe that it locks.
Juan Sequeda: What are you drinking Tim?
Tim Gasper: I am trying to relive the days of summer with this Summer Bliss New Belgium beer here. I'm longing for those very hot Austin days... No, not really. I'm actually a loving fall, but this is a great beer.
Juan Sequeda: I'm having, I've made this up. It is an Austin light whiskey, I forget what it was. I mixed it with a Licor of 43, which is a Spanish liqueur. I put a little bit of bubbly water because that was just it, just pure liqueur. It's actually really, really nice. I'm going to have to give it a name. I'll come up with a name. But anyways, let's go. Cheers to what? What are we toasting for?
Leah Weiss: I'd like to toast, Tim. Even though he was late and delayed to podcast. Because we met at a conference many years ago and it's nice that we were able to stay in touch and reconnect. So thanks for bringing us all back here.
Gabi Steele: To Tim.
Juan Sequeda: To Tim.
Leah Weiss: Thanks so much.
Tim Gasper: Cheers to Tim here.
Leah Weiss: Glad you made it. Yeah.
Tim Gasper: Thank you. Glad I made it.
Juan Sequeda: All right. So cheers to Tim. All right, warmup question. What is the wildest or weirdest thing that you have seen while stopped at a red light? You're New Yorkers, so I think you have a lot.
Gabi Steele: Well, maybe Leah, you can kick us off. Because I know that growing up in Colorado... We always discuss who's the better driver of the both of us. I'll give it to you this time.
Leah Weiss: So it's on the record. I did grow up in Colorado and I went to college in Maine. There's a lot of wildlife out there, but I have a memory from my days in college when I was driving and I saw a porcupine on the side of the road and I kind of just started following it because it was such a novelty to me. Then I actually got pulled over because I was driving a bit erratically following around the porcupine and had to explain, nothing strange was going on. I was just wanted a closer look.
Juan Sequeda: That's a good one, Leah. How about you Gabi?
Gabi Steele: Yeah, I'm trying to think of something I was going to qu similarly during my college years, I was driving a lot in Canada. I did my undergrad in Canada. I don't think it was ever like a moose that I saw. Because a moose, it's a pretty scary thing and it would've been a big deal. I think it was just a big deer with antlers. Similar to Leah, like slowing down, I wasn't pulled over but it was a little bit stressful. The wildlife thing... We're happy to be living in New York now and I enjoy not having to deal with this, but big deer, big antlers could have been a moose. Not sure.
Juan Sequeda: Go ahead, Rom, if you're there're on the chat, go ahead and share with us what's been the weirdest, wildest thing you've ever seen in a red light. How about you Tim?
Tim Gasper: Well, at a red light. One time when I was stopped at a red light, I think somebody was having a really bad day and just completely lost it. They like bar and took off all their clothes and was dancing in the intersection. That caused such a ruckus, people couldn't drive through because that person was blocking everything. That was the most bizarre thing I have ever seen in my life.
Juan Sequeda: Mine is, I used to live in Columbia for 10 years and I was growing up. There's just some folks put a tight rope on top of those lights and they were just kind of, that's how they're trying to get money, right? Just doing a tight rope on top of the cross section. That was crazy. That was one of the craziest things I've seen. But all right. Well, all right. Ready for the warmup. Let's go dive into our discussion. Leah, Gabi, honest no BS. How do we put the business in charge of their own data?
Gabi Steele: Cool.
Leah Weiss: That's the question. Yeah.
Gabi Steele: Yeah.
Juan Sequeda: You do the part.
Gabi Steele: Leah's going to start us off, yeah.
Leah Weiss: I think this is the thing that has driven most of our careers. It's really the problem that we've been trying to sort out and we've attacked it in many different ways. Just sort by way of background, I was an early employee at WeWork, got there before there was a data team and spent some time sort of setting up the initial data infrastructure and many versions of it as well as the data team. Was always sort of burdened by this gap. I was the data team of one for a while, which was a very particular experience, I'm sure a lot of people can relate to. You hold all of this institutional knowledge, you sort of know how things work, how we're encoding business logic and the value of the data that you have access to, but all of your friends scattered around the business really don't. It's a big job translating, making that accessible and trying to bridge the gap. We've done a few things to get at that problem, and maybe Gabi can tell the story of when she joined WeWork and we sort of came up with a wacky plan that went pretty well to try to get on it.
Gabi Steele: Yeah, we took it pretty far. I met Leah at WeWork, I joined to lead data visualization. I was coming from more of a design background. I had been at the Washington Post most recently before that, and a lot of my career was spent designing data and creating these visual representations of things that would take months and months to deliver, especially when it comes to sort front end data visualization pieces. Then they live for a few months, the data changes. Does it actually solve a problem? Does it make an impact? A lot of different minds go into creating these things. So similar in some ways, but also a different perspective. When I joined WeWork, there was a culture training program that onboarded data people who were coming into the company that tried to help them solve real business problems with their data. Because one thing we've seen time and time again is data engineering teams sitting in one corner of the company doing a lot of different work to build these robust data infrastructure and pipelines and all these things. Then the rest of the company kind of living without answers to a lot of their questions and understanding that process. Our approach was to take this onboarding training program and convert it into something that anyone could access across the company and train them on thinking about how could you access the company's data to solve 10 million dollar problems for the business. As we know WeWork was bleeding more than 10 million dollars at the time. There was a lot of different things going on there, but there was so many opportunities for us to bring in people from all different teams and a lot of people raised their hand and wanted to be part of this program. We started to run it on a quarterly basis and WeWork was a global business and we flew around the world delivering a program, which we were calling data cult at the time, and then became kind of the foundation for our later companies and ways of thinking. But it was super hands- on and super in person as we then learned later that those experiences would change. It brought together people from all different parts of the company to think about data and it was empower, and I think it worked in many ways, but there were definitely limitations.
Tim Gasper: Bringing people together around the data oftentimes in person. This ended up being a big factor in being able to unlock business people kind of understanding more about their data, also owning more around their data too. Or did some other things have to happen to unlock that second piece?
Leah Weiss: Yeah, I think the premise was, so before I met Gabi... I give her a lot of credit for this. I've been a grumpy data person where you're just fielding a lot of requests. You sort of understand how the system works and the people on the business side don't, and you can start to, I don't know, you take some pride in how you're the only person who can do things even though that's like to your own detriment. What Gabi really taught me is how do you actually build that bridge? How do you make it feel like you're inviting the business into this process with you and building of a community around it as opposed to sort saying it's really complicated you wouldn't understand. That's kind of the foundation of everything we're trying to do. Yeah, that was the point of that program, that's limited in what you can accomplish in a short time. But the idea was like, let's bring you all into this process. Let's show you how data moves from our source applications to the tables that you might access to the tools that you're using to operate things and teach them how to think about leveraging data. But it was really that mindset shift. How do you put the pieces together as opposed to digging in your heels.
Juan Sequeda: Let's dive into this. What is that process? What did data cult do? Because what I really like is that you guys had this real world experience of trying to go do something from a cultural perspective, right? Not just, " Let's go through, let's go start with technologies." What you see all the time, you really start with the people in process and now you're getting to the tech. Yeah. You don't see this, right? It's always the other way around and this is why I'm super excited about talking to you all today. Talk us through that, the process of the process that you guys had.
Gabi Steele: It was a curriculum that we developed together that involved teaching SQL, teaching looker whatever, data visualization tools. As Leah's sharing, going through the process of the data engineer, bringing these source systems into a data warehouse and teaching people who didn't necessarily have any data background. We brought people into the room from sales and marketing and architecture and gave them, pulled back the curtain and shared everything that we possibly could about what it meant to get data into their hands. The idea was also to create ambassadors across the business. People on different teams who could reference their understanding of how things were accessed and how the data team was treating it, which was really successful in a lot of ways because you can get the word out very quickly by bringing 30 people from all over this massive company into one place to go through that program. Then the end was about developing a product that solved that problem. And it's also really powerful to say to people, okay, let's build something in a few days. It can be a prototype, maybe it'll spin off and become its own team, maybe not. That solves a really impactful problem for the business that's beyond their day to day and allowing people who don't usually get the opportunity to be so creative. Tying it back to numbers and data was super powerful. I think that was one of the best things that we were able to do. It's something that we've also tried to help other organizations recreate. WeWork at the time was also starting to buy businesses. They were buying Meetup and Flatiron School. We would actually help as part of the sort of M and A process is bringing them in and understanding WeWorks data, understanding their own data. There was a lot of kind of opportunities there. I'll let Leah continue with how that was going.
Leah Weiss: Yeah. That sort of became the foundation. It was totally a grassroots thing where we just saw a problem and ran with it. Then when WeWork went the way that it did and Gabi and I were starting the next, and we sort of assumed that we could build these sort of programs in other companies as well because we had some experience. What we learned actually is it's really powerful to do infrastructure work, data engineering work paired with that sort of change management and culture work. So that became the core offering of the consultancy we started after where we would go in, we'd build a modern data stack, but with the attitude of let's bring the business along for the journey. Let's sort make advocates out of our business stakeholders instead of tense people who tolerate us and maybe don't know what's going on. It was really finding ways to translate that philosophy into everything we do.
Tim Gasper: Interesting. You took this kind of curriculum that you tested and validated and made work at WeWork and then really looked to turn that into something that you could bring to other organizations and have both the business and the technology people riding along together on that. Overall, so what was your kind of assessment? Was that indeed something that you were able to do? Were you able to take that and copy paste that other places? Or did you have to refine the model? If so, how did you have to refine it?
Leah Weiss: Yeah, definitely not a copy paste. I think, well one interesting thing to note, we sort of assumed that in- person experiences would be a big part of what we delivered. We started this business at the beginning of 2020, so quickly realize that wasn't going to be the way forward. I think we also learned a lot about how people shop for data tools and for data help there really is a mindset where you're buying engineering hours and you might not identify that culture is actually the missing piece. It's something that we say that we have to slide in there. Yes, we'll build out your warehouse and we'll set up your reporting infrastructure, but let's do a few things along the way to make sure that this is useful. But let you expand on that Gabi.
Gabi Steele: Yeah, I mean I feel like the short answer of all of the questions, first of all know that it's not totally replicable but this problem of data driven culture, data driven thinking, achieving that at organizations is definitely of case at every company we've ever talked to. People are struggling with this whether you're at the earliest stages or the latest stages. I think we were able to solve for it in many ways and the delivery of what we did at Data Culture as Leah's describing, which then transitioned into a lot of infrastructure and engineering offerings through the lens of delivering these experiences as well or making sure that we're always empowering the people that we're working with. There was a few core philosophies, no black box systems, multidisciplinary teams, which came from this work as well and bringing together these ambassadors, it was successful. But the gap in terms of what we could do to solve this problem persisted, which led us to Preql, which is a product approach to the very, very same problem. This has really been the through line of our careers because the trouble with creating data driven culture or whatever you want to call it, and everybody says that they want to create this and that they've successfully done so a lot of the time is that one, it's hard from a technical standpoint so you can get bogged down there for a really long time and it's hard from the people standpoint. We've tried to go about it from both sides, but what we realized was what was taking us the longest when it came to the infrastructure implementation was the data modeling part of it was transforming that information so that it was in the format that business users could leverage. The process involved really strong technical expertise on the data engineering side and the business side. Preql is the product approach to that problem, and we can talk more about it, but what we did learn through the work at Data Culture, it's kind of like this gradual transition. It started out maybe we can just solve it with the culture and the training and the people and everyone will get involved and maybe not everyone needs to write, but if everybody understood where the data was, then it would be solved. That didn't quite work. Let's try it where we manually implement the infrastructure and train people on the job. Still an offering still exists. Go to Data Culture if you want that support. As we're building Preql, we're now thinking about a product that could fit in and really change the landscape in order to solve this. So it continues to be a problem but, and we're continuing to tackle it.
Juan Sequeda: I want to get into the things what Preql is, and I mean we are kind of honest, no BS, right? We want get into the salesy part, but I do acknowledge that what you guys are doing is different from any other tooling out there. Because most of the tooling when it comes to transforms and modeling more for the technical side and you guys are seeing that opportunity from the business side to go do that. I think people would be skeptical about it too. But I'd like to get into that. But before I get there, two things. One, you said first the data modeling, that is something that touches me a lot and this is a topic I want to get into. But before that, how did you identify the people who wanted to go part of this? I mean were they just always open, motivated by themselves or were you trying to, hey, you should participate about this. And so how did you identify the people in second? How did you identify what were the good problems to go work on?
Leah Weiss: Yeah, I think that identifying problems, that is of the key piece of it. I think this also will resonate from people who have been that data team of one or who have been bogged down in requests. Part of it is teaching people how to frame a question and produce a problem statement where data can actually help. I would actually say that the most impactful thing that a data team can do on the culture side is really work shopping problem statements. So people are coming to you with interesting things that spark your creativity where data can really play a role as opposed to asking for CSVs, which is sometimes where people get stuck. I think on the second point, a lot of those people, if you've been a centralized a team fielding a bunch of requests, there's some people who just want you to send the report by tomorrow. But there are some people who really want to be able to be more self sufficient who just can't, they don't understand the systems, they don't have the skills, but you can see that something in them is getting sparked and those are the people that you really want to empower. Instead of just delivering the thing as asked, use it as an opportunity to teach them what you're doing and maybe make them your advocate so then they can describe what the process looks like when their boss is asking for numbers, that kind of thing. Yeah, empowering those people who really want to do good work with data, just keep hitting these obstacles.
Tim Gasper: Interesting. The people that have that spark that you want to empower. What did that look like in practice, either from your experiences or what you kind of advocated? Was that targeting those people, especially as sort VIPs through the training and enablement process? Or some companies usually slightly more established companies try to establish data stewardship programs and things like that. Was it kind of like, " Oh you're kind of a steward." Or how did that kind of look?
Gabi Steele: Yeah.
Leah Weiss: Because we knew how the data team operated, who your repeat customers are and who makes the most noise and all of that, you develop relationships. We also had an application process I think managers nominate and the criteria is basically this. Who are the people who are taking initiative? Oftentimes data people can look at people who are creating their own manual reporting processes as the enemy of the centralized data work that you're trying to produce. I think there's actually something to be said. These are the people who really want answers to their problems and they're not being served. Instead of telling them to stop, maybe just teach them a different way.
Juan Sequeda: This reminds me a while ago we had an episode with Cindy Howson from ThoughtSpot and I think I still remember one of the takeaways is that shadow IT that we all kind of saying, " Oh that's bad. We need to eliminate the shadow. IT." Wait, there's initiative right there, right? Those folks who are doing that shadow IT, they're really trying to get a problem solved and working on it. If they're putting that effort, I mean there's something so actually go find that shadow IT and empower them. Let's get that. It kind of seems like what you're doing is an approach is go find that shadow IT and saying, " You know what, let's actually make this visible actually help you to be successful."
Leah Weiss: Yes.
Gabi Steele: Yeah, totally. No, I mean we're just agreeing on that exactly and that we can go into the psychology of what people want to do at work and how they want to contribute but what we found was when given the opportunity, everybody was raising their hands and Leah's also describing there was eventually an application process but these lists were just endless. Some of it is the stewardship ambassador type thing and the other is when it comes to data and understanding that data is just information, everybody's job can be done better when armed with more information. It felt like something, I mean everybody was interested in jumping in on and eventually had to be a little bit more kind of nomination and who could really take that on. But yeah, go ahead.
Juan Sequeda: Let's get into the topic of data modeling. This is always, actually put it on LinkedIn this morning. Data modeling is an art as it is and which is a science here. I think data modeling is about being able to understand the particular domain of the end user, the subject matter experts and you need to go formalize all this stuff. What is this process of actually getting the data modeling done by those business users or by those end users and what are the types of technical kind of background that they may be lacking and how did you upskill that? Very curious about this.
Leah Weiss: Yeah, I think data modeling is maybe the most underrated skill in all of data. I don't think it's taught specifically enough. It's sort of just seen as a thing that you have a knack for or you don't, or a byproduct of other roles that you've had but it's so fundamental. I think what makes it really hard is you need to have people who are technically really, really strong in terms of actually writing the transformation in SQL or Python and whatever it is. But you also need to have a brain for architecture how to do that efficiently. Then you also need to anticipate not just the question that the business is asking you today but what they're likely to ask you for the next few months.
Juan Sequeda: Bingo.
Leah Weiss: That's just tough to find in one person. I don't see it being sort of tackled head on. I think DBT obviously has given rise to the analytics engineer, which is a really specific set of skills but often it's sort of the surface level skills that people are training on. But this big picture, how do you go from the domain and the high level questions that people are asking to an efficient transformation process and data model. It's just very hard to get right.
Gabi Steele: That's just for people that actually appreciate and understand that this is a problem. What we saw also time and time again is businesses being confused by their numbers don't match and just kind of missing that this piece is such a requirement when it comes to having a central source of truth. Everyone wants to be data driven, right? But this is an overlooked problem and especially from the CSU and a lot of our partners who were bringing in the support for data, they would be like, " Well, why do we need to spend six to 10 months setting this up and why do I need to pay someone this much money for evermore and this specific skill set and all of these things?" Getting someone to really comprehend what the consequences are difficult but that goes back to the business side of things, which is also why we felt there were such a gap in the market when it came to tooling that supported the people that would then be the consumers of the models and the information.
Juan Sequeda: Yeah, I just want to say that what you just said was so spot on Leah about you need to be able to go and answer the questions of today but also be able to prepare to answer the questions of tomorrow, which you don't know what those are. This is what I always talk about, this balance between being efficient and being resilient. I mean we're all so focused to be efficient. Let me get this done now. You're like, " Don't even think about how can I make sure this can scale tomorrow?" Not even scale in the size of the data but just scale in like, " I want to be able to go answer more questions with least amount of work that I'm doing." Right? I think is we just focus so much on being efficient. " Give me now, the numbers now." I'm like, " Wait, let me step back and probably do a little extra work right now but it's going to let me go answer a bunch of questions prepared for things. The knowns of today and the unknowns of tomorrow." I'm really glad you're bringing this up.
Leah Weiss: I think it's even more so when these skills get increasingly technical because answering the questions today, even though it can be a fire drill and it's not always fun, there're interesting problems to be solved and you have to be creative. I feel like sometimes really strong talent gets caught up in solving those problems and loses sight of... You're anticipating technical challenges and you're sort of knocking them off as you encounter them but that becomes the focus versus what is the business actually trying to get out of this and what are they likely to come to you next with that? I think that's a rarer skill.
Tim Gasper: Yeah. You said you have to be creative. What other skills does a good data modeler need to have?
Gabi Steele: Yeah, well you need to have the people skills to partner with the business side, right? Because there are going to be limitations to what anyway. They're the people that truly understand how they want metrics defined, whether or not they understand the process. To find somebody who technically is super skilled and super up to date with the best technologies they understand DBT. There's a lot of people that write DBT, that say that they can, few do it well and the technical debt of doing it poorly is so significant. We have a colleague Lauren Baylek who speaks quite frequently on this and we saw that also as a really common thing when we were doing the work with Data Culture and going in and supporting engineering teams. This combination of skill sets which is truly understanding at least the business user if not the entire business because that as Leah's describing, it's super difficult and then being highly skilled on the analytics engineering technical side which is evolving obviously and is kind of its own unsolved problem, is what we look for and being a strong collaborator as well. Leah, I'm curious if you want to add.
Leah Weiss: No, I'm just thinking. I think there is sort of a certain personality type that gravitates towards this role sometimes because there's so little glory in it. You're always blamed and the end user probably doesn't understand that if it was successful it was because of all of this work that sort happens under the surface. You kind of need a purist who just loves the work for the sake of the work even though they might never get the pat on the back.
Tim Gasper: Yeah, it's almost worse than data engineering because I know sometimes data engineering they have to do the plumbing but at least you can see the plumbing, you're like, " Oh I see the pipe." Whereas a lot of the modeling work is brain plumbing, you don't even see it.
Leah Weiss: Yes, yes. There's always a problem, you're never going to get it right on the first try.
Tim Gasper: Yeah, your comment about DBT is interesting as well around if you're doing DBT you have to actually be good at and kind of good at DBT. It seems like with some of the new tools, even though you can do very interesting modeling, you can implement your semantic layer if that's kind of the direction you want to go, right? Yet it's also easy to not do it well and just make spaghetti code and throw things at the wall.
Gabi Steele: Exactly. I think that was also fundamentally important to us when trying to create this product. We've been in the process of building pretty heads down with that for a while that didn't require consultants to implement because there's a lot of tools out there that are just completely they non opinionated, agnostic developer tools that take many months to learn and master and then once you've done so you still need so much input for them to be put in place, segment's a really good example actually of complicated developer forward, and then once it's in place you still need opinions for the business of how you want to be collecting information that sort of way. What we've been trying to do with Preql is something that you can get stood up immediately and the time to value is just so much faster than a lot of what's out there, but again it's a different audience. Back to DBT, which has completely changed the game. I think the analytics engineering field was in some ways created by them, which we're speaking about and we love it and it supported every project that was ever put in place by Data Culture and we consider it a future partnership. It's just being realistic about the amount of time that's required to actually take on the learnings of the yammel files and how you set it up properly and how people can make changes down the line and not just having, Because if you don't have somebody who can recognize the difference between spaghetti code and truly what it should look like, it's very hard for a lot of organizations and as they're going through that growth phase between being more of an early stage startup. We really thought it was series B, a lot of the time where companies then grew into needing more robust infrastructure and more robust like analytics engineering specific teams.
Tim Gasper: Then the need for building out a true data team and a well thought through data infrastructure becomes really important at that point. Yeah, this is interesting and I want to quickly, before we move to the next topic here and actually kind of bring it full circle to where we all started around the business needs and putting the business in charge of data. A quick call out. This episode is brought to you by data. world, the data catalog for the data mesh. A whole new paradigm for data empowerment, learn more at data. world. To bring it back full circle, we were asking about how do you put the business in charge of their own data? I think we've talked about data literacy, data modeling around Data Culture, the right data infrastructure. Ultimately, as you went through this journey with data cult and the Data Culture, you still found the need to build out this tooling a solution that could actually help solve this problem more from a technology aspect married to the other pieces. Can you talk through a little bit the story of specifically how that problem came to before? What really accentuated it for you, were the business team had this big issue? Then can you tell us also a little bit more about Preql? How is that trying to solve the problem?
Leah Weiss: Yeah, I can sort walk through how we got here and then maybe Gabi can tell us about where we are now. We were doing all of this work setting up the modern data stack for companies that were generally in their earlier days of building their data stack. You set up Snowflake, Fivetran, all your DBT models, you set up reporting and you go through this process and we try to infuse it with as much sort of collaboration and Data Culture as possible. Our team is amazing and our analytics engineers were amazing, but at the end of the day we would be working with a business stakeholder. When we're done with our work, we're handing back a GitHub repo that handles so much core business logic that they won't be able to maintain themselves. From a consulting perspective, kind of a good business because they need you on an ongoing basis and there's lots of work to be done and we could manage it indefinitely, but it just of gave us this feeling like we're not actually really delivering the value that we want to, we're not actually empowering this person because even if you want to make one tiny modification to a definition or update business logic or just update a case when, they need to find this specialized skill set engineering to make that change and then see the change of propagate through their reporting. There's just something a bit unsatisfying about that and we became kind of obsessed with it and we were researching and just waiting for tools to come onto the market that we could offer people in that position. Then unfortunately realized it's a big one but we were going to have to build it ourselves.
Gabi Steele: Yeah. We waited for a while and we were really ready to sell it. We kept thinking if only this tool was out there, it would be the solution to us leaving things behind and feeling better about the projects that we were wrapping up and we couldn't exactly build it through Data Culture without having a dedicated engineering team for it. One thing led to another and we started to talk more about the problem and we ended up spinning off Preql. But even just looking at our engineering hours when we were trying, you're with the service business, you're always looking at where is the time going? Where is everything adding up? It was the largest amount of time spent always was this process and it was the trickiest thing to do. If we didn't have a good partner on the business side it would take even longer. Back to that time to value was the reason that we set things in motion. Then speaking about where we are today, I'm here in our offices in Union Square. We officially started things around March, did a transition around that time from Data Culture to Preql. We have an amazing team of almost 10. We've got some great designers and engineers working on the problem today and we have an MVP that is being tested by beta users and we plan to go big and launch things early in the new year. So specifically right now focused on B to C companies because we're developing things from a verticalized approach but it should really help all organizations, ultimately. Yeah.
Juan Sequeda: I'm really glad that you're sharing what you guys are doing right now. As I mentioned before, I think it's this shift of what we're not seeing, right? Today, a lot of the focus is more on the technical side. It seems like this is the way you're describing the lack of the tool of the tech is you want to have almost like a BI tool- ish that lets the end users do what the look of mill would do beforehand, but more from the end user business user perspective in a kind of low code, no code manner.
Leah Weiss: Yeah. When we talk to data people sometimes we describe it as kind of a no code semantic layer. Because the process that we're trying to enable is having the business user who really knows their domain, they know the metrics, they even know weirdness is about the data because they've been doing it manually today, they're not empowered to create and manage those metrics. You sort of take those as requirements and pass them off to a data team and that back and forth can be really tense and sometimes inefficient as we've been talking about. The idea is to empower that person where they can construct metrics and we abstract away the process any sort of underlying transformation that's required and then it's kind of the management of that semantic layer in a no code way.
Juan Sequeda: All right, let me play devil's advocate and honest no BS here. What if we would just establish the teams where you would actually bring in more of that technical, the analytics engineer or whatever, right? That would be part of a business team and then there would be, I don't know, close to the hip right there. Then, " Yes, I need to ask you to do this transformation code, but you're literally right there and we work side by side." That seems to be a possible competitor to the type of tools that you're presenting here. What's your perspective on that?
Leah Weiss: Yeah. I think the question is, does every organization need to invest in an analytics engineering organization? Does that make sense? If you're a retail company managing of standard metrics and you're not necessarily a technology company, the idea that you need to go to the CTO and say, " First we need to hire data engineers, then we need to hire analytics engineers, then analysts, then BI developers." I don't know that, that's the right path for everyone. I would also say analytics engineers, don't necessarily love the job that you're describing. I've done a lot of that where you're just sort fielding requests and making these small modifications to business logic. There are really fun, interesting technical problems to be solved in analytics engineering. But this sort of short order cook thing we've heard it described as for the business can be a painful one. We often find the most talented people in our networks are the ones that are running towards data engineering or platform engineering or anything that gets them out of the line of fire.
Juan Sequeda: Yeah, no I get that. My point is, really if you want to be successful, at least the point I've been making lately is, if you want to be successful in your career, align with the business that figure out where the money's being made, understand that. I mean I'm not saying you won't be successful in your career if you just don't do the business and focuses on the tech side. If you want to get a full picture and just have a lot of career growth, I mean, I feel like understand how businesses make the money and what's the flow. I mean, follow the money. I it, I do perceive that. I wish we had more companies or more teams would want to go partner with the business, like I'm saying partner with the hip and stuff. I get that just the culture of oh we're just the engineers and we go on our side and stuff like that. Yeah. I think mean different cultures again. Again, it's not technology, it's just the cultures that you're trying to build.
Leah Weiss: Totally. We're data people first and foremost. We would never want to build something that a data person wouldn't be ecstatic to inherit. We've all sort of gone into a company and been like, what did the business buy and how do I rip it out and how do I move all of this hidden logic into something that I understand? The idea is basic reporting just shouldn't be this hard. It shouldn't require a team of exceptionally talented people in state of the art technology to understand the performance of your business. That's kind of the idea. That's a starting point, it's just people never sort of cross that hurdle. But anyways, the way that we envision it is we've done that annoying part for you where metrics are standardized, you have a source of truth now you can go build upon it, do more sophisticated analysis, but there's so much value loss because people don't get to the other side of this never ending back and forth between the business.
Juan Sequeda: If we think about it as the always talk, I call the data knowledge gap. On one side you have the tech side, on the other side you have the business side, right? On the tech side, you have where the data engineers, right? On the business side you have your analysts, the people are connected to the business, you need to bridge this gap. I think, I'm seeing three ways of doing it. One the gap is being bridged more from the technical side. The data engineers aren't doing that, but that means that they have to understand the business and we're actually making the case that, that's probably not really happening. The other part is that oh you create the new roles of who would somebody would go into that middle gap and go into the gap and this is probably the analytics engineer trying to fill that as a gap or the data product managers are also too. The third approach is just saying, " No, the way we're going to bridge the gap is from the business. The business is going to take ownership of that gap and bridge that gap." I mean that's how I'm kind of balling this framework. Your position is, yeah, the business is the one who is going to bridge that gap. Is that a fair assessment?
Gabi Steele: Yeah. I mean I think first of all, yes, our idea here is in order for the gap to be closed, the business user needs to be empowered. That's not to say that analytics engineering shouldn't exist or that engineering and tech teams. I think that's the same argument that you're making, which is to have everybody have more of a view, will benefit the bottom line, the revenue, why we're all doing this anyway and it'll be more interesting work, but it's not that one job is obsolete and the other is more important. I think that's what also we talk a lot about no code and whether Preqls a no code or not whatever, but that movement towards tools that solve the most kind of uninteresting as Leah's describing, parts of the problem. Bring everyone closer to the business because then their time can be spent thinking about why they're actually doing the work versus just the latest technology that will make this thing faster or that dev tool or whatever it is. Yeah, and I think that we're so aligned in terms of... Leah, we talk about this a lot, why we're so interested in data and why we are data people is it gives you a lens into the business. It's so interesting to look at different companies' data and that's fueled a lot of our work as well. How do we share that?
Leah Weiss: Yeah, well just because we've been working on this problem for so long and in so many different capacities, I think it would be silly to say that there's a silver bullet for this. I think everyone needs to work on how they can bridge the cap and I think it technical people moving towards the business a hundred percent, that's the advice I would give a thousand times over. But I think maybe there are workflows that can be improved and of translation layers that can be improved just to ease the most fraught parts of this process. But every business is always going to be about people and process and communication and no software is going to change that.
Tim Gasper: Just before we move on to our lightning around and some of the next steps here, I really want to hone in on what I'm thinking I'm seeing as some of the value here of something at this layer that you're talking about. Gabi and Leah, let me know if I'm getting it wrong here but I think about recently I saw that post from DBT where they were saying, Hey, this is what DBT looks like for DBT and it was kind of showing off their own use of it internally. I think that they showed off that they had 700 models or something like that and I was like, " Holy cow. Is this not good or bad? I think it might be bad, right?" I'm wondering if one of the values that you're looking at here, to your point around streamlining workflows, is it like, " Hey, if you're committed to the semantic layer, great." What's going to happen is things are going to sprawl, things are going to get complicated. Ultimately the business user's always going to be asking the analytics engineer for help or whatever, right? To model that metric just a little differently and now there's model 701. Is the goal kind of here, let's really minimize the sprawl, empower the business user. Is that kind of another angle at looking at this or do I have that a little bit wrong?
Leah Weiss: Yeah, I think that's right. I think sprawl happens when you don't have alignment from the beginning on a few core things and then everyone comes up with their own approach and then you've got many different implementations of overlapping things or that, that's one thing that contributes at least. I think, there's so much analysis you can do and there's many different ways you might want to structure your data in a high growth org, but there are some things that I think we should be able to figure out and that's aligning on performance metrics, knowing how they're defined, knowing how to customize them, knowing how to integrate new data sources without breaking all of your reporting, that kind of thing. I feel like that gives you a way to tackle the hard stuff because what I've observed is every time you think you've gotten through the data trust issue and the basic reporting issue, you find that you have to return to it because somewhere that cycle got broken. The idea is maybe we can ease that part of the process and be more deliberate in what we build after that.
Juan Sequeda: Now if you look at the landscape, which is so crazy, how many different tens of tools, right? 40, 50, 60 tools, right? You see all these companies or these transform tools right now, right? Now, the metrics layer and all these things. What I'm perceiving is you really want to have the type of a transform metrics semantic tool altogether, but whose audience is going to be more on the business side, and that's your approach over this?
Leah Weiss: Yeah, I don't think business users care one way or the other semantic layer what you use for ETL or ELT, what they care about is, can they consume metrics that they trust? I think our hypothesis here is that they'll be able to consume metrics that they trust if they're empowered to own business logic in a way that they can't today because they have to translate it to this other audience.
Gabi Steele: Yeah. I think just one more thing on what you were saying Tim, about all the models, it's like you're referencing what metrics matter and that was also a big part of our thinking and our work around the definitions and data models. People tend to care about the same metrics. Sometimes metrics that seem really important are actually the worst things to be looking at and maybe that's one of them, but some sort of standardization there would save a lot of people a lot of time and that kind of came from this work as well. How do we direct people and get them thinking about, get analytics engineers working on the problems they really care about, get the business people thinking about the problems that are most pressing and can have a real impact and then putting that power in their hands.
Juan Sequeda: Yeah, time flies. I have so much stuff I want to dig into this, we need to go to our lightning round site, but I think there's still conversation to had to understand. Where is the work going to go between the data engineer, the analytics engineer, like the middle of the gap called analytics, whatever, and the business side, I think. But as you said, there's not a silver bullets like, " Here's the pattern to go do it." It's going to depend on the size of the company, the industry, the culture and so forth. I think it's a fascinating discussion and there's no right or wrong answer here. With that, I told you we've been talking for 50 minutes, we can keep talking for another hour. At least you're easy. All right, let's move to our lightning round, which is presented by data. world, a data catalog for your successful cloud migration. I'm going to kick it off. Question number one, is the rise of the analytics engineer helping the goal of good data modeling or is it actually kind of harming it?
Gabi Steele: What a question.
Juan Sequeda: Honest no BS.
Leah Weiss: I'm going to say both.
Gabi Steele: Yeah. Okay. Is that fine?
Tim Gasper: Leah, go ahead.
Gabi Steele: Go ahead. Leah, says both. Leah says both.
Leah Weiss: I would say both. It's like it's great that it's more accessible and I think over time that will sort of standardize and create best practices, all of that. But right now, I think it's a bit of a mess.
Gabi Steele: Yeah. I was going to say yes, because even though right now it's a bit of a mess, it's curving, it is shedding light on the problem and that takes us in the direction where we can actually solve it. Even though ultimately right now might be a no, it is at least bringing attention to this problem and I have a big issue with this being an overlooked problem. But yeah, I think.
Tim Gasper: Yeah. I like that perspective. It's like we're shedding light on it and as part of that, maybe it has to get a little worse before it gets better.
Gabi Steele: Exactly.
Tim Gasper: Interesting. Second question, can technology eventually... It's pretty open- ended here, can technology eventually solve the Data Culture problem?
Gabi Steele: Cool. Well we're kind of banking on it right now, but I think technology needs to improve in order to solve this problem. But our work has always been about thinking beyond that. Even with our product, it's not just going to be the product that solves the problem, it's going to be everybody that works on our team and touches the product. I kind of want to say no, but go ahead, Lee.
Leah Weiss: Yeah, I think if technology can be really focused on helping people achieve really specific things, this is like a human problem that we're tackling, which is why it's so tricky. The idea is just to make that process better and make it more efficient. But you still need the minds to really think about how they want to measure things. I don't know. I'm being, I'm saying yes and no to all of these things but...
Tim Gasper: Great response. I don't know if there's a good answer to that, right? It's a piece of the puzzle.
Leah Weiss: Yeah.
Juan Sequeda: Next question. Will we get to the point in the next, let's say five, 10 years where the business users, the analytics end users, they become the people who actually build the data modeling in that semantic layer?
Gabi Steele: Yes, 100%. I believe that. I think that whether the business user is a data analyst and someone with some data experience, I think the modern data stack is moving to a place that anyone could set it up. I think it's already going close to that direction. That's not to say engineers won't exist, it's just can you set up a full data infrastructure without requiring extensive knowledge on any given technology? I really hope so.
Tim Gasper: Yeah.
Juan Sequeda: I'm with you. I hope so. Now will we get there, huh?
Tim Gasper: Yeah, Leah, anything to add there?
Juan Sequeda: We better get there.
Gabi Steele: Yeah, I'm like, " We better." Exactly.
Leah Weiss: Yes. I think these things, they move closer to the business. That's sort of been, that's how data engineering has gone. I think that's how analytics engineering will go as well.
Tim Gasper: Interesting. Cool. Yeah, it sounds like the flip side of that question, is data engineering going away? And the answer would be no, it's not going away. All right, last question here. We talked about the semantic layer towards the end of our conversation today, and y'all are kind of, I think in this space, or at least dancing around it, is business intelligence, is BI, the killer app of the semantic layer?
Leah Weiss: The killer app meaning the thing that kills it or the thing that's killer?
Tim Gasper: The thing that's killing.
Leah Weiss: I'm very excited to see how BI starts integrating with the semantic layer. I think what we talk about a lot is Tableau was built for a totally different data infrastructure and most BI tools are about manipulating columns in row. And I think a new generation of really interesting tools will be built on the semantic layer or the semantic layer won't work for the reason that it's not compatible enough with BI.
Gabi Steele: Yeah, I think that...
Tim Gasper: Gabi, anything to add there?
Gabi Steele: Yeah. I spent so much of my career talking about data visualization and different ways that you can build a chart that will solve a problem. I think a lot of the work that has led Leah and I to this moment of Preql has come from dissatisfaction on the BI side and we didn't want to go out there and start building the next look, or we felt like that wasn't the right way to start tackling the problem. Semantic layer is the first step, and I'm under the belief that a new type of BI solution will come about and the semantic layer plus that is going to solve the problem versus being killed by a crappy BI tool, which is any BI tools are very limited.
Tim Gasper: Yeah, I love the way that you're...
Gabi Steele: Hopefully, we can go beyond.
Tim Gasper: I love the way you're thinking about this, and I implemented Looker at my previous job and I've always been very fascinated and also slightly terrified of the look in our layer and been like, " Wow, this whole semantic layer is great. I mean, they really pioneered it." But then I was like, " Man, you got to learn this whole new language and stuff like that?" I love where this is all going and certainly think that the world of BI is going to change here. I'm excited that you all are trying to help it change.
Juan Sequeda: Yeah, I'm with you on this. This is my freaking PhD over a decade ago was exactly on building these semantic layers. I better freaking work. I kind of wasted my part of a big chunk of my career. Yeah. All right. Well, no, we're totally in line on the business needs to be involved and let's get the semantics, the knowledge in here. I really appreciate this conversation with that, Tim, take us away with takeaways. You go first.
Tim Gasper: All right. This has been an amazing conversation. Time truly flies. We started off by kind of saying that there's this huge job to translate for the company if you have knowledge about the data as well as the problems that need to be solved and connecting that all together and that it's a really huge job. Then at WeWork, you all had the opportunity to try to bridge that gap in a variety of different ways. One of the foremost things was really developing this curriculum and this training approach to being able to try to solve that gap. Onboarding people, helping train them on data tools, on Data Culture. Then it was so successful at WeWork that you really took that methodology, that curriculum to other companies. This is all around this whole Data Culture process, right? You have to learn modern tooling, implement a modern approach to data, find and create ambassadors in the business people that you can really leverage as your centers of knowledge, your reference, really evangelize, get the word out, bring in people from across the business, try to iterate quickly, build something in a few days. Let people who don't normally have the opportunity to be so creative, get to be creative with data. I think there was a ton of really good thoughts around and approaches around how to build that Data Culture and bridge that gap between the business as well as the data folks. I think interestingly, you talked also a little bit about the challenges of implementing that at various companies. For example, you thought that in person training was going to be very important and unfortunately the pandemic happened. It's like, " Well, it's going to be remote first." Right? Also companies kind of falling into this trap of being infatuated with the engineering piece and not as much the culture piece. It's like, " Well, how about we just have the data engineering and we don't really need the Data Culture piece." It's like, " Whoa, our company's named after it." I'm like, " Hey, how about we just include it in some of these other packages?" I thought it was interesting to hear about some of the story there. And ultimately as you went through this journey, more from the services and consulting side, it kind of led to Preql, which I think Juan will go into here in a second. Juan, I'll pass it to you. What were your takeaways?
Juan Sequeda: Yeah, so a couple here. What can the data team do to impact the Data Culture? I think you talked about being able to get the people together and produce problem statements. I really like this go workshop problem statements because it's sometimes you think it's kind of so obvious, but let's get people together saying, is this truly the problem that we're trying to go solve? Are you able to go write it down? I really like that. People that really want to go solve that problem, go identify those who are the people are that spark. You want to go empower them. Who are those people who are repeat customers of data? Those are the folks that you want to be able to go bring them in so they can impact that Data Culture. " Hey, the managers will know, right?" Their the ones who are going to nominate. They're the ones who know, who are the people are taking initiative. We brought up find that shadow IT, empower them. Those are the people you want to be able to go bring in to impact your Data Culture. We talked about data modeling and how that's the hardest part. You said data modeling, it's most one of the most underrated skills in data. It's not taught enough. What are the kind of other skills you have to be creative, but what other skills do we need? People skills, not just the tech skills. The kind of person who sees a problem asked once and thinks, " Hey, I think this is a problem that is going to be asked again and again, we should optimize for this." Right? What are those questions that you need to answer today? You can also figure out what are those questions that can probably will be asked tomorrow that you may not know so how are we going to balance those things? Somebody, you mentioned about, " Hey, if you're doing DBT, you actually have to be really good at doing DBT because otherwise you're going to be generated as big sprawl things." Then kind of closing up to the tools for the business, which is to kind of close entire circle here is ideal tools for the business is that you don't need consultants to go implement them. One of the big problems right now is that if you like me to change any type of definition, you need to go have those technical folks who have specialized skill sets to go do that and you spend so much time on those transforms. The ideal scenarios, because what you guys are envisioning here is, like a no code semantic layer or the business users really know the domain and they're empowered to go build and manage their own metrics. I think you said something really great and it's on the 45- minute mark. Technical people need to get into the business. That's the advice you would give a thousand times today, you said that. We really need to be able to go minimize that sprawl. At the end of the day, business users, they want to be able to consume metrics that they can trust and they can if they own their own business logic. How did we do for takeaways? Anything we missed?
Leah Weiss: I think you nailed it.
Gabi Steele: That's right.
Leah Weiss: Yes. Yeah.
Juan Sequeda: All right. Well we'll throw it back to three questions to both of you. What's your advice about data, about life? Who should we invite next and what resources do you follow?
Leah Weiss: Okay, Gabi, you think about who to invite? My advice...
Gabi Steele: Okay, I got it.
Leah Weiss: My advice, we teach a lot of young people when we have the opportunity, which is really the favorite thing that we've been able to do in our careers. But my advice is always just follow your curiosity. These technical skills are ways of being better at whatever you want to do, but it's often a means of solving a problem. It's not sort of the goal in and of itself. Any problem that's exciting to you, learn the skills, you'll be motivated to learn the skills that you need in order to solve them.
Gabi Steele: Yeah, no. Totally echo that, and just gives me more ideas of people we should invite. Some of our young scholars who did art data science would be amazing. Yeah, bringing more voices into the space of data science and engineering has been super important to us from the beginning and felt like a responsibility in being a part of this world. Yeah, we have always been telling... And the fact that data touches everything. There are so many ways that you can bring your personal passions into the work. We've also started companies for the purpose of letting people bring their full selves to work and that sort of thing. Even though we're working in data, we're trying to do a little bit more than that and make more of an impact. What was the next question?
Leah Weiss: Who they should have on.
Gabi Steele: Okay, cool. I'll give who they should have on. Then you think of resources because you're the big greeter of the two of us. First person I thought of was an amazing woman called Rhys Berkwitt, who leads data strategy at Data Culture. She was previously at Segment, has a really interesting perspective on the problem and works with a lot of our clients. She works closely with Neil Oliver, who you should also bring on. The two of them are an amazing dream team group. Then there's a young person, Anam Ahmed, who would be delightful on this podcast. She is going on to study data science at Barnard College and is a Kode With Klossy alumnus that we know very well.
Leah Weiss: But Gabi, some amazing Data Viz people, who should they bring on from that world?
Gabi Steele: You could... I mean, I always reference Giorgia Lupi. I think she's a really interesting kind of person of the time. A little bit different from the two that I brought, just thought of. The data visualization, well, Marisa Ruiz Asari is also an amazing person from the database community. I graduated from Parsons' state of this program, so there's a ton of people from that program. I can send you lists and lists of Data Viz people from there. If you can get Georgia to come on, she's got a really interesting take on smaller data sets and day to day data. She's done a lot of work around her life in a day and kind of drawing that out. I think when we talk about BI tools and the future, it's got to be a blend of some of that more innovative data is, and the stuff that we're seeing come out of Looker, which I think is now being sunset by Google. But anyway, Leah, resources.
Leah Weiss: Resources. I don't actually know how I get the information that I get, but I read everything. I guess being in all of the slacks.
Gabi Steele: Wow.
Leah Weiss: Sorry. I realize the day has changed so now I have no light on my face. Sorry. The DBT Slack. Locally, Optimistic, any Slack channel where data people gather. We actually have employees in Austin now, and we've heard that there's an amazing data community there. Yeah, and I don't know, data Twitter is a lot for me, but there is good stuff there.
Gabi Steele: Stay away from data Twitter, it's just whew. No, I mean it's interesting but it's a lot. No, but the point of that is self people just posting things is actually one of the best ways. Not necessarily turning to a single voice in a book or whatever it is, I think has been really interesting for us. Then those live updates, Data Culture has its own Slack channel as well, so that's a good place to go. Not that this is a salesy thing.
Juan Sequeda: So much information this last couple of minutes.
Tim Gasper: I know, I'm seriously typing notes here.
Gabi Steele: Oh yeah, you can get us going. Yeah, yeah, yeah. We're going to invite you back on our podcast next time so this is just...
Juan Sequeda: Love it. Love it. All right.
Gabi Steele: ....
Tim Gasper: Awesome.
Juan Sequeda: This is awesome. I mean, we have had an amazing conversation. We've passed our mark, so this is always a great sign. Quickly, next week we have Dan Bennett, who is the Chief Data Officer of S& P Global Commodity Insights. We'll be talking about the need for more semantics, especially in all the database vendors. That's going to be a fun conversation next week. Also information, we're going to be live, doing a live broadcast of our podcast at DGIQ, the Data Governance and Information Quality Conference in Washington DC. Live Wednesday, December 7th at 4:00 PM Central. We're doing that live at the conference. If you're going to be in DC let us know. Reach out to us because we're going to organize a happy hour after podcast event and stuff, so let us know. With that, Gabi, Leah, thank you so much. This was an awesome conversation. As always, thanks to data.world, that lets us do this every week. Cheers. Have a great one.
Tim Gasper: Cheers, Gabi. Cheers, Leah.
Gabi Steele: Cheers, Tim. Cheers, Juan.
Juan Sequeda: Draw your beer. How do you do now?
Speaker 1: This is Catalog& Cocktails. A special thanks to data. world for supporting the show. Carly Berghoff for producing. John Williams and Brian Jacob for the show music. Thank you to the entire Catalog& Cocktails fan base. Don't forget to subscribe, rate and review, wherever you listen your podcast.
DESCRIPTION
Data and business teams become a convoluted intersection, and when they struggle to communicate, it leads to bigger problems than awkward water-cooler talk.
So what comes first? Translation? Data literacy? Company culture? The chicken? The egg?
Co-founders of Preql, Gabi Steele and Leah Weiss, join hosts Tim and Juan to discuss how to put the business in charge of their own data and how this leads to the answers AND massive alignment between data and biz teams.
Today's Host
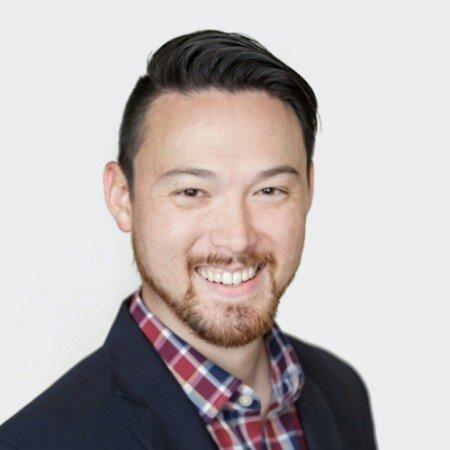
Tim Gasper
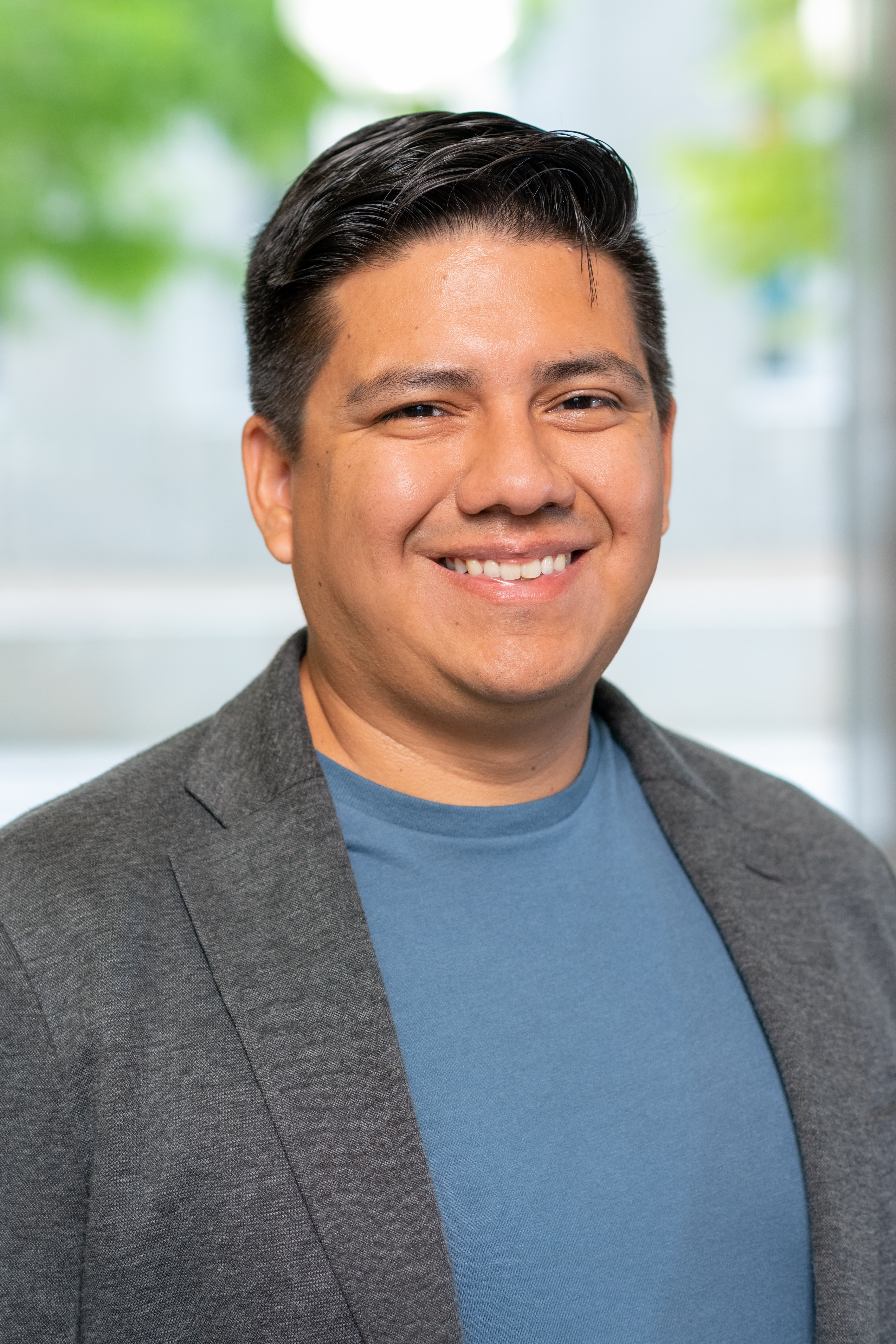
Juan Sequeda
Today's Guests
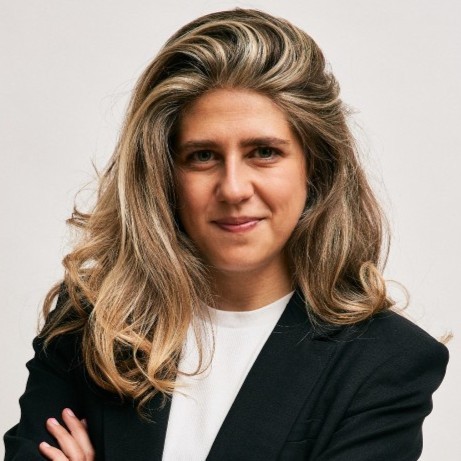
Gabi Steele
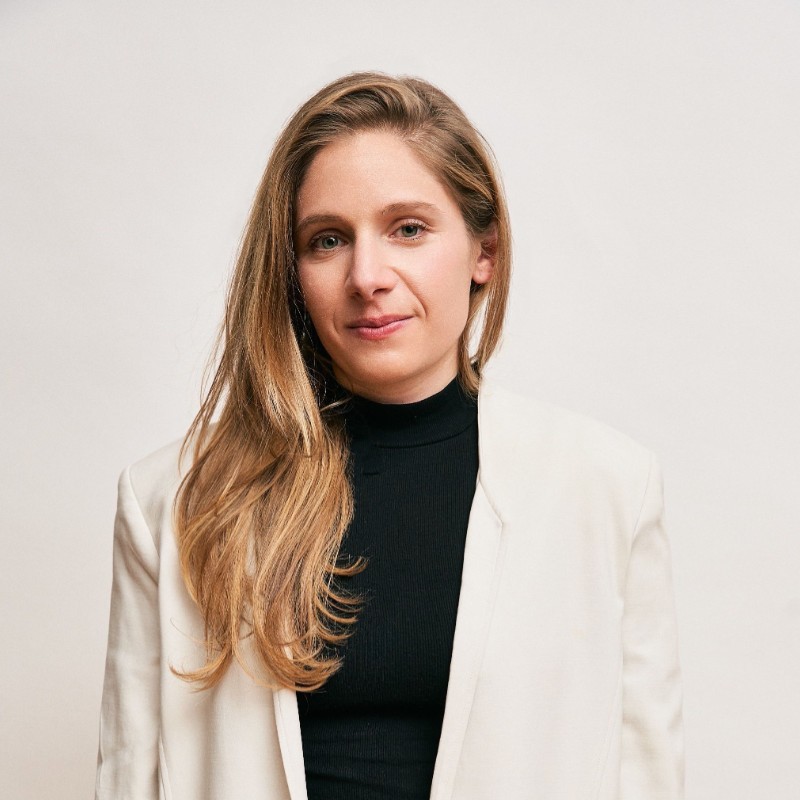