Are data teams keeping up with AI teams? w/ Theresa Kushner
- 0.5
- 1
- 1.25
- 1.5
- 1.75
- 2
Speaker 1: This is Catalog& Cocktails presented by data.world.
Tim Gasper: Hello, everyone. Welcome to Catalog & Cocktails presented by data. world, the data catalog for leveraging agile data governance to give power to people and data. We're coming to you live from Austin, Texas, and somewhere else. It's an honest, no BS, non- salesy conversation about enterprise data management with tasty beverages in hand. I'm Tim Gasper, longtime data nerd and product guy at data. world, joined by Juan.
Juan Sequeda: Hey, I'm Juan Sequeda, principle scientist here at data. world. As always, it's a pleasure. It's middle of the week, towards the end of the day, and it's our time to take a break and chat about data and AI. We have our guest today, which is Theresa Kushner, who is the head of North American Innovation Center at NTT Data. Theresa, how are you doing?
Theresa Kushner: Oh, I'm fine. Thank you, guys. It's so great to be here with you.
Tim Gasper: So, glad to have you.
Juan Sequeda: Yeah, thank you so much for joining us. I think you have so much vast experience and be able to go talk a lot about data and AI here today. But first, let's kick it off. So, what are we drinking and what are we toasting for today? Theresa, do you want to kick us off?
Theresa Kushner: I am at work. So, unfortunately, I'm not toasting. I'm not drinking anything, but I'm toasting to the success of this show today. How about that?
Tim Gasper: Oh, awesome.
Juan Sequeda: Excellent. Yeah, no, we've had a little bit of some glitches at the moment. I think we're okay. We'll see how this goes today.
Theresa Kushner: Good.
Juan Sequeda: How about you, Tim?
Tim Gasper: I am today drinking a Cayman Jack margarita. It comes in a highly portable form, but it is a cocktail which I thought was appropriate. I will toast to hanging out with awesome data people, which we've got two awesome data people here and one person who's drinking margaritas.
Juan Sequeda: All right. Well, actually, I have a real cocktail here today. It's called the Beat the Jet Lag. It's a beet infused Don Julio with dry curaçao, lime juice, agave, and some Lagavulin spritz. I'm actually getting off a plane. I'm at the United Polaris Lounge right now. So, we do this live, real live. So, I'm cheering for I get the travel and get to pause and go find a place to go do the podcast. So, here we are. Cheers.
Tim Gasper: Cheers.
Theresa Kushner: Incredible.
Juan Sequeda: Well, timely warmup question, which is planes, trains, and automobiles. What's your favorite way to travel and why?
Theresa Kushner: My favorite way to travel is automobile simply because you get to see everything and you can stop when you want to. You don't have to pay attention to where you are or get your seat up to go to the restroom. You can just stop and do your thing. I love that part.
Juan Sequeda: How about you, Tim?
Theresa Kushner: I'm waiting for my self- driving car because I figure that'll be an even better way to get around.
Tim Gasper: Yeah, I can't wait for that. That'll be fun. I do like the freedom that comes with driving in an automobile already. You can control your own destiny there. I really think trains are cool. So, I think that's my preferred way to travel, but mostly because I like the idea of it. I wish we had really good trains here in America because I would use that all the time. When I was in Europe and also when I was in Asia, using trains is a great way to travel and can be really fast. So, I like trains.
Juan Sequeda: Yeah, so because I'll have to take the other one. So, I'm going to take planes. I mean I am a big avid flyer or traveler. I've a United 1K. I've already flown a million miles on United. I'm literally doing this from the airport right now. So, I think it's fascinating is that you sit in that chair in some metal tube for 10 hours and suddenly you're in a different world in a different environment. That's just fascinating. So, I was in Munich yesterday or this morning and now I'm here.
Tim Gasper: That's modern teleportation, right?
Juan Sequeda: There we go. All right. Let's kick it off with our honest, no BS discussion. All right. Theresa, honest, no BS, are the data teams keeping up with the AI teams?
Theresa Kushner: Generally, I would say they're struggling a little bit. The data teams are trying to get the data in shape for the AI guys to use, but there's a lot of work that has to be done from a data engineering perspective to supply that to an AI data scientist. That's why most of the data scientists say that 70% of their time is spent on getting the data right. And then when the data scientists take over, they create feature sets that are brand new sets of data that somebody in the organization has to manage. So, they need each other, but it's a contentious relationship often if data engineers are assigned to data scientists.
Juan Sequeda: That last part that you said that when the data scientists get the data, which will come from the data teams and then they start generating these feature sets, which themselves are other data sets, that's something that the data teams are not managing very well. So, you may have, for example, some governance set up for the data teams and the data products that may come out, but then the AI teams are doing things too. Are they following the same guidelines or not? I think that's very important thing to consider, because then we're not being aligned within the organization.
Theresa Kushner: Yeah, that's a very big consideration, especially from a governance perspective. I was talking to someone this morning about the fact that when we look at governance overall, there's a brand new way of managing data and we're looking at data as a product. That's what all the new organizations are doing is, " How can I look at my data as a product? What kinds of things do I have to do to protect it? What kinds of things do I have to do to manage it or catalog it?" But in that world, there's got to be an exchange somewhere and a governance process that manages the data so that I can know if I'm in marketing, how much my data's worth to sales if they want to utilize it and what the value is of my information. So, we've got some contentious things from a governance perspective set up. If you're managing your own product and your own organization as a data set of some sort, then you have that control where you can actually say who gets to use your feature sets and who doesn't. So, you've got a contained environment, which is what we're heading for with the Web 3. 0 and some of the other kinds of innovations that are coming down the pike.
Tim Gasper: Interesting. Do you find that data teams and AI teams are often embedded with each other, or are you finding that they tend to be very separate from each other? If they're separate, are they helping each other or are they avoiding each other? What's the relationship between these teams?
Theresa Kushner: Yeah, it just depends on what the culture is and the business that you go into. If you've got someone who's got a strong data culture and everybody in the organization understands the value of the data and they're helping to manage it, then you don't find that contentious relationship at all. I've managed analytics teams a lot in my life. Usually, analytics teams are set to take advantage of whatever has been put together from a data perspective. Usually, the data resides in an IT organization, because AI is created by applications and applications belong to IT. So, that's where most of the data sources end up. Analytics teams don't often end up there. Sometimes they do, sometimes they don't. They often end up in finance or in operations, where they can use the information to be more valuable to the business. So, that sets up this divide and it shouldn't be a divide. There should be a coming together of those two sources for sure.
Tim Gasper: Interesting. Yeah, so there actually is in some cases structural reason why these groups are in different parts of the organization. If your company is not good at making those different groups work together or has a culture that's against that, then that can cause some problems. Interesting. Okay.
Juan Sequeda: Yeah, so one thing that you just said, which I find very insightful, is that the AI teams are the ones who are consuming the data and then also this happens a lot of the analytics, but they're not used to being the ones who are producing the data. But we're now starting to see a shift of like, " Well, hey, if the applications are the one producing the data and the other teams are consuming them," this is not just a divide like, " Oh, you're a team that produces data, go manage that." You're a team that consumes data. Here's how you consume it. We need to have these ways of being able to go say, " Hey, you're actually producing and consuming at the same time or consuming and then producing based on what you consumed." What are your thoughts and guidelines on how we should best define, we call it, best practices, approaches for teams who are only producing data? I don't know if that's actually the case, but teams who are only consuming the data and teams who are consuming and both producing the data.
Theresa Kushner: Yeah, it's a very interesting conundrum that we are in right now because you're absolutely right. The AI teams produce data because they do produce feature sets. They also produce algorithms that go into all kinds of applications. So, that becomes almost a data set itself. You've heard this concept about data is the new code. In a way, that's exactly what we're talking about. So, now, all of a sudden, you have people that have got to manage all different kinds of data and it's not just data that is coming at them fast like streaming data or IoT data. So, it's not those kinds of types. We haven't even talked about that yet. We're talking about normal ordinary data. How do they manage that in an organizational construct? They manage it with the IT organizations because that's where they need to be, but they also need to be able to manage it with a data structure and a data organization. I've been around you guys long enough to know that you predominantly sell or try to sell in to the chief data officers, but I'm sure you've noticed that chief data officer...
Tim Gasper: I think we lost Teresa there for a second.
Juan Sequeda: Theresa, you're back. You're just saying that you're selling to the chief data officer.
Theresa Kushner: So, the chief data officer, but the chief data officer has required this new thing called analytics. So, they've got a chief data and analytics officer. What that does is that it puts all of your data in one place so that you can begin to manage what you need to manage from both a data and an AI and analytics perspective. So, I think that's where you see a lot of these coming together. Again though, I always hesitate to tell people this is that when you give the responsibility of data to one organization within a company, everyone else says it's not my problem. That's like when you give quality control to a quality organization, quality doesn't become anybody's problem but those guys. I think that that's what we're seeing is that a lot of the analytics and a lot of the data quality and a lot of the data problems that go to the chief data and analytics officer, they stop there. So, it's got to be an integrated organization. That's why I'm excited about having data products, because data products puts a dispersed organization out to each of finance or to marketing or to HR and it gives them the right to control their end in the way that they think is important. It gives them the right to secure it. It gives them the right to have it used in a certain way. So, from that perspective, that's also where you guys come in, because a lot of what happens with those organizations is the first thing they have to do is catalog and manage their data, get it in shape so that they can be able to sell it or to be able to process it in other organizations. So, there's a lot of opportunity for you in those spaces.
Tim Gasper: Interesting. So, just to go back a little bit to this data products conversation that you're bringing up and that's a topic that comes up quite often on the show. You had mentioned that gives the opportunity for the group to have more ownership and control around that. Are you thinking about that in terms of the data products that are coming more from the different parts of the business that they can then publish a data product into this marketplace or things like that?
Theresa Kushner: I guess the best example that I have is that in working in a lot of organizations, HR data is pretty secured data. To get HR data into a sales organization or into a finance organization, it usually takes an act of God or the CEO, whatever. It's not one of those things that people just make available, but if HR can make their data available in a product way, a productized way, then you have a way as a sales organization to purchase that data and use it in the way that would create value for your organization. That way, HR can feel better about securing it and making it part of their product set. So, they can do the things that keep their data up to date. They keep it accurate. They keep it moving within the organization.
Juan Sequeda: So, you said something key here, which is purchasing the data. So, couple things. I want to talk about this purchasing the data. How are you thinking about chargeback models around this? I want to expand on that. So, again, I want to expand also on the CDO versus the CDAO. What is the two differentiation around this? Because I mean putting the analytics in there, there's a difference in there, but I wanted to continue on that thread. The third thread is something you said about data as code for AI teams, right? So, I'll let you continue. This is fascinating discussion.
Theresa Kushner: Oh, goodness. I don't even remember what any of those were.
Juan Sequeda: So, let's do purchasing data. Expand on your thoughts on purchasing data.
Theresa Kushner: Okay. First of all, let's look at what we have to purchase. Purchasing data starts with, first of all, you have to value it. You have to set a value on what your data is. I'm not talking about selling your side to the company. I'm talking about the value managed between organizations. So, you can value your data in any way that you have to, but you've got to get it straight. You've got to have the metadata there. You've got to know what the value is, so the people that you're going to make it available too. There has to be some exchange. In some of the organizations who I've seen this work well, you have an exchange that is a centralized corporate function that actually manages what people can put in. So, they control what the cost might be or how it might be worked between organizations. Sort of like the stock exchange does in a way, but it's an exchange. I think that's probably the best answer to it. It also gives you a place to manage governance a little bit more effectively. You get a centralized organization that's managing the exchange of data across the organization. That's a very helpful thing.
Juan Sequeda: I like this comparison with the stock exchange. This makes sense. Also, I think it's some bar, right? Because you're not going to put all data in there. The data that is going to go into that exchange is data that has well defined value that other people are already convinced, right? It's almost like you're going to do an initial public offering for you.
Theresa Kushner: Exactly. Yeah.
Juan Sequeda: This is a fascinating point. I did not think we're going to get here today. I love this.
Tim Gasper: Well, the data product's analogy ties in well as well, because to put something on the exchange, you need to make that product available. It has to meet certain standards and things like that.
Theresa Kushner: It very quickly tells you where you should be putting attention to the data quality. If your product is not moving in that data exchange, if nobody really wants to use it, why are you putting it up there? Don't you have a different process for managing it than you might have for something that's more valuable? In actuality, the feature sets that get created in an AI organization are often some of the most valuable data sets that could be made available in an exchange.
Tim Gasper: That's interesting. I think there's a lot of our listeners that may not be as fully versed in some of the things that are going on in the AI space. So, I'm curious if you could go into a little bit more detail just for folks that are looking to understand a little bit more. What are sometimes these feature sets that are coming out of AI teams and things like that? Why is that a useful data set?
Theresa Kushner: Well, for example, I might have an application that keeps information about the square footage of houses that I'm selling if it's a real estate application, but I also keep the how much those houses sell for in a different set. So, if I'm creating something in an artificial intelligence algorithm, where I really want to have the cost per square footage managed and it's not a field in any data that I have. I create a feature set. Now, all of a sudden, I have a brand new data element that can enter into my algorithm, enter into my analysis of some sort. That's what I'm talking about feature sets. It's that creation of those data elements that come together that may not be in your data in a native way. It's got to be something that has to be created.
Tim Gasper: Right. Okay. So, now you've got these new fields that can be leveraged that are augmenting your data here. In some cases, certain AI applications may be creating many hundreds, many thousands more of these types of different observations and fields.
Juan Sequeda: This is a fantastic point, because you'd think that in this exchange that you could have, what are the data products people would be interested in are the ones that would augment the data I have. Those are the ones that could probably be traded the most. Those are probably not things that are specific to my company, not the transactional type of data that's going to be used for analytics, but it's other external things.
Theresa Kushner: It could be.
Juan Sequeda: Yeah. So, that's a very interesting point to go do that. I mean to try to find what has the most value, defining value here.
Theresa Kushner: The exchange too can be used for external data as well if you have external data. Because if you've got an exchange set up, then you've got a way to manage who's using it, how much it's valued at when it's used, how often it's used. You've got information about that data that you wouldn't necessarily have otherwise.
Juan Sequeda: This is great. So, let's go on the topic of the CDO versus the CDAO. How are you seeing this today? Because from my perspective, CDO is what you hear the most and not as much CDAO. So, please make the definitions of these two and how they compare.
Theresa Kushner: Yeah, in organizations where data is really, really important, you get a CDO and the CDO understands what to do. I just read an article today about the fact that we're not hiring CDOs that understand the business. For the most part, CDOs are getting hired as technical people, people who grew up in the technical side of the house. CDOs need to be people who understand the value of data. That doesn't necessarily mean you have to understand the value of data infrastructure. It means you understand the value of the data that you have. So, I think that what happened a couple of years ago is that we saw the rise of artificial intelligence. Every CEO in the world, everyone I talked to, we got to have an AI team. Well, what does that mean and why do you have to have them? Because they needed AI, they thought, " Oh, well, AI and data, that goes together. Let's put analytics into the chief data officer's organization." Let's face it, most of the time, managing data is not a very sexy job. I got to tell you. It's hard. It is just the most thankless job in the entire world. I tell you for 25, I had that thankless job. So, it's not one of those things that's just really everybody wants to go into, but analytics, everybody does. Because analytics drive revenue. It drives cost savings. It drives all the things that executives in the company like and understand. So, now you have the worst job in the company, data, coupled with the best job in the company and you've got an alright job, but one or the other, it's not the same thing.
Juan Sequeda: I love it.
Tim Gasper: This is a perfect, honest, no BS take right there. Juan, this is perfect. I love this. I mean you see organizations whether they focus on the data and they get too much into the weeds too. They lack all that business literacy to understand. People outside of their bubble doesn't know, " Why are you doing that? I don't see the value of that." They're like, "Oh, but we need all these things." I don't understand that. But the moment they do analytics, that's how they connect more. But how are you successful is if you know the business.
Theresa Kushner: Exactly. Every company I've ever worked for where they told me I needed to go do master data, the next thing I would tell them is, " When I get this all together, what are you going to do with it?" Because the interesting point is that nobody cares about the data and how it's cataloged or managed. That's like, " How do you care about your library?" Are all of your books in alphabetical order in your personal library? Yeah, probably not. If they were, what value would that bring you? It would save you X number of minutes a day because you know exactly where this one volume is. But executives don't understand that. What they do understand is the use of that data once it's been cataloged and managed and available for use. It's that analytics side that captures the attention. So, never take a data job without taking the analytics job too.
Tim Gasper: It sounds like you're pro keeping them together.
Theresa Kushner: That's my recommendation.
Juan Sequeda: No, so this is a good point. Should there be a CDO or should there be a CDAO?
Theresa Kushner: You need to put them together, I think. Yeah, it needs to be the chief data and analytics officer.
Juan Sequeda: So, are you predicting that the titles of CDOs are going to go down and we're going to see CDAOs increasing?
Theresa Kushner: Actually, the article I was reading says that's already beginning to happen. That's beginning to happen. I think it's like 70% of corporations that were in this survey have CDOs, but they're just now beginning to move them to see CDAOs. That's where the attention we'll get, because that's the point at which you can prove that your data's valuable is with the analytics that you do with it.
Tim Gasper: That's interesting. I know that at data. world, we work with a lot of different roles. We work with a lot of CEOs. We work a lot of CDAOs. It is interesting to see when you're working with somebody who's more of a CDAO who has more analytics in their role as well, just how they're approaching some problems differently. Whereas a CDO may get pulled more into like, " We got to do our master data management initiative or whatever." You see CDAOs often getting pulled more into, " Hey, we've got some a business problem. We know that data is the answer to solve it. I'm tasked with making sure that we can solve that problem," right?
Theresa Kushner: Yup. Exactly. They have more of an urgency sometimes than a CDO does. A CDO knows that he's in it for the long haul. It's not a job that you can do in a year or six months. It's a job that takes years to do if you're trying to clean up someone's data and make it available to everyone in a way that's acceptable. The analytics officer is out there to generate things immediately. They've been hired to bring in and use that data as quickly as possible. That's why when you mix them together, you start to get a good feel in the middle.
Juan Sequeda: So, if you do mix them together, then you are bringing in what I call the efficiency and resilience. So, we need to go solve problems today, but let's make sure that we're building that infrastructure. We're building that muscle on creating data products, focus on the data side. The technical thing about the analytics is what's connecting it to the business. So, another thing that you came up and I'd like to expand is data is code. Can you expand on that please?
Theresa Kushner: Well, I think that what we're seeing a lot of is in the AI world, data makes AI work. Without it, there is no AI. It's all predictive patterns. In these kinds of environments, all of a sudden, what you do with AI in an algorithm, all the data that's used is the code that is being managed. I give the example is that an entity in the innovation center, we do a lot of things with what we call virtual humans. In other words, responsive avatars. The data that comes in for those avatars is being pulled from a lot of different places, not just information that we want the avatar to be able to answer a question with, but the avatars are reading your facial expressions and deciding how they should respond to you. That is becoming part of the entire makeup of what we look at from a code perspective for that avatar. So, that data becomes part of what is actually fed out into the avatar itself, into the vision that you see on the screen. So, data is code. There's a lot more behind that. I'm not technical enough to be able to tell you all of it, but that's one of the things that we're looking forward to in the future. When you look at the Web 3. 0 and what we're doing with that, the community of a Web 3. 0 environment is to have the data be part of everything that works in that. We're going to protect that data. We're going to be able to manage that data within that community. So, making it part of the code is part of what you do. The other thing is that with generative AI, we're not going to be generating code very much longer anyway. The AI algorithms themselves are going to start to generate the code. That's going to make it very different. I mean, we already do that. We generate art with AI. We generate music with AI. Oh, my favorite, we generate white papers with AI. That's my favorite, because I could write a white paper in about 20 minutes with an AI generating capability.
Juan Sequeda: Yeah, I just saw yesterday, a couple days ago, another of these language models, they took all the scientific papers and then they're like, "Well, now, you can just make summaries, tutorials about the latest bits of science." Now that stuff is not always working very well, but that's what we're heading towards, so for sure. So, then what are your suggestions for companies and organizations that have the data teams and the AI teams, the CDOs, separated from the AI analytics? What's your message to them right now?
Theresa Kushner: Oh, where they have everything separated out? You can do that if you've got collaborative people. We've got Republicans and Democrats in Congress. They've got to be able to work together. I'm sure really a big challenge. But it doesn't matter where you put it. You've just got to make sure that the systems allow you to talk together and you're going after the same things. I think the thing that I would look at is people operate on directives that they are given and what their KPIs are, because that's how they usually get paid. So, manage your KPIs effectively for data and for the analytics team. Manage them together so that they have the same goals to go after. That would be my advice. Whether you've got them split up or you've got them in the same organization, look at what you're managing with them against.
Tim Gasper: Interesting.
Juan Sequeda: That's a very key one because it pisses me off, with all due respect when I say this, when you see people say, " Well, no, data is everywhere, so it's super hard to go put the ROI on our data that we're doing." I'm like, " That's crap. That's just an excuse. You need to be able to go to define and understand how you're providing that value." Because if you're not, guess what? Everybody in the organization is accountable. Everybody. Are you on some magic high horse or whatever that you can't know? Of course, if you're not able to go do that, then you're in the wrong place and actually, you're not being set up for success. So, what I like is how you're saying is all of these teams, the data analytics teams, they should have shared KPIs going forward and they both need to understand where the business is going and align this. That's a very key point right there.
Theresa Kushner: And alignment. Yeah. The thing is data is so important. It's the blood of the body of a corporation. It makes things happen in the company. But for years, it's been something that's been neglected. It's an after product of an application or an after product of I'm pulling everything together, I'm putting it in one space. We don't treat it right and it needs to be broader accepted. It's that same concept of quality. If I give quality to someone else, that's their job, which means I don't have to have to do any quality about my work, because it's your job. You got to check it out. You got to do all the QA to make it possible. We've learned a long time ago that doesn't work. You've got to get quality to everyone. So, everyone manages data. Everyone manages data. It used to just irritate the bejesus out of me for sales guys to say... When I was trying to create capabilities from an analytics perspective and yet sales guys could not be bothered to enter information into Salesforce. com because that took too long or you're going to stop my sale or something without the understanding that what they put into that application could be used to develop more clients for them, it could be used to sell more products for them. It was such shortsightedness because my goal was to sell product. Now, if I had a goal that was a KPI from sales that also said, " I've got to maintain my client information at a certain level," wouldn't that be nice?
Juan Sequeda: This is so spot on, what you just said right here. I mean everybody needs to be managing data and everybody needs to take responsibility of their data and the quality of the data. With just the example you just gave, hey, sales people in Salesforce, go add it in, because it's going to have an implication. I mean, you are right. They're the person who is producing the data. The data's being produced by human and a machine right here. So, we got to take ownership about that stuff. Reminds me to bring this up. I know we're the non- salesy podcast, but this is a really great example is one of the folks we work with, the CEO has a mandate on quality of data, CEO mandate. They said the 20% of the bonus of everybody in the company is tied to the data quality. What they did was identify very specific business use cases that they tie it to. The example I was given is there's a height of warehouse. What happens if I don't have the correct height of a warehouse? I'm leaving money on the table because I could have packed more boxes or I actually thought I could pack more and I can't. So, I'm into trouble. But the goal there wasn't about, " Oh, let's go improve the quality of certain data elements." No, it was about creating that culture and I think this is a super important part about it. So, yeah, I really, really love this and it ties back to how we're actually providing value here within the data side and the analytics side.
Tim Gasper: Yeah, that's really powerful.
Theresa Kushner: Who do you guys service most? Do you service the CDO or the analytics officer?
Tim Gasper: I think that's a good question. I think we most often are seeing catalogs being adopted and brought on by the data office, the CDO. In some cases, the governance office, right? Data architecture in some cases. So, we're seeing it more that central data office of some form or fashion. In some cases, it may be analytics and things like that, but not as often as you might think, unless it is a unified function where it's more data and analytics together. At least that's what we've been seeing.
Juan Sequeda: I would actually argue that you see people coming in more from the technical side, right? Oh, we need catalogs to be able to go manage lineage or where does this data come from? A lot of more technical things or protections. The governance is to protect the data. And then on other side, there's like, " Well, no, we need the data to be found." So then there's folks who are placed to go do discovery data when you go search for data. So, those types of use cases we'll see are more about I need to expose the data to be able to use it more for analytics. So, we see both of those types of things. A trend that we're definitely seeing constantly is about the data products. I think everybody's talking about it and there's a handful of people who have very opinionated views about them. Tim and I have one. We have our data products who are opinioned about that. We're just figuring this out, but I think the principles of" Hey, there needs to have some responsibility, ownership around that stuff." What we're starting to hear more and more but little, still more of it is the value. Find the value to the products.
Theresa Kushner: I think the issue that we're going to have when we start to look at data products is that the skill sets for managing a data product are a little bit different than those for managing data. So, that's the issue that we're trying to solve is that you actually have to have a product manager in there. They have to understand what it is to create a product, sustain it, test it, make it available, understanding their market. It's a little different than just managing data. So, I think that's where we're going to hit a little bit of a stop.
Tim Gasper: I really like that you're bringing that up because I think that folks are underestimating that at the moment. I think they're excited about this idea of data products and they're like, " Oh, yeah, we should treat our data more like a product and start to build out some data products and identify them and things like that." There is definitely a mentality and an approach and a toolkit that product managers have if you just want to bring it from the software world around what you mentioned, testing. Another aspect that I like to bring up with folks is around the life cycle, right?
Theresa Kushner: Absolutely.
Tim Gasper: Usually, we just think, " Oh, yeah, you produced the data and there it is. It's sitting in the lake."
Theresa Kushner: That's it.
Tim Gasper: Well, maybe I should retire that product or is it deprecated? You think about the life cycle of it.
Theresa Kushner: Right. As a product manager, you have to think about the market too. What am I really doing with this? Do I need all of this? These are skill sets that people that manage data don't have an abundance. So, it's going to be a different world.
Tim Gasper: This is something where experience design is going to maybe start to become a thing. What are the user stories?
Juan Sequeda: We've been talking about data marketing. So, I mean apply marketing 101 principles to how we do data. Who are the users? I mean you go talk to these users. Where is the market? Where is it heading? Let's actually go promote it, right?
Theresa Kushner: Exactly.
Juan Sequeda: Let's go create ads for our data.
Theresa Kushner: That's right.
Juan Sequeda: Right, it's how people understand it. It's how people are using it today and give those examples, right? Because at the end, it goes back to the KPIs. Well, if this is actually producing value and if some group's already getting value out of this, you can get out of it too. So, let's proceed.
Theresa Kushner: Product versioning, things like that. Today, the data might represent certain thing, but tomorrow, it's going to be different. What are the versions? How do you keep that? How do you make sure your customers are still happy? It's all the things you learn about software management from a product perspective.
Juan Sequeda: It's what is product, but also it's I think the marketing side. I've been having these discussions. I encourage all their data teams out there, the data analytics teams to go talk to the folks in your marketing team. Tell them, " Hey, assume that the product that we sell is our internal data. How should we market that? I think that's something we're not considering. I mean, we're not even scratching the surface on that." There's so much to go do. It helps us also to get more integrated with an organization. Let's have everybody talking about this stuff.
Theresa Kushner: Right. Yeah. Oftentimes though, it comes back to that question of you can't really create a product around something that's not valuable. So, you have to determine what the value of the data is and that's where a lot of people get lost to begin with.
Juan Sequeda: Definitely. Definitely. So, before we head out to our lightning round, I'd love to wrap up with just your final takeaways that you would like to go. What is the message you want to give out to more of the teams out there, right? Because we discussed there's a missed opportunities by data engineers and data teams, but they need to go step up. So, if you have those data teams out there who are listening to you, what's your message to them to say how can they keep up with all the analytics and the AI efforts that are going on?
Theresa Kushner: One of the things I would say to the data teams, and I've seen this a lot, is that as data teams, we get really integrated. We want to go deep into what we do. We really want to understand if it's master data or if it's ETLs. We really want to understand that. I would encourage them to broaden it out. This is the time where it's important to understand the big picture first and to make sure they understand the entire landscape before they zero in on one part of the data supply chain, so to speak.
Juan Sequeda: So, people like to zoom in. It's time to zoom out and see the forest from the trees.
Theresa Kushner: Exactly. Exactly. It is time to do that. I've been a data lover for my entire career. So, anything in the world that is good for data has got to be good for the world. That's the way I look at it. If you can manage data well, you can manage the world well. We've learned that already. We learned it at COVID. We didn't have good data for COVID and we didn't manage it well. We don't have all the data we need for managing climate control. So, manage data well and the world takes care of it.
Tim Gasper: I love that. That's a great thing to recommend and to say there. Maybe one last set of takeaways before we move to our lightning round. For companies out there that are thinking about AI initiatives and how AI can make an impact, do you have any tips on folks trying to scrounge up funding for AI initiatives or what can be most impactful to focus on?
Theresa Kushner: Yup, I do. This is my favorite question. I always tell people who are looking to do AI teams, I always ask this question. What is it that is the biggest problem in your company that you think AI can solve? They don't necessarily understand what that big problem is, but they're willing to go solve it. So, if you can find a problem within the company that is something that they really care about and apply AI to it, that's how you get an AI program solved. What I've discovered over these years is that a lot of times, the biggest problems in the company can be solved with just plain, ordinary analytics. Not necessarily AI, but AI they think they need because it's the magic word. Quite frankly, Gartner's told us that AI includes analytics. It's the entire world. Now we're calling everything AI. So, I think that that's something that people need to look at is why would you really spend all of that money to put up an AI organization if you can do it with statistical analysis.
Juan Sequeda: Oh, this is perfect. I love this. Thank you for sharing this, because you got to be honest and no BS with yourselves, with everybody right now. Do you really need all this fancy AI or could you do it with our traditional BI reporting analytics tools? If you can do that, then what is the extra value they provide? Otherwise, you're trying to go use this fancy objects, fancy tool. You need to be honest and no BS please.
Tim Gasper: Apply AI for the problems that really should have AI applied.
Theresa Kushner: Yeah.
Juan Sequeda: Anyways, this has been an awesome conversation. Let's go to our lightning round, which is presented by data. world, a data catalog for successful cloud migration. I'm going to kick it off. So, are AI opportunities and companies underinvested at the moment or overinvested?
Theresa Kushner: I think they're underinvesting always in data. Everybody underinvests in data, because it's easy to skimp it. A little bit of overinvesting, I think, in setting up AI organizations that may not be useful.
Tim Gasper: Interesting. That's helpful. All right. Second question. You mentioned that CDAOs are a rising trend.
Theresa Kushner: Yes.
Tim Gasper: Should AI be part of that charter? Should it be C AAIO?
Theresa Kushner: Well, it's analytics. I just throw it all in there together. The A is AI and analytics. Yes, it should be.
Tim Gasper: Analytics and AI together.
Juan Sequeda: But didn't you just say that Gartner's saying that and that's confusing people because then they're pulling?
Theresa Kushner: It's confusing people. Yeah, they do.
Juan Sequeda: All right. So, A means analytics in AI but know which one you're using, which of the A's.
Theresa Kushner: Yeah. The problem with it is that artificial intelligence is not just one brand, no one variety. You've got NLP. You've got machine learning. You've got all these different things that AI is. So, it became the umbrella term for everything. So, for machine learning and for NLP and for statistical analysis, it became everything.
Tim Gasper: That's a very good point.
Juan Sequeda: Next question. So, we're now seeing these roles called AI engineers or machine learning engineers and we have data engineers. They continue to rise. Does that mean that the data scientist role is going away or is this just a rebranding of the data scientist role?
Theresa Kushner: Because we can't find data scientists all the time and because they're rare and they're expensive once you do find them, what they're trying to do is utilize as much of the data scientists as possible by creating machine learning engineers or data engineers to support the guys that are doing the statistical analysis, the guys that are running the codes. So, that's why you've got this machine learning because machine learning data engineers are going to be the ones that are going to end up managing the product of the feature sets. They're going to have to work those back into some organization of some sort. So, machine learning engineers are important. I have seen that. In fact, not too long ago, I had to do a job description for what a machine learning engineer is versus a data engineer.
Tim Gasper: Interesting. This is an interesting takeaway here, Juan. Augmenting the data scientists with more specialized roles since it's hard to find all these data scientists, right?
Theresa Kushner: Yeah. I was at MIT a couple of weeks ago and I heard one of the professors there say that and my lights going out here, by the year 2030, we would require about a million more data scientists than we have. So, I'm sorry, my lights. I'm in witness protection program.
Tim Gasper: Oh, no worries.
Juan Sequeda: Actually, this was funny because the exact same thing happened to our guest last week. His advice was you got to move around in your office.
Theresa Kushner: I'm going to move around.
Juan Sequeda: All right. Final question, Tim.
Tim Gasper: All right. So, our fourth question here. Right now, the dashboard report is the primary thing that people think of as the end result after you do a data analysis, right? It's like, " Oh, it's the dashboard." Is that going to change you think as AI expands and proliferates or do dashboards just get better, more trustworthy?
Theresa Kushner: I think what happens to dashboards is that instead of you having to go to them, they come to you. That the information a dashboard has is going to be so versatile that it comes to you, instead of you having to go to a dashboard. Now, there'll still be some things that you're going to want a dashboard for, because quite frankly, that's a way of looking at information. In some places, that's really helpful, because you can compare things side by side. But the data that you need in order to make decisions, you should be able to get anytime, any place as quickly as possible. That sometimes takes away from a dashboard, because the dashboard, you got to refresh it, you got to call it up. It's got to be there. So, I think dashboards just get better and I think the movement of the data is going to get better too.
Tim Gasper: Awesome. Yeah, better movement of data, better dashboards, and dashboards that find you. Maybe they anticipate the questions that you have.
Theresa Kushner: Exactly.
Juan Sequeda: We got to start putting this AI inside of the dashboard then. So, the same way you have the AI that's writing your white paper, then you're going to have your AI who's going to write up and tell you what the dashboard is.
Theresa Kushner: Exactly.
Juan Sequeda: That's analytics and AI together.
Theresa Kushner: That's it. That's it.
Juan Sequeda: That's the data's code. There we go. We circle it all together. But I mean honest, no BS here, that's not crazy. I mean, if you actually are able to understand what are the problems we are doing and you see different ways of representing data stuff, I can foresee that. Actually, I'm curious. I haven't seen any work or any tools or companies having automatic generation of dashboard or stuff.
Theresa Kushner: You can see that in places. You can see automatic generation of a dashboard, automatic generation and display of data. That happens in a lot of different places.
Juan Sequeda: All right. So, Tim, takeaways. TTT, Tim, take us away with takeaways.
Tim Gasper: Yeah. So, tons of awesome takeaways today. Thanks so much, Theresa, for joining us. So, we started off with generally data teams are struggling to keep up with the AI teams. That there's a lot of work that has to be done to support the AI teams, a lot of the data preparation work, data engineering work, so much so that even the AI engineers are having to do a bunch of time spent preparing it and managing the data. Not only are the AI engineers and the broader data teams needing to process the data, prepare the data for AI work, the AI work produces data. It produces these feature sets and these derived data sets that then become additional data that you want to leverage that can provide value to the company. This starts to tie into, and this will come through our takeaways a few other times here, this idea of the data product. Oh, these data products coming out of the AI teams and a product approach could be a better way to standardize. Because you asked that question of, " Are AI teams following the same standards and approaches as the other data teams?" Probably not by default. Are the AI and the data teams working together? It depends on the culture of the company. Many companies that have collaborative cultures, those teams probably work pretty well together, but in some cases, it might be a more contentious environment. You also mentioned sometimes you see these structural things, where actually, an analytics or an AI team may more often than not be actually embedded into a part of the business, whether it's a particular product area, finance, marketing, some other part of the business. Whereas the data team might be more centralized in a place like IT. So, structurally, that can maybe cause problems at times. The way to help people focus on creating data products. We also talked about purchasing data. Purchasing data can also be data products. Third- party data can be data products. A place where you can manage your data products pretty effectively is maybe this analogy of an exchange. It's like that stock exchange that you have, where it's a central corporate function where people can put in data products. They can be empowered to make their data available to others. You can get governance through that. It's like a stock exchange. If your data product's not being traded, then that's where maybe quality comes in and you need to do a better job of enhancing the quality so it gets traded more. So, I like that analogy. Teams that produce data, teams that consume data, and teams that produce and consume data, AI is something that can affect all of that. You mentioned this idea of data as code, where everybody really manages the data. Everyone has responsibility around the data and treating your data as code can be a form and a way to think about this from a conceptual standpoint. So much more, but Juan, over to you.
Juan Sequeda: Yeah, I love the whole discussion about the CDO and the CDAO, right? So, you said you're analyzing that CDOs have been hired that have more of a technical background. They're not businesspeople. Yes, the CDOs, they need to understand the value of the data, but that doesn't mean that they need to know all about the data infrastructure. So, it's this balance that needs to have. Let's be honest, managing data isn't always the sexiest job and not everybody wants to get into it, but analytics, that's the one everybody wants to get into it because that's the one that is directing cost savings and revenue. So, the CDAO has actually combined the worst and the best jobs together. The CDAO, this is a good trend that we're moving towards and they are in the position to make a bigger and a broader business impact. For organizations who have these two different teams separate, the data teams and AI teams, how do we get them to work together is with those KPIs, they need to be managed together for the different teams and we'll have them get focused, aligned with the business. We're going to see all the skill sets for managing data as different from managing data products. This is a very, very important takeaway here. For data products, we're thinking about you need to test it. Is it sustainable? You might make it available. You understand your market. What is the life cycle of the data? What does it need to be taken down? How do we do versioning? Who are the customers? What is the marketing behind these stuff, right? This is very different than just the pure data engineering side of the infrastructure. And then recommendations, data teams want to go very deep and understand the details, but you're encouraging them to broaden out and understand the big picture. If you can manage data well, you can manage the world well. I see somebody wrote a comment about manage data, manage the world. I like that one. And then finally, advice for folks looking into setting up their AI teams. Well, people will be like, " Well, what is the biggest problem that company faces? Let's go put AI on it." It's like we got to be careful, because sometimes those problems can be solved with statistical analysis. We really need to understand what are the problems that the company has today that truly can be solved best by AI and not by existing approaches. Theresa, how did we do? Anything we miss? Anything to add?
Theresa Kushner: No, that was great. That was great, guys. What a roundup. Amazing.
Juan Sequeda: Well, I mean we just round it up what you were talking about.
Tim Gasper: That was all you.
Juan Sequeda: That was all you.
Theresa Kushner: That was great.
Juan Sequeda: Okay, let's throw it back to you for three final questions. What's your advice, who should we invite next, and what resources do you follow? I mean, people, books, conferences, blogs, podcasts, whatever.
Theresa Kushner: Oh, my.
Juan Sequeda: So, what's your advice?
Theresa Kushner: What's my advice? I think I've given you a ton of it already. My advice is I don't think that data's going to get less important in an organization. It's going to get more. So, my advice is to start paying attention to it now if you're not. If you are already, pay more attention to it. Spend more money on it. Put your better people on it. Do things that make it different for you. That's my advice. Who should you invite next? I'm going to tell you. If you haven't already had her, you need to invite Maria Villar. Maria Villar is the Head of Data for SAP. She manages and teaches a masterclass in data. She's great. So, that might be someone that you want to talk to. What do I read? I read everything. So, I think the best solutions for today's problems come from understanding everything that's happening in the world, not just what's happening in the technical world. So, the technical world, I think that the newsletters that come across TechCrunch and some of those, I do that. So, I try and keep up with that, but then I read a lot of technical papers too. We're a member of the MIT organization, so I read a lot of research from MIT. But I think that from a data perspective, it's responding to the world in the shape that the world is in today. So, look to the world for your data and for your capabilities.
Juan Sequeda: This is a beautiful way of wrapping up this fantastic discussion we had today, Theresa. Thank you so, so much. Just a quick, next week is Thanksgiving in the United States. So, Tim and I, we're going to take a quick break. So, next week, we won't have any live show. After that, on November 30th, we're going to have Allison Sagraves, who's a former CDO of M& T Bank. She has been on an entire journey for, I think, 20 years at M& T Bank, closing out as a CDO. So, it'll be a fantastic discussion with her. And then after that, on December 7th, Tim and I are going to be live at DGIQ in Washington, D. C. We're going to be the closing event at the DGIQ conference with Catalog& Cocktails. If you're in Washington, D. C., let us know because we're going to be organizing some fun events.
Theresa Kushner: That's great.
Juan Sequeda: Yeah. And then with that, Theresa, thank you so much. This was a fantastic conversation.
Theresa Kushner: Sure. It was great. It was wonderful. You guys are great.
Tim Gasper: Cheers, Theresa.
Theresa Kushner: Cheers.
Juan Sequeda: Cheers.
Speaker 1: This is Catalog and Cocktails. A special thanks to data. world for supporting the show, Karli Burghoff for producing, John Mellons and Brian Jacob for the show music. Thank you to the entire Catalog& Cocktails campaign. Don't forget to subscribe, rate, and review wherever you listen to your podcast.
DESCRIPTION
You have to have a lot of data to get AI to work. But the data folks are not jumping on it as fast as they should.
So what happens when data teams aren’t up to speed, companies are hiring more data scientists than they are engineers, AND current data teams are focusing too much on biz reporting and not supporting AI?
This week on Catalog & Cocktails, join hosts Tim Gasper and Juan Sequeda as they chat with special guest, Theresa Kushner, Head of North America Innovation Center at NTT Data Services to discuss how the AI train is leaving the station and data teams can only run so fast.
Today's Host
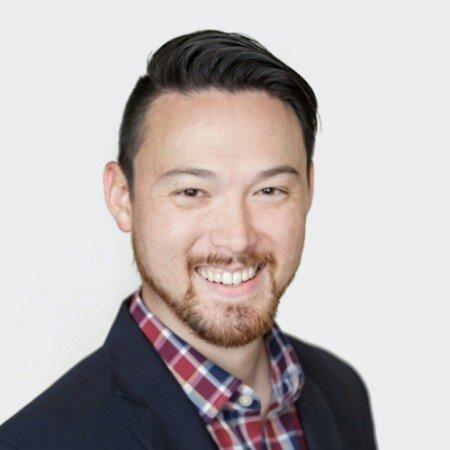
Tim Gasper
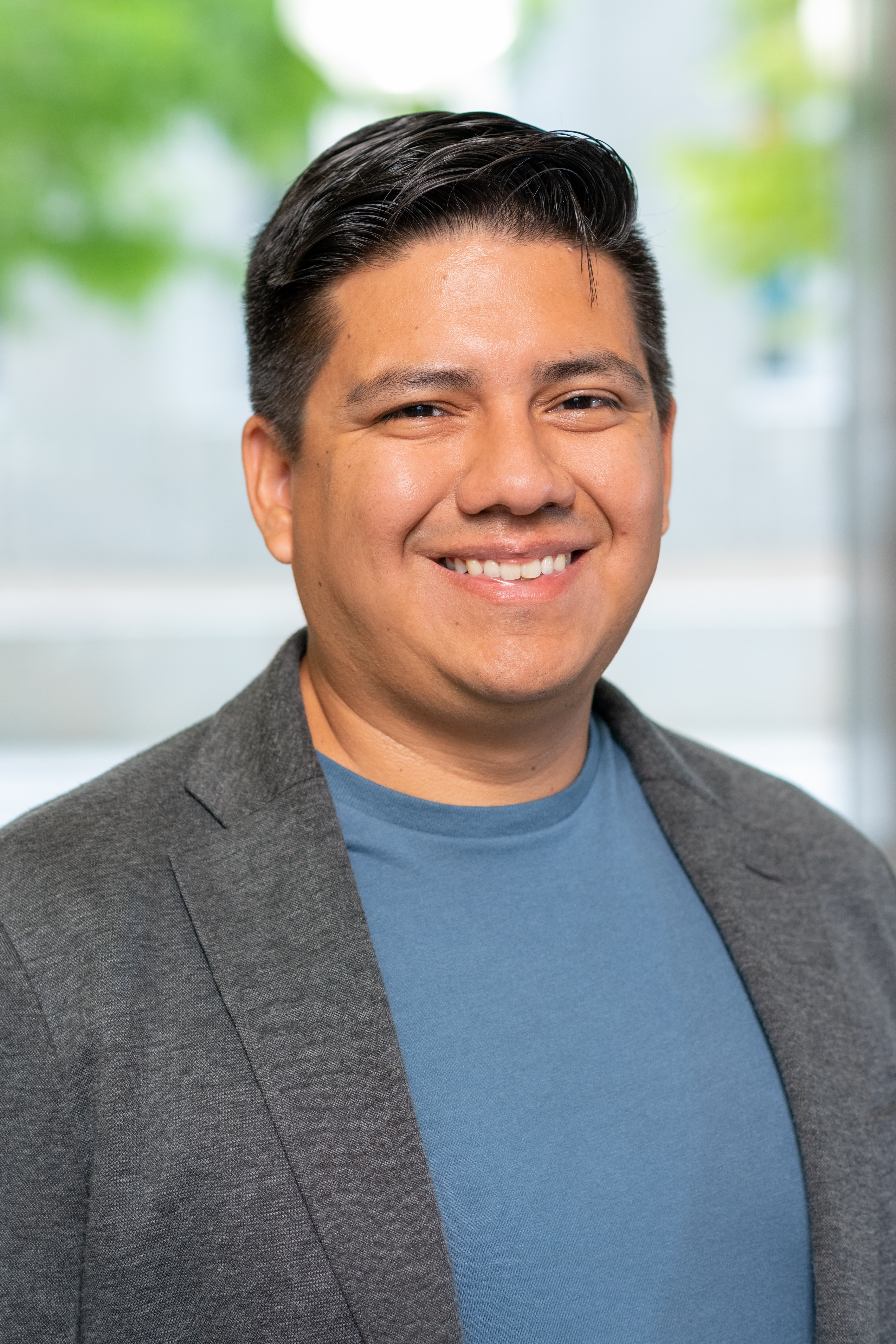
Juan Sequeda
Today's Guests
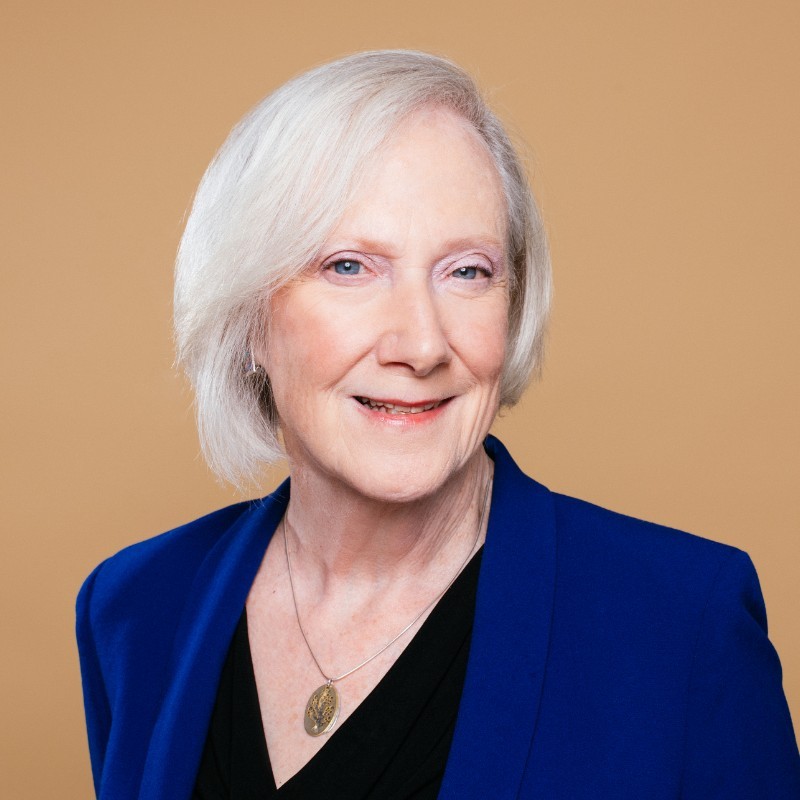