Modeling and Semantics are more important than ever, because of AI with Joe Reis
- 0.5
- 1
- 1.25
- 1.5
- 1.75
- 2
Tim: Time to kick off season 6 of Catalog& Cocktails presented by data. world. It's your honest, no- BS, non- salesy conversation about enterprise data management with tasty beverages in hand. I'm Tim Gasper, product guy, customer guy at data. world, joined by Juan Sequeda.
Juan: Hello. Hello. I'm Juan Sequeda, principal scientist at data. world, and we are back season 6, year four.
Tim: I can't believe-
Joe Reis: Oh bang. Congratulations.
Tim: Thank you.
Juan: And-
Joe Reis: For condolences, I'm not sure which, but...
Juan: This is exactly we're like, Tim and I was like, " Can't believe we're doing this. It's again, I can't believe we're doing this again. And I don't know." But...
Joe Reis: Let me ask you, well, from when you started the podcast to today, is the mission still the same? Was you guys still having fun turning the podcast back on to you guys? But I'm very curious. It's a long time for the podcast.
Tim: Yeah.
Juan: So but hold on. Who is this person who's talking to us right now?
Joe Reis: Oh, I'm Joe Reis. How you doing?
Juan: How are you doing, Joe?
Tim: Welcome, Joe.
Juan: Joe, this is the first time we have a repeat guest and as I wrote on LinkedIn, the three of us we just bumped into each other in Snowflake and we were having beers and we were like, " This is a good conversation, let's just do this on the podcast." And I think Tim and I were honestly like, " When are we going to start the podcast again?" I don't know. I'm like, " Maybe we should just do this with Joe. We'll kick it off." And I think we were going to do it last week but you couldn't do it. So then we decided to do it this week. That's how it goes. That's the honest, no- BS about it.
Joe Reis: No, it goes. It works. It works.
Juan: So back to your question, we just like doing it. We've never... What has been our mission, Tim? I don't know.
Tim: Well, we wanted an excuse to drink at four o'clock central on Wednesdays. But then secondly, and most importantly, it's just been about learning. Right? I think we've been wanting to meet people, have interesting conversations and really we've always been thinking, man, the best conversations are the ones that happen on the side of the stage, not the ones that are happening on the stage. And so that's kind of that mission continues and we get to have great conversations with folks like yourself, Joe, and talking about what's really going on in data.
Joe Reis: Well, I'm happy and sad for you.
Juan: Well, here we go. Well, so let's kick it off. What are we drinking? What are we toasting for, Joe?
Joe Reis: I'll pull to your success, obviously, as a four- year show. I mean, I think that's what we should be drinking to. That's pretty awesome.
Juan: But you've been doing this also for many years now too?
Joe Reis: Yeah. What year is it?
Juan: 2023.
Joe Reis: Three- ish years maybe? Yeah. So cool. I mean, a lot of this started as kind of a COVID project because I don't think I would've gotten into podcasting more for COVID. Because I think back then I think everyone's mindset was still very provincial and localized. Right? And so I just did it because why the hell not? I have nothing else to do. And so, you guys are doing it probably a year before and then yeah, it's a cool thing to do though. And it seems like everyone has podcasts. I was joking the other day like, " All of my phone calls are basically just podcasts right now when I talk to people."
Tim: There's a lot of podcasts going on now.
Juan: One of the things I'm pretty proud is that you go to listen notes. com, they're an aggregator and we're actually on the top 2. 5% of all global podcast. That is they aggregate over 3 million podcasts. So that's cool. All right. So what are you drinking, Tim?
Tim: So I'm actually on vacation right now. I'm in Dripping Springs, Texas. There is a dripping spring in Dripping Springs.
Joe Reis: I know. I looked at it and I was like, "Oh, it's literally a spring that drips." It's the craziest thing.
Tim: So I'm here with my family. We like to hang out with somebody else's pool, steal somebody's pool. But there's a distillery close by here called Treaty Oak that I really like. This is their Ghost Hill bourbon. That's really solid. So if you're ever in the Austin area, drive 25 minutes out, stop by Treaty Oak. But actually the thing I'm drinking right now is actually a Peach to Julep cocktail. So that's what I got going on over here.
Joe Reis: It's fancy.
Tim: Yeah.
Juan: And I'm having, there is some nice rum, Flor de Cana and it's nice and hot outside here, Texas. So I'm having just some sparkling water with some bitters. Nice, refreshing. And I think it's just going cheers to us having podcasts and people actually paying attention to us. So yeah.
Tim: Cheers Joe.
Joe Reis: It's awesome. Congrats. Yeah, and that's good. The listen notes seems awesome. I'm just drinking a diet Red Bull because why not? Got to watch my figure.
Juan: All right, a quick warmup question. So modeling and semantics is all about representation. So what object would you choose that represents you the best?
Joe Reis: So it was a bit of a doozy of a question. So I actually asked ChatGPT what ChatGPT would represent itself as. And it came back as a library or a database full of information. So that's kind of interesting. I'm either, yeah, I don't know, probably a meme or something. So my goal in life is actually become a meme of myself. And so I think that might be how I represent myself. How about you guys?
Tim: I like that. That's actually a good kind of a meta response. I like that. I was thinking maybe I'm like a pencil and creating, but iterating and flexible. It's got an eraser, right? You screw up, you can erase it. So yeah, maybe I'm a pencil.
Joe Reis: That's Cool. What about you Juan?
Juan: I'm going to go with, I can't find the objects. I'm just curious. I just kind of to talk to people, got to learn and just go, " What would be that object be?"
Joe Reis: So like a sponge?
Juan: There you go.
Tim: Sponge.
Joe Reis: You cook things up. Yeah.
Tim: Or a microphone.
Joe Reis: Or a microphone.
Juan: A microphone, microphone.
Joe Reis: Or recorder or something like that. Or sponge, it picks up the eraser bits when you're done, Tim's done.
Tim: Yeah. I make a mess. You can clean it up. Yeah.
Juan: All right.
Joe Reis: A mop, the mop. That's what he is.
Juan: Let's get into this. Okay, so we were having this conversation with beers and-
Joe Reis: It was a lot of beers by the way too. It was kind of funny.
Juan: It was pretty-
Joe Reis: So shout out to Ethan Aaron and all the people involved in the low- key happy hour in Vegas a couple weeks ago at Snowflake Summit.
Tim: Yeah, that was awesome.
Joe Reis: Made a sneak appearance for that night and that was super cool. It was kind of like a who's who of the data industry just walking around, bumping into everybody. We bumped into each other and...
Juan: We started talking about this. Well first of all, start talking about that you're writing a new book, so, you have a very famous book right now. How is that going? And now you're talking, you're writing a new book and tell us about that because that's how we got the conversation.
Joe Reis: Yeah, I got a famous book, Fundamentals of Data Engineering that's been doing really, really well. But after that, there's one chapter in the book I actually didn't really feel like it did the topic of data modeling justice. I kept getting a lot of comments and emails about that chapter in particular, like, " Oh, why didn't you cover this? Why didn't you cover that?" Or " Why did you cover this at all? Nobody cares about this." And so it was kind of the spectrum of response and I'm like, " Yeah, I do agree. I think that maybe the topic of data modeling needs to be looked at maybe a bit more closely." And also probably resurrected to some degree. If you ask, especially newer practitioners to the data field right now, when you ask them about data modeling, you get a variety of answers as in terms of what they think that is. But for the most part it feels like a lot of the, not just the tactical practices everyone thinks that data modeling is Kimball or something like that, relational modeling. And I think those are certainly techniques, but the art, the philosophy and really the why of data modeling and why you want to do it, I think that's been lost to an entire new generation of data practitioners. So as I started thinking about this more, I'm like, "Well, maybe I should write a book on this." And I started thinking a lot about it and later last year I decided to start writing it. I wanted to be done by now actually, and I thought it'd be done, but a few things happened. The very famous book also meant that I started making courses, which take up a lot of time. So that's been I think a blessing obviously. And then large language models. And I think the popularity of AI right around November, December of last year and into now it's red- hot. And I think for a lot of data professionals, that also meant you're rethinking a lot of, okay, so maybe not rethinking, but you're thinking, okay, so what does this mean for the industry? What does it mean for what we've been doing? Does it change anything? And if so, what changes? And are we ready for this? I think that's the other question, that was a question that we were talking about actually over many, many beers was, it feels like there's this mad rush into AI right now. But I mean, we all work in data. We all see data sets every day. And I don't know about you, but the ones that I see, they could be better to put it politely and to put it more bluntly, a lot of more flaming trash heaps. And so to try and put AI on top of this, I think it's an interesting idea. I'm very curious how it works out, but I see this as a huge opportunity for the field right now. Either we can start getting the fundamentals correct again, semantics, which is Juan's territory, data modeling, which is what I've been thinking about. These are very, very much related. These are basically siblings and we have a chance to get this right, which means the promises of AI can be achieved for real, but if we can't get this right, I feel like this, a lot of the interest and investment and frenzy around AI does have a chance of actually backfiring on itself. So yeah.
Tim: Love your take on this. There's a divergence here between thinking that AI is going to allow us to forget about the fundamentals and say, " Oh, well now we can kind of zoom straight to the finish line." Or there's a perspective of, oh shoot, the problems that we have around our semantics, around our modeling, around our data quality, around the access around data. Those things are going to be amplified by this movement and it's going to be even a worse situation than it was before. And I don't know how many people are in the middle of that spectrum versus, I know you faced a little bit of even controversy as you talked about wanting to write this book around semantics and modeling, right? And people kind of are like either, " Oh my gosh, I love it, we need this book." Or" Why are we spending time on this right now?"
Juan: So one of the things that comes to mind-
Joe Reis: We'll talk more about that. Sorry, inaudible.
Juan: ... is,when we talk about AI, I think right now, well we use the word AI and it's always been machine learning and now we use the word AI and now it's LLMs and generative AI. So we just kind of shift what the word AI is. And now if we look underneath what it is, the input to that stuff, we always say, " Oh, data quality, all that stuff." But at least for the large language models, which is with our current definition of AI, it's text, it's unstructured, and now we're going to go to images and the multi- model stuff, but it's really not about structured data yet. And I think, so we're seeing all this excitement around that stuff, but I think there's like, " Hey folks, you know you want to go tie in your internal data to this whole ChatGPT and stuff. But that's structured data." And now I think the argument is that, oh, there's always way more unstructured and taxed and all that stuff, and that's what we should go focus on. I would actually argue that, well, I mean that's true. I would argue that the structured data smaller than it is for the unstructured is higher, has more value, and I think would solve more of the questions people have than just the unstructured. Put that claim out there, put that position out there and happy to get pushback from it. Now when we start doing that, we start focusing on the structured side, then we're like, " How do you put in structured into your LLMs?" And we'd look at all the trainings on vector databases and how do we do embeddings, all that stuff. It's not about structured data into itself. And I think that, so if we want to start putting structured data combined with these large language models and generative AI, this is where the modeling in semantics is going to kind of... Yeah. And right now we're not talking about it. And I think this is, it's a fundamental part that we need to do. So anyways, that's kind of my opening rant here. inaudible, Joe.
Joe Reis: Completely agree with your opening round, right? It is the blind spot right now. And you're absolutely right. You talk to people like Bill Inmon, right? He's working on text data. That's what he's cared about for a long time. If you talk to him, it's like the data warehouse that he came up with back in the day. He doesn't really think about that very much. It's like that's pretty old hat, the text is. And I think to this point that there's a large amount of text data in a lot of companies, and driving meaning from that is important. But tying that back to those systems of record that you're talking about, those rows and columns of very valuable operational data that businesses need and live and breathe off of, that's if you can tie all this stuff together, that's amazing, that's powerful. And all the images you collect and tying that back to all the data in your ERP system and whatever other data you have, I mean, tying this all together, I think if you could do that, that's cool. We've achieved something that we've been try trying to do as an industry for a very, very, very long time. And I'm bullish on that. I think angle, right? And if we can get there, that's cool. But you have to be realistic too and understand what's in between here and that vision.
Juan: All right. Let's dive into the data modeling part. So where are we right now with it? And give us a sneak peek of what's going through your brain that you're trying to put it down as paper.
Joe Reis: Yeah, some things that come to mind. So there's a talk, I kind of debuted it in Vancouver a few weeks ago, called Data Modeling is Dead, Long- Lived Data Modeling. And so it really comes back to the, I think, the premise that as an industry we've sort of lost our way with respect to having, I think, a coherent view of data with a perspective of business processes rules, vocabulary, semantics and so forth. We're so used to capturing or seeing modeling as really an exercise of capturing ad hoc queries at this point. And I think responding to ad hoc requests that we've really lost sight of the bigger picture in terms of, I think the grander data modeling practices like conceptual modeling for example, then logical modeling, then physical modeling, right? There's a couple of threads to this. One is I think we're so focused on the tactical, we've just lost everything else, all perspective in the context. The other part of this is it's because... and I hear this often, modern data stack especially, I like it a lot. It's achieved a lot. But one of the challenges is you can throw a computer at anything. And so when I talk to analytics engineers for example, they're like, " Well, I can just throw more compute at stuff, so why would I need to model it?" And I'm like, " You could throw a lot of compute at stuff, but do you understand what it is you're trying to model in the first place and how it fits into the bigger picture?" And then you zoom out across the spectrum, and ignoring analytics, for example, which is our domain, all of us, you talk to software engineers, it's the same thing except they're the ones creating the data that the analysts and the machine learning engineers depend upon in a lot of cases. And it's even worse in software engineering. The notions of data modeling, it's, for a lot of software engineers I talk to, it's nonexistent. You're talking about event streams for example. It's just like I package whatever I need to package into an event, throw it off, you consume it, there you go. Have a nice day. Right? And if you have a database, a lot of databases these days are managed by ORMs, object relational mappers. So if you've ever written a Rails app or a Django app for example, or many other languages, node, whatever, it's like ORMs are great. They also give you so much flexibility that your database can basically implode in itself if you're not careful about your model that you're creating. So what I see there is people will make models according to, say, a form that they built or a web interface or something. And that's sort of the quote model. Again, this is where the data is going to, first is created, and then obviously analysts pull whatever they can. And so it is a cascading effect. And I think when we talk about modeling, it's like how do you model across the data life cycle? How do you think about the coherence of business concepts, rules and processes and so forth you're trying to capture in the data across, whether it's application, analytics, machine learning, streams, whatever. I think that's a huge piece I think we're missing. And the other part I'm looking at is if I look over here, there's data modeling books. I have Codd's books, I have Kimball, blah blah blah, but it's like data vault and so forth. But there hasn't been, I think an evolution in thinking in data modeling for over 20 years now. The last big one was data vault, and since then I think there's been some attempts, but I think they're too focused on particular silos and don't expand the picture to see data as a whole. Which I think, especially with AI right now, I think it gives us a chance to see data as a whole. I don't see why you would need to silo it, but these are things that are on my mind. Again, some of these ramblings are, I think, somewhat coherent. Some of them are, I'm still thinking about, but this is what's on my mind. That's what you asked.
Juan: Yep. All right. Tim, you want to go first? Let's pick this apart. I like, I like. I got a couple thoughts. But you go first. You, go for it.
Tim: Yeah. I want to start with a follow- up question, Joe, which is, what's the biggest risk that we run here? Do you think it's a... is it a productivity risk? Is it a cost risk? What do you think are the biggest walls we're running into by not thinking enough about modeling?
Joe Reis: I think the risk is that we're going to do dumb things more quickly. So you'll get the hallucination that you're productive.
Juan: Well that's fail faster?
Joe Reis: You'll fail faster. Well, you'll just may do dumb things more quickly, but you won't even know you're failing, right? Until it's too late. Yeah. Because I think what you're going to see giant productivity gains, but at the end of the day, did you gain much? So that's it. Because I think one of the big problems we have right now is obviously large language models are the great, super what they do, they generalize really well. They're also black box solutions. You have no idea what happens in them. You have no idea if the output is correct. Obviously things like knowledge graphs for example. There's a lot of research going on where that might solve that hallucination problem. Maybe it does, maybe it doesn't. But the problem is if we don't start at least having this conversation in terms of, I think data correctness for example, what does it mean? The risk is that we actually do things too well while doing things too badly. If that makes sense. We'll have the impression that we're doing things awesomely, but then it'll be entirely wrong.
Tim: Yeah. Well and I like your comment and your tie in generative AI as well because I think sometimes even now, right? We're very enamored by the magic of it all and we're just like, " wow, look it wrote the SQL query. Great." Can we just have generative AI write all our dbt for us? Is that a good thing? Probably not. It's an interesting productivity hack, but...
Joe Reis: You got to know what to look for. That's what I mean by you could be doing a lot of things, but you could be doing them all wrong. If you didn't know what to look for in a SQL query that it outputs, how would you know that it's right or wrong? I mean it's pretty... So I do this with my kids a lot with homework. So we use ChatGPT quite often and it's just to check homework. And I'm like, " But sometimes it gets it's wrong." And I'm like, " So you need to check GPT's answer and tell me why it's wrong now." And I think that to bring it to education for example, where we're all educators for example, and I think that's one way I think teaching is going to change is you're going to have to teach to tell people how to validate stuff in the future. But this comes back to business. How do you know... So if a CEO types into the new generative AI BI tool, because everyone wants that or the catalog for example. And it's like how do you know the data's right? How do you know the output's right?
Tim: Yep.
Juan: Yeah. So I think the education part is critical here and which is my intended, we need to be more critical. And I think, this is not just an AI thing. I mean I was organizing a round table last month about it and people were bringing this up and somebody said, " We've been saying I just read it on the internet. And then you're just saying these things out there too." So it's the whole being more critical as something that is not just now, it's been going on for a long time. And every generation can argue that the last generation, the next generation is-
Joe Reis: So stupid.
Juan: Yeah. So forth. Right? But still we should kind of... I think being critical thinking is a key thing here. Now I love this quote, Tim, we got to do our t- shirt store one day. This one will say, " do dumb things more quickly, but you don't know you are failing." No, I mean, Joe quote, like that. So what the meaning part, this is goes back to, I was having the conversation today. Data literacy is a topic, I have my friend about this is I hate people using the word data literacy because that means that people are illiterate in data. I'm like, " That's not true. People are not illiterate in data, actually..." and we call the business side needs to get data literacy. The business side actually knows their own data and their own business. They may not know all the tools and all the techniques for doing statistics and stuff, but they know their data, they know what they care about it. Go down to the data side, they're the ones who have that one, I would argue they're business illiterate. They need inaudible. So this is like call all the time is that we need to, the folks who are managing the data, they need to understand how the, I'm using the word business, but they understand how their domain work means. And again, this goes back to education and that's where modeling comes in because modeling and semantics, I'm going to just bundle these two things together because modeling is just a way to understand and understand these semantics, the meaning of what we're talking about. I mean, you got a green board behind you. If we're going to go start talking about something and we're confused, we end up just going to the green board right there and start drawing things out. And guess what? Probably going to look like bubbles and lines and guess what? That looks like a model, data model of something. Right? And I think the other issue is that as technologists, we just don't like to talk to people. I just want to get go code and like, well I got the form and I see the code for that and I can create a package of that which is in JSON and just send that and then I get that and I'm going to store it and then I'm done and then I'm going to just dump that into a database and there it is. And now go do your analytics on it. And then somewhere else people saying, " I want my data to look this way." And so they're basically creating a new UI form for the end result. Now transform it, so you're just doing a bunch of syntactic transformations and say, " Okay, this thing now fits here," but you don't know if that was the correct thing. And because just go talk to people. So anyways, I think right here is the modeling is all about the people side, which is we don't like to talk about people because we're technologists. That needs to change.
Joe Reis: Well, yeah. And it's interesting because I like to punish myself and go on Reddit once in a while and look at the data engineering subreddit. I very rarely post there and it's great people and stuff. But you know, you see posts, like the other day, somebody's posting, " Well do I need to use Kimball modeling or do I just..." and I'm like, "Well, what's your alternative if you're not going to do that then what's..." Because a lack of modeling is still modeling, it's just a really crappy model, you're still trying to represent the reality that's in your head. It's just Kimball I think gave you a really good way of doing it for analytics. I mean it works and it's worked for decades. So my answer would be do you think you're smart enough to reinvent the wheel in this case? I mean, again, something that works, I mean if you want to go for it, but I'm not saying... The other approach I'm taking too is I don't think that there's one single way to model. Right? I think that's one of the big mistakes we make as an industry. And that's why I posted, they posted the other night about it feels like there's religious wars in modeling. I think that's true, it is true to some degree. Right? There's various factions. The relational camp has been hammering on that for 50, 60 years now. 60 years almost. And dimensionally analytics has its own sectors and stuff. My whole approach is pick what works for your situation, just understand the tools available to you. So the analogy I'm giving right now is mixed martial arts, before UFC 1, or actually before I would say Bruce Lee inaudible, but anyway, UFC 1 is what really came to a head with mixed martial arts. But it was like until then, if you always grew up in the eighties, which I think a lot of you did, it was like there's always like the kung fu master who had the secret art that was the most powerful thing in the world, but he would never show you because if he looked at you you would die or something like that. But the question was always what would happen if Mike Tyson and Bruce Lee got into a fight? Or Wrestler and Bruce Lee or whatever, Hulk Hogan and Mike Tyson get into a fight. These are the questions, right? But it started actually being realistically answered in the nineties with UFC and you come to find out that there is no one true martial art, there's a bunch of them and you got to know all of them and you got to know when to apply them. And to me that's no different than data modeling across the data life cycle. You need to understand the different techniques that have been around forever. You need to know the new techniques and be willing to apply them to new situations. And that I think is going to bring about a new era of just how we deal with data and how we talk about data, how we use it, et cetera. But until we bridge that gap, we're operating in so many DAMN silos right now. It's holding us back. That's the crazy thing. We like to think we're making progress, but you look to your left, software engineers, you look to your right, whoever the hell is consuming your data, it's like everyone's operating their own silo. But it's all on the same sort of data that flows through. It just changed i. e. modeled differently but it still carries the same concepts hopefully through. Right? And so we need to get back to that notion. But I think we need to get rid of these stupid idiotic battles about the one true way. I have a book 50 Years of Relational Databases from Chris Date and that whole book is him just pretty much trash talking anybody who doesn't agree with the relational model, 300 pages of this crap. Good book. But I think it's symptomatic of, I think of an old school mentality that if you're not with us you're an absolutely anatomy, right? That's right.
Tim: Yeah. You're either a part of the religion or you're not. I like this analogy you're using around mixed martial arts and it's not like, oh Brazilian jiu- jitsu also always wins or TaeKwonDo always wins, it's knowing what techniques apply to which. And knowing your opponent, and this makes me think about lately there's been some buzz around things like activity stream and things like big wide tables and things like that. What do you think about some of these like, oh here's a clever approach to solve a lot of use cases. Are those just other tools in the toolkit?
Joe Reis: I think there's other tools in the toolkit honestly. Activity stream. I saw that come out. I was like, that's cool. I think it fits what Materialize is doing for example or inaudible schema. But I think that's dope. If it works for them then it works for them. Right? My whole thing is just to know what the trade- offs are, what's a trade- off? You take this technique, inaudible the trade- offs.
Juan: This was going to be my question. So what works for them? What does work mean? What are the things that you should be considering the trade- offs? People listening here is like, " okay, I have now passed the first bar saying I don't need to choose one thing. If there's end things I need to consider the end things. Right? So I'm not part of the religious word that number one is the best one and that's it, right?" So what are the criteria to understand, could I compare and contrast and figure out, oh for this particular use case this is the best, what works the best for me?
Joe Reis: And it's a good question, right? Because I mean ultimately it's about what's valuable and useful. I mean that's how say Bill Inmon would define what he's been trying to work on just is valuable. Is data valuable and is it believable and useful? But the trade- offs right? That's an interesting question. So how do we get to this situation in the first place? I could argue it's about the trade- offs, right? We're trading off time and efficiency and money and versus quality of rigor and those sorts of things. And right now the pendulum is swung basically towards fast relaxed versus rigorous and formal. But what's interesting is if you go to Europe for example, which I know Juan you've been there, I don't know about you Tim, but Europe's a different ballgame there. They move a lot slower business wise, they just do. And the thing is data modeling there is much more rigorous and much more formal versus here where people just move fast, break things. That's how we do things. It's a feature and a bug. But I think you talk to data modelers or data practitioners in the States versus Europe, it's a much different, you get two different answers in terms of how you should do stuff. So I think it's the trade- offs really here. It's like people, if you talk to most analytics engineers or data engineers, I don't have the time to think about modeling. I'm just going to make say one big table to your point Tim, and that's how it's going to be. So I don't know, it's an interesting question.
Juan: The culture aspect here in countries, this is a very, very important one that you bring up. This would be interesting analysis to go, just go on LinkedIn and look for people who's title called data modelers. I would believe that they would, I don't know, apply to 80/ 20 rule, 80% would probably be in Europe.
Joe Reis: But I'd want to take that bet too. Yeah. It's just a different culture over there. I mean they're doing stuff like data vault for example, which I mean I don't know very many people in the states are doing that, but that's a great methodology but just requires a lot more work and a lot more investment and it's just here for whatever reason, the investment is more like I just want to get an answer today. And that's maybe part of the problem, but that's a trade- off like, how accurate and how correct and how holistic you want to be versus just getting an answer out the door. I mean in recent podcast I did with him, Tristan Handy, shameless plug of the Joe Reis show, go check it out. It's on Spotify. But Tristan, he's the CEO of dbt labs and he's not blind to the fact that there's like dbt models sprawl everywhere. He pointed out a company that had 39,000 dbt models and it's not like he said this is like awesome. He's like, "Yeah, this is a huge problem that we got to tackle because you got to..." That's like 39, 000 different, at least different concepts, maybe more. There's just somewhere in a data warehouse.
Juan: 39 different things and then those things are probably duplicated. Think about the semantics, they're-
Joe Reis: Bingo. Yes, exactly. So it's like, yeah, so he's saying the complexity issue is definitely something that he's definitely thinking about and trying to tackle and it's just... But this is what happens. One of my coach actually, my fitness coach, she is a data analyst as well and she's telling me the other week like, " Oh, I'm doing a data model." And I'm like, " Oh interesting. Tell me more about that. And when you say data model, what do you mean exactly?" Right? And she's like, " Oh, I'm making dbt models." It's like, " Huh, that's interesting." And so to her that, again, semantics the term data model even, it means a lot of things to different people. I wrote an article about this a few weeks ago, WTF as a model because it's like you have a machine learning model. So when I talked to machine learning practitioners like, " Oh, I'm modeling right now." I'm like, " Okay, what do you mean by that?" It's like, " Well, am I making a machine learning model." Like, " What do you mean?" And i talked to app developers and model's a totally different thing too. I mean, in a lot of cases it's literally a model file in your ORM. But anyway, that's the predicament that we are in right now. To answer your original question though, how do you know if you've done it? I think it really does come down to what you... Given the trade- off you have at hand and the constraints, what would you define as a success? I think it's the only way you could really answer that because you couldn't just say, well it has to achieve the highest ROI for example. Because I'm like, " Well, but in this case you probably justified yourself that you just did that." So it's like I don't think you want to achieve the lowest ROI outcome.
Juan: Yeah, well there's some obvious there. There's the obvious, oh we want to make money, all that stuff, right? But then it gets much more profound here.
Joe Reis: Yeah.
Juan: I don't know what's inaudible, Tim.
Tim: Well I wanted to ask a question which is that I think sometimes, especially in the US when we talk about this sort of faster, more like some, I think we move the slider on the scale from efficiency to resilience. We love to slide that slider real high, real heavy on the efficiency end of that slider. And I don't see data teams spending a lot of time, first of all thinking about modeling upfront but then not really spending time to refactor. And I think that there's an art to, oh, okay yeah, we're going to move fast. Maybe let's just use the dbt scenario for a second, right? Oh we've got a hundred models now. Oh shoot, maybe that it's time for us to go look and see how many of these models deal with customer, right? Oh, 10 of them do. Oh, shoot. Well maybe we should have one customer model and be taking into account any dimensions or supplementary tables and things like that that need to support customers. So that way we're not having 10 customer dbt models. I don't know if you're seeing that problem too, Joe. And if that's something we need to solve if it's a cultural problem.
Juan: So let me add to this, throw it to you is how are we dealing with tech debt and data?
Joe Reis: I kind of separate this into three categories really. In the software land it's easier because you have tech debt and sometimes it's data debt, but especially for data teams you have three types of debt. You have tech debt, maybe pipeline debt, I don't know, infrastructure debt or whatever, data debt, which I think more of we are referring to how are you controlling the decay of your data over time? Because it will, concepts will erode and data will become less relevant and so forth. Or it'll just be plain wrong at some point or it might just disappear too. Anyway, I digress. Organizational debt takes into account basically when you start out as a data team, picture this, you're given a punch pass and you have 20 punches on this thing and this is the amount of times you can erode trust in your group. So you have 20 screw- ups basically after the 20th screw- up. I don't know what happens to you. Probably bad things. So this happens a lot with data teams, software teams too, but I think data teams, because there's more of a feedback loop with the business. So say this report's wrong, it's an obvious one. Yeah, it's a punch in your past, data's not available, another punch in your past, right? Keep accumulating these things. And so that's organizational debt that I think data teams in car with quote the business. But what was the last point that you hit on though, Juan?
Juan: Well I was just asking how are people dealing with data tech debt today, right?
Joe Reis: Yeah. Data tech-
Juan: inaudible point is like yeah we got to refactor. Are people even thinking about refactoring and taking a pause? I mean, our engineering team, all engineering teams will go on like, " We're going to go do this stuff, we have a sprint, but we're going to put some time to refactor to go look at the debt and writing. There's a week that we're going to go do stuff." Is this happening?
Joe Reis: Yeah, I think it's one of those things where it's kind of like all of us I think also say especially at the beginning of the new year that" Yeah, I'm going to get my life in order and really get on a plan. I'm going to exercise and eat keto and really, really get on the ball, not drink as much," but about three weeks in you're just like, " Yeah, whatever. That was hard." So I think that's a lot of teams really, right? When it comes to debt it's like, yeah, I think that the ambitions are good. I don't think anyone has malicious intent or is it inherently-
Juan: But is software like that?
Joe Reis: Oh, software's like that for sure. Every software team I've worked on, it's definitely like that. You have your tickets and I think the good teams definitely spend a certain amount of time trying to chop down debt. Each sprint, so different teams have different ways of doing it. You have to be very intentional about it. But in data it's interesting because you can actually mask over your debt by just making a new query and just say, " Oh, well here's your answer to that." And so you didn't really change the situation, you just changed the output.
Juan: Data, you can mask over your debt by creating new query. I would say in data you can mask over the problem by creating query, creating more debt.
Joe Reis: You just create more debt, yeah, you create more debt. But it's like I say that I just need you to tweak this report though, right? I'm just going to add another filter to it and like, " Here's your report." So that's what I mean, it didn't change the underlying fact that you got it wrong in the first place and you didn't address the root cause of that, right? But you did address that well here's your answer to that question. So it's easy to sweep things under the rug, so it's interesting like, I love dbt for example. Another great tool is Looker. Looker I'll argue it was one of the first popular semantic layers, so to speak. It was coupled to the BI and I won't get into all that nitpicking on that, but the whole purpose of Looker was to define something once and use it anywhere. So just as you would in quote, do not repeat yourself and so forth. And what was crazy is that I seen Looker ML files that had so many duplicates and so much repetition and didn't take into account dynamically scaling out the type of problem you're trying to solve like you if you're writing code conditionals and so forth. It was literally just copy and paste, change a filter there and so forth instead of using conditionals and stuff like that where it's just like, okay, so it was obvious that somebody asked you for a something really quick and you just need to get an answer that quickly. And the other crazy thing is Looker was supposed to be the self- service layer. You're supposed to be able to do self- service analytics. The holy grail of analytics is self- serve, everyone wants to do that. And lo and behold, so self- serve obviously means that the end user can just use a tool, get their analytics and then you're done, follow up. Right? Analysts can work on the definitions neatly and so forth. And the separation concerns are there. And all too often what I would see also is that analyst is supposed to be working on building a better model. They're pinged on Slack probably say, " Hey, I need you to make a report. Can you also send it to me in Excel?" So, so the whole situation is just bananas. But this is the reality that I see quite often actually. So, interesting world. Sometimes I figure maybe I should go do cattle ranching or something. That'd probably be more fun. So I don't know.
Juan: That's a topic for another podcast. If you were engaged, what would you be doing? I think we have good conversations.
Joe Reis: That would've been a good icebreaker as well.
Juan: But I want to bring up this comment here that Jefferson is sharing. Sprints are often the suppressor of good upfront modeling. Thoughts?
Joe Reis: I think there's some truth. In fact, I was actually on a... chatting with this Slack group of all these software engineers I'm friends with, really hardcore ones too. And we got on the notion of scrums and agile really. And one guy was like, " I really hate scrum and agile." And I was like, " I think, you probably hate how it's done, right?" Because the essence of scrum for example, that came out of lean manufacturing that it actually, nothing to do with software, was applied in software. But it was like, it's something that came out of a, I think Toyota or maybe the one of the lean people. So it's interesting because the whole notion is a banish waste, that's all it is, right? What's interesting with sprints is that we actually spent... it's a very wasteful activity in a lot of cases. So I think it's how you do your sprints is incredibly important. But I'd be more curious to dig into what he means by the suppressor of good upfront modeling and what he means by upfront modeling. The way I would imagine upfront modeling is, at least the way that you'd probably do it in the old times is there's a lot of requirements gathering, a lot of sitting down with people and stuff. And that's one thing I've been thinking about too is maybe is there a better way of doing this in this day and age? Because I think that it's definitely more of a waterfall approach, which obviously would be kind of contradictory to the notion of a sprint. But it's... some people like Larry Burns for example, and John Giles and others definitely point towards the need for more agile data modeling. And I do tend to subscribe to that viewpoint. But it's-
Juan: So I think there's two things to, we should confuse them. One is the modeling technique. Give me why, and then what is the methodology of how you're using that technique, right?
Joe Reis: Correct.
Juan: We're a waterfall approach, boiling the ocean, you're getting all the requirements and then we're going to do this right? Versus an agile methodology. So I think that, and then the methodology can apply to whatever modeling technique that you want to go do depending on whatever success you're having. So I think that's the one.
Joe Reis: And it's interesting too. So one thing I would urge people to do is revisit the notion of data model patterns. Especially when you're at the conceptual phase. This is something that have been advocated quite often with, was it Len Silverson? For example. He had three books, fantastic books, the data model resource books, volumes one through three, 2000 pages of nothing but model patterns. And so if you're a retailer for example, these patterns just lay out how does a retailer work, how do you think about all the entities and a retail model, not just entities, but stepping back, just like things that happen in a retail business. Right? Things that those things happen too. You got a product, you sell it to a customer. These raw patterns and the thing is, these patterns still apply today. It's not like the world of retail has completely changed the point where it's like you don't sell things to people anymore. That still happens. So I think to a large extent the just reusing patterns I think would maybe help with what Jefferson was saying here with upfront modeling. Because it doesn't need to be faster. I think the big complaint was everything takes too damn long.
Juan: So this is all about having existing industry models. I mean retail, you'll have the orders, customers, I've been doing insurance, some stuff and you have claims and covers details and underwriting. All these things are the same and shouldn't, they're not changing across the in industry, across companies, the essence are there. But I think going back to the rigorous, seeing that pendulum, you can see some folks being very pedantic of, we have to go use this model as is. And I'm like, then this is where he fits. No, some people will be in that camp and I'm like, " Just use it as an inspiration maybe magically as it is, it works perfectly for you. Okay, perfect." Maybe 80% of it work, maybe 20% of it works or whatever. At least you're not starting from zero, not being Brent in the fricking wheels.
Joe Reis: Correct. Yeah, exactly. No, you're a thousand percent spot on. I agree. It's take what works. Again, it's back to the mixed martial arts. What applies to your situation? What do you need to tweak, right? If I'm in a mixed martial arts event, for example, I'm not going to sit there and try and do a perfect triangle choke, if you know what that is. It's like I'm going to do adapt to the situation and nail it as best I can, knowing that the other guy on the other end is probably knowing what I'm doing and it's going to try and get out. That's how reality works. And the thing is, business is slippery, especially these days. Things change quickly, but there are processes that probably don't change as much. So try and capture those and build around those things. And I think that's the whole point of this is it's just what we're doing right now is we just become so myopically focused on what's in front of us, directly and focus in front of us for this hour even. I need to respond to this query. And the problem is we're losing the entire context of what we're doing with the business and then if you extend it to data mesh between businesses. So it's a very fractal problem. But I do agree with Jefferson's initial comment though. Sprints are often the suppressor of good upfront modeling. I think, yeah, I tend to agree with that. I think sprints are also a suppressor of good upfront a lot of things, not just modeling. So the way a lot of people do sprints is I think quite poorly done right now. So I've managed poor sprints before so I know what I'm talking about. So I think we all have, I'm not going to say that I'm perfect.
Tim: Yeah, well sprints can be something that folks get very religious about also, right?
Joe Reis: Oh, yeah.
Tim: And if you embrace agile software practices in data but don't embrace thinking about business value and thinking about data products and how you're actually using agile data development to accomplish said value, then you're just being obsessed about a tool or an approach instead of the value you're actually trying to create.
Joe Reis: Religion. Yeah, exactly. I mean or philosophy as Juan was yelling at me the other night about, no, I was just messing with Juan actually him. I mean-
Juan: It was a Friday or Saturday and I think it was 10, 11 PM for me. And I already had some drinks. I'm like, " Why is this such a bad... It's philosophy." I was being philosophical myself already that night.
Joe Reis: No, no. And I was like, " Yeah, he's right. I'm just going to mess with him." But it is interesting though, I mean you bring up some interesting points there too. We get so caught up in the dogma really. And I think that especially now, again, AI has so much opportunity to change businesses and I think change the way we do a lot of things. We really do need to capitalize on this moment and get our house in order. I think what's going to concern me is companies are going to throw large language models on top of their whatever SharePoint, I'm just joking, but probably to that too. And it's going to be weird. So I mean you have two choices. You can assume that what these generative AI is outputting is correct. Right? Or maybe a third assumption actually is going to say the other one is you can assume it's not, but you can see your data's been wrong the entire time too. Maybe that's also the case. So I don't know. But it's going to be an interesting world and what I fear is that we're going to enter more of a whole of mirrors type situation than our businesses where nobody knows what's right. Whereas before, I think the original point about when we first started, Juan, where it's like business people, they know their numbers. You show me a sales guy or saleswoman who doesn't know their sales number right now? Every salesperson knows their number. If they don't, they're out of a job. You know your number like the back of your hand, that is the one number you know. You know that probably better than your kid's birthday or anything, you know where you are. So you know your business.
Tim: Yeah.
Juan: This is the key thing right there. People know, we're using the word know here, it's knowledge.
Joe Reis: Yeah. You harp this all the time. It is knowledge, it's all it is at the end of the day. But I think you're right though, and what you're saying earlier where it's like, I think it's more of the data people that we got it backwards. We need to focus on the business and talk to the business in ways that they understand. And another article I wrote was, a business doesn't care about quote data. Because they don't. Go to a salesperson, talk about knowledge graphs, for example, they'll just look at you like, "I have no idea what you're talking about." Unless you happen to sell knowledge graphs. They'll just say, " Juan, have a drink and sit down. We can talk about something else." So, look like you have a lot in your mind there, Juan. But that's the reality of it. I mean most data people want to talk in terms of whatever we're trained to talk about and what we talk about amongst ourselves, but a lot of people don't live in our filter bubble.
Juan: Definitely.
Tim: Yeah, I think before we go to our lightning round is one of the biggest takeaways you think that folks should have is kind of going back to this mixed martial arts analogy. Learn the tools of the trade, figure out what they're good at and what they're optimized for and how to make the best of them. But really focus on modeling, especially in the advent of generative AI and how that's just going to amplify everything. Think about modeling more holistically and think of it in terms of right tools for the right job and really tie it back to the value that you're trying to drive.
Joe Reis: I think that's beautifully put. And if you take the mixed martial arts approach, it can be awesome. Like Mark Zuckerberg here hanging out with the UFC champions.
Juan: Inaudible.
Joe Reis: Yeah, so funny. I don't know why I get such kickoff of this whole Zuck versus Musk. What a crazy situation.
Juan: I just want to close that with one thing before our lightning round.
Joe Reis: This is crazy.
Juan: I think the generative AI I started off with, oh, AI and not large language models. It's all about unstructured. We're not thinking about the structured data and we're going to start putting these large language models with structured data writing queries over our database. And we're going to, my hypothesis here, I'm going to bet on this, is that we're going to start doing these, chatting with the data and having all these natural language questions translating into SQL where our underlying, there's no understanding of modeling and it's just going to fall flat. We're going and look at cycles in history, we've had AI winters, and this is something that we can kind of say, oh, the promise of this stuff, but our most valuable data is our structured data, our analytical and transactional data, and we're not being able to leverage that with our AI. And that's we're going to go fall flat on that.
Joe Reis: Bingo. Totally.
Juan: Now the way my hypothesis is that the way that it's not going to happen, we're actually going to succeed. Where we can really leverage this is putting the semantics, the knowledge, and I've actually already been doing these experiments, I'm writing a whole poster about a benchmark. So I'm creating this benchmark where it's input are just questions. The underlying database schema is a schema of an open, taking some industry model. I'm working on insurance, stuff like that. But it's going to be in the physical layer. It's not very clear semantics. So you're going to take your query that's going to translate it to SQL and it's going to work for small stuff, but more complex questions where you're actually going to get KPIs and metrics. It's going to fall flat and you're just going to invent stuff right there and then put the semantic layer on top where we're going to have everything well- defined governance, all that stuff. I think that's where we're going to go see the difference. And we're going to have to shift from, we're going to be efficient on things and immediately we're going to fail. And it's going to be obvious because we're failing because the queries are giving just bad, not even compiling. That's how we're really going to fail and then we're going to find that pendulum. So that's my hypothesis. Hitting on this right now.
Joe Reis: I totally agree. It's interesting too. I know we're coming up on time, I know you wanted to do the lightning round, but it's interesting because I feel like we're in a classical machine learning winter right now. So my friends and I would joke back when deep learning came out that we were in a SVM sport vector machine winter since we used to work on and now we're in AI, all, if it's not LLMs, right? You're in the non- LLM winter right now. So, it'll be back though. I mean a lot of these techniques, they still hold weight. They're still awesome. It's just different tools for different jobs. It's all they are.
Juan: So this gives us a, let's hit our lightning round and our first question, let's keep it quick, quick yes or nos. Number one, are the past principles around data modeling out of date?
Joe Reis: No.
Tim: Perfect.
Joe Reis: Yes. Or quick, yes or no.
Tim: Well, I want to ask one B to that though. What about for things like streaming for example, are there parts of the data modeling approaches which are unexplained, under fleshed out?
Joe Reis: I think streaming needs to be fleshed out in general in terms of data modeling. Everybody I've talked to, people who have built the big streaming systems. Nobody has a consensus on streaming data modeling right now. Nobody.
Tim: Okay.
Joe Reis: That's a wide open territory.
Tim: All right. That resonates with me too. Second question, data architects versus data and analytics engineers, are data and analytics engineers the future keepers of the model?
Joe Reis: Yes.
Juan: Quickly expand on that one.
Joe Reis: Okay. I just think the roles are becoming more and more close to the metal, so to speak. I think the notion of a data architect, unless you're at a larger company, that they don't exist anymore.
Juan: Okay. This is good. So data architects and larger companies, data engineers, analytics are going to be the owner for smaller companies?
Tim: More full stack data engineer type folks, right?
Juan: Right.
Joe Reis: Yeah. Just because you have to. Yeah.
Juan: So as, next question, as organizations look to take their own knowledge and data and combine it with generative AI, whether custom LLMs or small LLMs, will semantics and modeling be the biggest barrier to value?
Joe Reis: Absolutely. Yep. A hundred percent. For reason we just discussed, yeah.
Tim: All right. Fourth question, we talked about sprints and iteration around data towards the end of our chat today. Can data modeling happen iteratively in sprints?
Joe Reis: It can and it needs to. So I think we need to reevaluate how we're doing modeling right now. I think that's part of the crux. I think we need to figure out how that fits into the reality that it's going to be part of a sprint. We aren't getting rid of sprints, we'll get rid of data modeling before we get rid of sprints.
Juan: And this is my book and I have a whole chapter on how to do it inaudible modeling.
Joe Reis: Oh, nice. I'll go get your book. I need an autograph version of that too.
Juan: I need to get an autograph, my version of your book, which I have.
Joe Reis: We'll let this happen.
Juan: All right, take us away first with takeaways.
Tim: All right. So we first started off by just kind of mentioning your book, Fundamentals to Data Engineering and also us getting together while we were all in Vegas with beers in hand and talking about the state of data and the advent of generative AI. And one of the things that you had mentioned both in live when we were chatting but also today, in our session today, was that a lot of folks when they saw your book, you had sort of one chapter around data modeling and some folks were kind of asking, "Well, why is that kind of there in the first place?" And then other people were asking, " Why only one chapter? This is so important. There's so much more that needs to be said around modeling, especially in the advent of things like generative AI", which are going to take all of our data issues and our opportunities and simply amplify them. And I think it's exciting and interesting that you're going to be digging more into that and be writing a book around that. And you had mentioned that the art and the philosophy of data modeling in general has been lost and we aren't ready for AI. And there's a huge fundamental opportunity to get the foundation right, so that the promise of AI can be real and maybe we can head off this winter that we may be destined for around LLMs if God can save us on it and if we can take proper data engineering and data modeling and general data practices to it. You also mentioned about tying different types of knowledge and information together. And you mentioned for example, Bill Inmon, right? Everybody knows him as being a maven and one of the godfathers around relational database modeling and the enterprise data warehouse. And right now he's focused a ton on text. And I'll throw in a comment for myself. It's been interesting to watch the career of Bob Muglia, the former CEO over at Snowflake and now he's obsessing over text and unstructured data and knowledge graphs, right? And it's the marriage of these things together that's going to be really interesting. And so we have to be honest about, you had mentioned we have to be honest about all the work that it's going to take to get from here to there. You mentioned a talk you gave recently in Vancouver. Data Modeling is Dead, Long- Lived Data Modeling. We've really kind of lost our way around having a coherent model around data. Models tend to be aligned these days more towards the form, the web UI and that kind of dictates a lot of the model. Also ad hoc requests are dictating the model quite a bit and how we're approaching modeling and really we need to zoom out, we need to not be religious about it. We need to think about all of these modeling approaches as tools in the toolkit, relational database modeling using Kimball maybe that's Brazilian jiu- jitsu, but you can't just focus on the one art, right? You got to focus on everything and use it together. There's no one true way and these are just different tools that you have to bring together. You got to know what to look for, you got to focus on value. And there was a lot more there. But Juan, I'll pass it over to you for you anyways. Thank you.
Juan: The trade- offs, we're discussing like" Oh, make sure it works for you." So what does work mean? First of all, just you have to find what success looks like. So to figure that out and I think usually the pendulum goes into being very efficient as fast as possible versus the quality and being more rigorous. And I think this pendulum swing is right now generally more on the efficiency side, but it's very cultural. If you look at the, I love looking at this, if you look at the EU, people do business, they're a bit slower about that stuff, how they do business. So they really take their time and they're focusing more on being the rigorous part. So I think it's always like do you want to get an answer as fast as possible out the door versus being more rigorous. So I think this is being able to understand the trade- offs and that's what you need to be able to go look into. And also very quickly discuss on what is a model? Everybody uses the word ML models are different from data models, different from dbt models and app developers have their own models, which can be even the model file. So we got to go figure out which model. Talk about semantics here, right? Talk about tech debt, right? So are you refactoring how you're dealing with this data tech debt? You said three types of debt. I like this tech debt, which is like you got pipeline infrastructure debt, you have data debt which is the decay of data. I mean concepts will erode just become completely wrong or they'll disappear. And the organizational debt, and I love the analogy you brought up like that punch card, you can only punch that card 20 times. You can only screw up 20 times after that, who knows? So the report didn't arrive on time? Punch card right there. And the other issue is that in data you can mask over debt with another query and I think part of it of the debt is that you want to not repeat yourself, just do it once and Looker was this whole approach, but when you actually look into that stuff, you would still see so much repetition. So it's like we don't really learn this stuff in data, we're not seeing it. And then kind of wrapping up, are there other ways or better ways of doing modeling? Think one of the stuff is look at the different methodologies, things there are, we can and should do better when you look at different methodologies, looking at different modeling patterns and reuse existing patterns and figure out if you can't reuse them as is, you can be inspired to go take parts of it. At the end of the day, just be pragmatic about it. Bottom line, right tools for the right job.
Joe Reis: Love it.
Juan: Anything we missed? How do we do?
Joe Reis: Fantastic guys. It's like you've done this before for four years now.
Juan: Part of our fourth one. Hey, we took a month off.
Tim: And Joe, you're the perfect guest to have here twice because I think you've got a wealth of knowledge and we're just so glad you could join us today.
Joe Reis: Anytime guys.
Juan: Joe, take us home quickly. Advice about data, about modeling, about life. Who else should be invite next and any new resources that you want to share, that you follow that people should follow?
Joe Reis: Who should you invite next? I think Ethan Aaron and Kevin Wu and those guys would be amazing to have on.
Juan: Ethan's coming soon.
Joe Reis: Cool. Yeah. Yeah, there's a lot of people and yeah, I'm sure I'll have some more. I'm about to start another world tour in a few weeks, so I'm sure I'll be able to recommend a lot more people that I want to recommend people off the beaten path. I think there's like the LinkedIn kind of filter bubble, but there's a lot of interesting people I meet who you probably haven't heard of before. A guy, John Giles, would be fascinating for you to have on the show. His last name's G- I- L- E- S. Yes, he's in Australia. He's amazing. He's like Yoda when it comes to data modeling. You would love him. I think he lives in rural Australia as well, so he probably literally lives in inaudible, but people like that are cool. Larry Burns is another guy I would have on, so yeah, he's dope.
Juan: By the way, why is there so much modeling in Australia? That's another question I have.
Joe Reis: I don't know. It's a weird thing. Yeah.
Juan: Larry Payne.
Joe Reis: I'm looking at several books. It's like, yeah, Graham Simpson there. There's all these books I have from Australians. I don't know what it is in the outback or something. Must be inaudible about modeling.
Juan: Yeah. And where can people find you? You're going off and going to your next events.
Joe Reis: Yeah, yeah. So I'll be, you can find me on Substack. I'm at Joe Reis at substack.com. You can find me on LinkedIn, just add me there. Apart from that, yeah, this upcoming I'll be in Australia, Europe, Middle East, maybe India, maybe again Canada, and also on tour with dbt. dbt and Joe Reis Road Show hitting the city near you. Next one's in... was it Atlanta? On the 10th I believe. And then Seattle and I think a few other cities as well. So yep.
Juan: Joe, thank you so much.
Joe Reis: Thank you guys.
Juan: It's been a pleasure. Next week we are going to be... I'm going to be at CDOIQ in Boston and-
Joe Reis: Nice.
Juan: Tom Redman, who's the data doc. We're going to have him live right before we do on this no- BS dinner. If you happen to be in Boston, you want to get our... I think we're actually booked, so, but hey, do you want to join our dinner? Let me know. Maybe the seat opens up.
Joe Reis: That's at MIT, right? CDOIQ.
Juan: Yeah, well I think it's fine inaudible officially anymore, but anyways.
Joe Reis: Okay, good one.
Juan: It's awesome event. All right. Joe, thank you so much.
Joe Reis: All right. Cool.
Juan: Inaudible a little bit over.
Joe Reis: See you guys.
Juan: Inaudible.
Joe Reis: No worries.
Tim: Cheers, Joe.
Joe Reis: All right.
Tim: Cheers.
Joe Reis: Thanks everybody.
DESCRIPTION
In this episode, Joe Reis, best-selling author of Fundamentals of Data Engineering explains why data modeling and semantics need to take the center stage for AI to truly succeed.
Today's Host
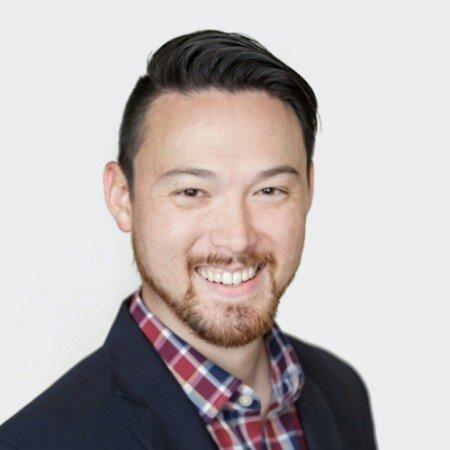
Tim Gasper
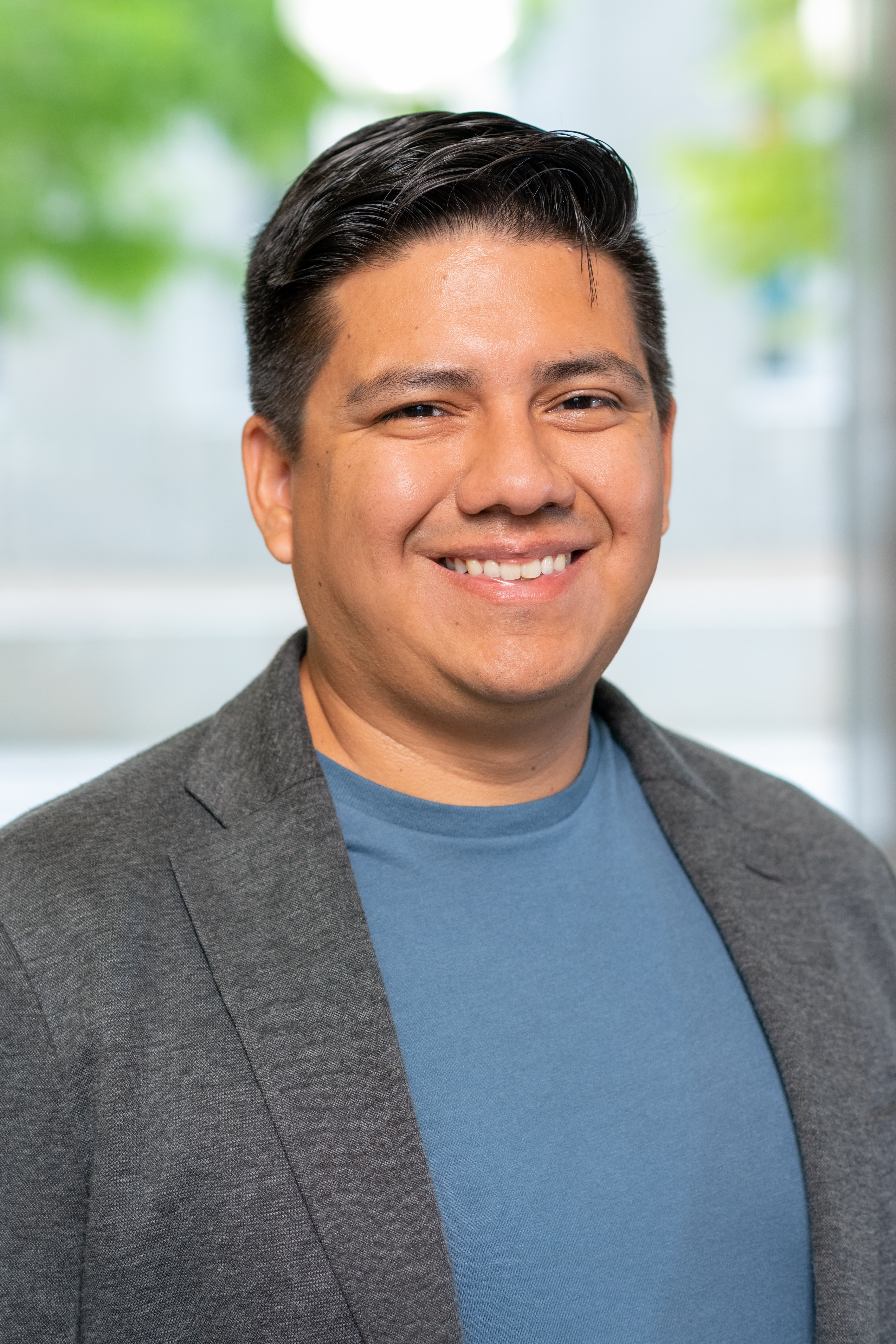
Juan Sequeda
Today's Guests
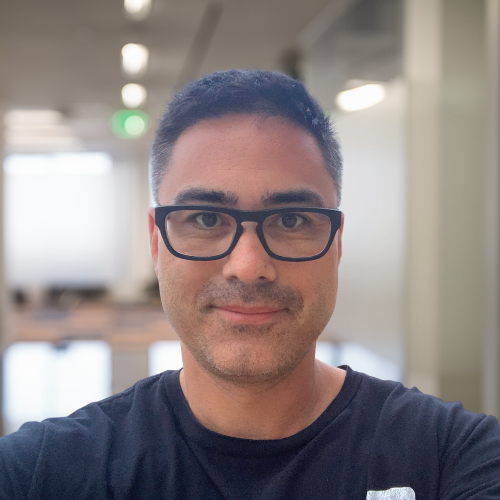