The Future of Data Catalogs w/ Ole Olesen-Bagneux
- 0.5
- 1
- 1.25
- 1.5
- 1.75
- 2
Speaker 1: This is Catalog& Cocktails presented by data. world.
Tim Gasper: Hello, hello, hello, everyone. Welcome to Catalog& Cocktails presented by data. world, the data catalog for leveraging agile data governance to power people and data. I'm coming to you live from Austin, Texas and you'll find out where else we're coming to you live from in just a moment. It's an honest, no- BS, non- salesy conversation about enterprise data management with tasty beverages in our hands. A shout out before we get started to data. world Summit, which is coming up on Thursday, September 22nd. Get registered, go to data. world, amazing guests. The theme is People plus Data, so check it out. I'm Tim Gasper, longtime data nerd and product guy at data. world, and this is Juan.
Juan Sequeda: Hey Tim, I'm Juan Sequeda, principle scientist at data. world, and we are here live 11:00 PM. We do this live. We are very happy to do this live. We are in Paris, Paris, France with my good friend, Ole. How are you doing, Ole?
Ole Olesen-Bagneux: I'm doing fine, Juan. Thank you. Thank you for having me here on Catalog& Cocktails.
Juan Sequeda: Ole, for those of who you don't know, because if you don't know about Ole and you listen to our podcast, you've been literally living underneath a rock, is the author of the upcoming O'Reilly book, Enterprise Data Catalog. I am so excited that we had the chance to meet here live and have this podcast face- to- face here, because I think we've been having the podcast already for the last two hours while at dinner. I think we're probably going to continue doing it after this, and tomorrow and so forth. I'm so happy, so let's kick it off with our tell and toast. What are we drinking? What are we toasting for? Hey Tim, what are you drinking in Austin right now at 4: 00 PM?
Tim Gasper: Well, right now 4: 00 PM, I'm drinking a ginger, raspberry and gin, so that's what I got going on. Something light and refreshing. What about y'all? What are you drinking? It looks like you got some fun stuff there.
Ole Olesen-Bagneux: Let's say burgundy, wine cultivated in a bio way, so very naturally. That's it.
Juan Sequeda: Yep. We're having some French wine because we're in France right now. I think we're just toasting because we are here in person. I think just a personal note here, we've been interacting I know since I think April, March and April. It's just been a really cool just getting to know each other and talking about metadata, and knowledge data, catalogs and everything. We finally get to meet after so many, many months talking. So I'm just super excited for that. Cheers.
Ole Olesen-Bagneux: Yeah. Yeah. Likewise, Juan.
Juan Sequeda: Yeah. Cheers, Tim.
Ole Olesen-Bagneux: Cheers, Tim.
Tim Gasper: I'm excited for your book, which is coming soon. That's going to be awesome.
Ole Olesen-Bagneux: Thank you, Tim. Thank you.
Juan Sequeda: Yeah. Just a quick reminder, Tim and I are both going to be next week in London at the Big Data London Conference. We're both going to be giving a talk on data products and we are going to be doing some special shows. I think on Wednesday and Thursday night, we're doing live shows of Catalog& Cocktails, so don't miss that. All right. Let's kick it off with our warm- up question today. So if a data catalog were a cocktail, a drink, a spirit, alcohol, whatever, what would it be?
Ole Olesen-Bagneux: That question is for me, right?
Juan Sequeda: For all of us. I got an answer. You go first.
Ole Olesen-Bagneux: I think that regardless of whether it was a wine or spirit, it wouldn't be something that you would blend with anything. It would be very simple and pure, and it would just age beautifully.
Juan Sequeda: That's a very powerful answer right there. Tim, what do you have to say?
Tim Gasper: That's actually a great articulation. I was actually going in some different directions. I was thinking well, maybe it's like a scotch, because at first maybe you're a little like,"Oh, I don't know if that's what I'm into." But then over time, as you mature your taste, you're like," Actually, this is what I need. This is my go- to." It becomes the center of a lot of stuff. That was the scotch argument. But then I was actually thinking maybe it's more like vodka for actually the opposite reason that you said, Ole. Because I was like," Well, vodka goes with everything, and metadata and catalog can go with everything."
Ole Olesen-Bagneux: That's also a very good answer, Tim.
Juan Sequeda: Well, I think as a catalog, there's going to be so many different facets of what you're going to be cataloging. I think wine for me, is something that just so many different facets. The geography around it, the types of grapes, the types of notes that you get on the smell, on the nose, and on the nose and the taste. I think it just make you want to be able to have a catalog that can deal with all that complexity and simplicity. That's why for me at the account, I'll relate that to wine.
Tim Gasper: That's a great answer. I like that. Wine is so faceted that you could benefit from a catalog of wines, which there are some sites that do a good job of that.
Juan Sequeda: For sure, for sure. Yeah. Actually, just for people to know, we've actually been creating our own data, that world data catalog of all the Catalog& Cocktails' episodes. Not just episodes of topics, but also all the cocktails and we got a couple surprises coming up, for sure.
Ole Olesen-Bagneux: Nice, nice.
Juan Sequeda: Yeah. All right, let's kick it off. We got so much to talk about and I know we talking for months so we can go so many places. All right, let's kick it off. Honest, no- BS, where the hell are data catalogs going?
Ole Olesen-Bagneux: Yeah, big question. I think to provide a short answer, that data catalogs should look much more at unstructured data and really become a search engine for companies. Like the way to find whatever you need, whatever knowledge you need to find in your organization.
Juan Sequeda: This is interesting. You're saying that the one thing is unstructured data.
Ole Olesen-Bagneux: Yeah.
Juan Sequeda: Okay. I think we covered the structured data part. The unstructured data, let me go pause there for a second. Are you already assuming that data catalogs today are already doing semi- structured data very well? Like cataloging all types of, I don't know, APIs and Kafkas, and JSON streams, and XML stuff that we may be having?
Ole Olesen-Bagneux: Yeah.
Juan Sequeda: Is that being addressed today or is there still room for improvement there?
Ole Olesen-Bagneux: Definitely room for improvement, but I also think that depends very much on the vendors.
Juan Sequeda: I see. One of the things that I find fascinating about all our conversations is that I personally, and I think Tim and I, we come from more of the computer science background. You have this non- traditional background from the technologists that we have, which is one, the information and library sciences. I think one of the things that I've really been passionate about through our discussion, is that you bring this different perspective. That when I go off and I chat with our customers, our prospects when I'm at the conferences, they're not thinking about it the way you are thinking about this. So in a nutshell, what is the library and information sciences world bringing to the table when it comes to data catalogs, that people are not thinking about right now?
Ole Olesen-Bagneux: Yeah. High level again, I think my perspective on data catalogs really is about providing a way to organize data and search for data, in a way that just increases the efficiency of data catalogs generally. I'm very occupied with search and the way you can search for data via a data catalog. I know this is perhaps not like there's been many discussions around accessing data and, of course, data mesh is a big topic. It goes up and then it goes down, and up again and down again. People are wondering whether or not this is the future or not. I think a separate discussion here really is search and how we search for data. I think that many of us have been raised with this search engine Google state of mind, where we could just type a word and find everything that we wanted. I still think that holds a lot of truth, but I think search, and we will discuss this later also, is something that needs to be considered in a more nuanced way, where we also look at different information needs. Sometimes we do not need one specific thing, we do not need one hit. We would need more hits and in some cases, we would need many, many, many hits to actually find the things that we want to find in a catalog.
Tim Gasper: In that sense, are you saying, Ole, that the search paradigm of Google and the search paradigm in a metadata sense or catalog for your data, is a little different than maybe when you say more hits, less hits? I sense more ability to what is your use case, depending on your use case to adjust how you approach it? Is that a good way of thinking of it?
Ole Olesen-Bagneux: Totally. I don't think that catalogs should move away from a Google paradigm. I think that it should just be supplemented with other ways of searching for data, that are perhaps not as fast and as precise, but opens to a bigger set of hits that just expresses another form of recall actually. I don't think that we should move away from this smooth search engine feeling. Not at all, very much the contrary. I think that we should keep this powerful way of searching, but supplement it.
Tim Gasper: Yeah. Interesting.
Juan Sequeda: One of the things that we've discussed a lot. When it comes to search, is what you've been calling and you have in your book, called the information retrieval query language. The first time we discussed this and it was really hard for me to understand because first of all, you used the word query language.
Ole Olesen-Bagneux: Yeah.
Juan Sequeda: As a computer scientist, I think about query language and was like sequel. I'm like," Wait, what do you mean by this?" My understanding after a lot of discussions like," Oh, this is really just an expressive, more advanced search capabilities of how you want to go."
Ole Olesen-Bagneux: Totally.
Juan Sequeda: Have Boolean types of queries and not just Boolean, but you want to be able to go group, and have negation in these things. We also discuss this whole expressive spectrum about search. I'm looking here at the comment that we have from a LinkedIn user who says like," Hey, why not think about a data catalog as an application that helps the users to find the most valuable data sets versus search for data?" Is this the same or is this different?
Ole Olesen-Bagneux: Searching for data, let me look at the question just a second. Why not think about a data catalog has an application that helps users to find the most valuable data versus search data. Yeah. The way I think of that question, is actually a way of assessing whether or not you're looking for the most precise hit in a universe of knowledge, or whether or not you're looking for a lot of potentially relevant hits. If you're looking for a precise hit, then I think search engine capability is just the way to go. But if you're looking for a recall of many potentially relevant hits, then you would be needing a more detailed query system or language. Is that what the question alludes to? I hope so. If not, please respond whoever it is.
Juan Sequeda: Well, I don't know. Tim, how do you perceive the situation about accessing what is inside of a catalog? This is when we start thinking about is data metadata? Your data is my metadata and so forth and how we're accessing it, because I know we have a different perspective. I want to hear what you think about this, Tim.
Tim Gasper: Yeah. No, totally. Then I'm curious, Juan, what you would say and then, Ole, what your thoughts on this. I think there's the paradigm that we've been forced into, and then I think there's the paradigm that I think we all wish that existed but doesn't, for obvious complexity reasons. I think the situation that we're forced into that can be fine, is I think we as a space, we segment metadata and we segment from data. Where it's very much, I want to search and I'm searching for knowledge, and I'm searching across concepts. I'm searching across my data sets and things like that, but it's all at that metadata level. Then we talk about this analogy of shopping for data, where now you've found the data product or the data asset you want to get access to. Now you're going to request access to it and then maybe once you have access to it. Then you can query it, and you can actually work with the data itself. I think that separation works and we've been forced into it because of security and things like that. But in an ideal world, ideally, metadata and data would be more intermixed. The facts that lie within the data and the metadata, it's all data. I think that's where search starts to get really interesting. I don't know, that's my take on where we're at versus where we're going. Curious about y'all's take on that.
Juan Sequeda: We've had this discussion about searching for data and searching in data.
Ole Olesen-Bagneux: Yeah.
Juan Sequeda: I think this is the distinction that you clearly make within your book. Expand on this.
Ole Olesen-Bagneux: Yeah, totally. Tim, in my view, I will expand on that, but there is actually also another point I want to get back to, Tim, in your comment. In my book, I rely very much on the fact that in library information science, a catalog is always conceptualized as a reference database. What this means is that it refers the users to data that resides outside of the database itself. Now, it's not a technical definition of a database. It's a conceptual understanding of a collection of data that actually refers people to sources outside itself. It's a natural understanding of catalogs in libraries, digitized catalogs, books in this way, in any collection of books or archives, or whatever you have. A catalog works very much in the same way. It shows different data sources, IT systems at animated data level. When you search for those things in a data catalog, you are referred outside the catalog and into the IT systems themselves. In that way, data catalogs can be conceptualized as reference databases. The consequence in terms of search here, is that you need to apply a different search language to effectively find everything you need in different scenarios. Now, we all have these simple search experiences with search engines, and those are also true for very good data catalogs. But in many professional contexts, we also need to retrieve data that is expressed in longer queries, so longer information needs. That is where you need this information, which we will query language. We can perhaps go a bit more into detail about that, but I want to touch upon another thing also, Tim, that you mentioned here and that is the shopping experience that you mentioned. I got to say I disagree with this notion of a shopping experience.
Tim Gasper: That's interesting. Go on.
Ole Olesen-Bagneux: Yeah. Can I?
Juan Sequeda: Yes. Go in and we'll see if we agree or disagree, go.
Ole Olesen-Bagneux: Yeah, totally. I don't want to like us to agree on everything. It's just that a shopping experience when you shop online, is something where you are presented with things that are more or less tempting to you that you want to consume. I don't at all oppose the idea of, for example, data as a product and all these things. I really do think that's a very relevant idea. But the challenges, I think, that we have with shopping experience when it comes to data catalogs, is that fundamentally, you will be needing to search for stuff that contradicts a shopping experience. You will be in need as a data governance person or a compliance person, or just a lawyer or whoever is searching in the data catalog. You will be in need of finding stuff that is complicated to find and not perhaps expressed very logically. The shopping experience tells you something else. It gives you offers that you would want to consume, that persuades you to consume certain stuff. There's nothing negative about that, but sometimes search just doesn't mimic a shopping experience.
Juan Sequeda: I think I agree with you and disagree with you on a couple of things. So one is, I think we're using the word search very generically here. I think it's important to understand the personas, but also what you also bring up is the information needs that those personas may have.
Ole Olesen-Bagneux: Yeah, totally.
Juan Sequeda: So if somebody has a very specific information need that they know that they're looking for something specifically, I would argue that they know what they want. Now there may be some serendipity of saying," Hey, you want this thing, but you know other people who had similar needs also bought or got this other thing, so you may want that too." But they started with something very specific and they found what they needed. Now, then there is another scenario where it's like," I don't know exactly what I need very specifically, but I kind of know." I'm not looking for that one specific thing that I'm putting in my shopping cart. I need to go and start navigating around these things. That's the thing that's the second scenario. Then a third scenario, which I would argue is a subset of that first one, which was I'm looking for a very specific thing, is I'm looking for a very specific complex specification. I need to go find data that has been done this, that has touched this, that hasn't been touched by this, that goes into this thing. You want a much more expressive way of searching that, not just by I'm looking for data about customers or orders, which is what I consider that first one. I think if you're searching for I need data about customers, then you find a bunch of stuff, you put it in the shopping cart and that's what I want. If you're searching for I don't even have the clear requirements, but I maybe even have a hypothesis or intuition, then you don't even know what you want to go, you're really just navigating. You're not even putting anything in the shopping cart. I agree and disagree with you. I don't know. I've been ranting here. Tim, what are your points, what are your thoughts?
Tim Gasper: I don't think I necessarily disagree with what you're saying. I think where my mind is going, is how shopping compliments a good search experience. And it's one potential modality of," Oh, I'm searching for a product." Which maybe if we translate that into data speak, maybe that's like a lot of data and a lot of your data assets aren't data products. When they are, then having a shopping experience can make sense if you don't yet have access to that data. Or if you've got different departments that have accounting practices around how data has to be accounted for and things like that, then maybe that's where the shopping experience comes in. I guess where my mind started to go was actually extending, Ole, your Google analogy. I was thinking about how Google has Google Shopping, where it now is indexing lots of different products out there. And if you're searching for something that feels shopping related, then you're going to get directed to the shopping experience, but it compliments the overall search flow.
Ole Olesen-Bagneux: Yeah. Yeah.
Tim Gasper: I don't know if I have any conclusions based on that, but I'm curious if that triggers anything from your perspective.
Ole Olesen-Bagneux: I think that's a very nice way of providing a little perspective, Tim. I really do. Let me tell me you a short story from back in the days, where I was just fresh out of university and in my first position. I would find stuff. I was working for this big pharmaceutical company in Denmark where I live in Copenhagen. It's called Novo Nordisk. I work in this information management department, and I could find a lot of stuff. During audit center's inspections, we were asked to retrieve stuff very, very fast. For example, I think I mentioned this to you over the phone, Juan, one time. Inspectors could ask," Okay. What was the pH value of this fermentation tank in the month of February in this specific site in Nova Nordisk? A fermentation tank in a specific site, in this company during the month of March in 1996?" That's a very, very precise information need. I need one hit to that answer, but there will never be a shopping experience around such a search because it's too detailed. But there needs to be a structure that facilitates the retrieval of that hit. I think just to compliment you here, Tim, that I think that below the search experience, there needs to be something that's a little more raw, but that is good enough so that we can find the stuff we need. That's very much the space that I'm arguing in data catalogs.
Tim Gasper: Yeah, that's interesting. Well, and this is an interesting segue to some other things that we've talked about in the past, as we were preparing for this episode today. Where you've done a lot of thought around how cataloging intersects and can be very well informed by traditional computer science, and information science, and even library science concepts. Can you talk a little bit about how those concepts relate and how they impact search and metadata in general?
Ole Olesen-Bagneux: Yeah. Yeah. So it's a big concept. They could go in many directions. We've already touched upon information retrieval query languages. If I should bring about some other concepts, it could for example, be ontology. When I started library information science, we designed ontologies all the time. I did an ontology of a zoo, actually. It's a pretty cool ontology. Think of all the buildings and all the animals, and everything that you have to link together, build that ontology. It's pretty fun, actually. So building ontologies, for example, is something that's completely native to information science. Now, we have the possibility to actually not only design these ontologies conceptually, but putting them, bringing them into life in a data catalog, at least if it's a knowledge graph based data catalog.
Juan Sequeda: This is one of the things I am super excited on and why we really clicked on this is because of your background and my background too. We've clicked on the whole issue of semantics, of knowledge, of ontologies and how the ontologies is just a way of being able to go represent knowledge. What we've talked a lot about here, is actually, let me say this again. The word data catalog is something that I think is not enough. It really should be a data and knowledge catalog, because you really want to go catalog what the business represents. You want to be able to catalog represent that knowledge and that knowledge, how is that knowledge represented such that you can catalog it?
Ole Olesen-Bagneux: Yeah.
Juan Sequeda: As an ontology, I think part of that goes into, well, does this automatically exist? Can I go create that? Do I have to go talk to people? This is the social, technical phenomenon that we have to occur, that may exist as a part of processes that you can extract that. But at the end, either if you talk to people, or you're interviewing them or you're able to extract those processes from somewhere, you are building an ontology. You're really representing the way how your domain works. I think that is something that a catalog needs to have, hence why it's more than a data catalog. I don't know. This is my true belief. What are your thoughts here?
Ole Olesen-Bagneux: Yeah. Yeah. I think I need to get a little closer to the mic still. I very much agree on this. I think that catalogs will evolve into machines or repositories, or whatever we want to call them, that represents knowledge to a more intellectually satisfying level than just data itself. So I very much agree. Many of the concepts that come to mind, same to your question and still are questions. Concepts such as, for example, a thesaurus. I'm very accustomed with building a thesaurus, that's a very finely structured vocabulary.
Tim Gasper: A thesaurus, is that what you're saying?
Ole Olesen-Bagneux: Yeah. A thesaurus. Those things I also finally see as something that is possible to build with the data catalog. I think many of the concepts, many of the methodologies that were applied at a conceptual level or are a more raw, technological level in information science, is finally being executed or coming into life by a thesaurus.
Juan Sequeda: I think if you look at catalogs just in general today, having a business glossary is that first step. You're just very basically scratching the surface here. Then you go into that next level, which is having a thesaurus, these different words are related to each other and so forth. Then you start adding more relationships. Oh, this term of an order is placed by a customer, so that relationship's there. And then you can start adding much more detail expressivity of what that knowledge means. I think the way you started out is with a glossary, but little by little, you want to be able to go start cataloging more of that knowledge of an organization.
Ole Olesen-Bagneux: Yeah. Totally, totally.
Juan Sequeda: So one thing we need to go talk about, which is the life cycles of data. All right. I just said life cycle of data, go rant.
Ole Olesen-Bagneux: Yeah. Yeah. One of the things that I discuss in my book and that I really would like to have more focus on, is data life cycles. Also, system life cycles and also the life cycles of assets in catalogs. The reason I'm very occupied with this, is that traditionally in information management, a data management life cycle is a big thing. For how long time should we keep a specific kind of data in our company? For how long time do we keep data about a specific call that we had with, if you're in the life sciences, an HP, healthcare professional? That really depends on whether or not it's subject to GDPR, or if a sample of a specific product that your company is providing was delivered to this person, this HP. Because if it's GDPR, then you have to keep the data for two years. If you delivered a sample of your product, then you have to keep the data about this call for 10 years. Now, this is very, very difficult to manage. It's very difficult to manage. I've tried doing that. I think that data catalogs provide a big potential here, if we can get control of the data life cycle via that data catalog. I hope this resonates a little bit with you, but just to provide a little more context, this is not something that is not taken care of today, but a data life cycle has several phases. The last phases of a data catalog, of a data asset is the disposed phase. It's typically in the disposed phase where you gain control of the retention period of saying," Okay, we will place this data in this storage solution until the end of the retention period." What the data catalog actually offers is to... Yeah. It's very, very difficult to get control of the data life cycle at the latest phase of the life of the data in the disposed phase. Now, the data catalog actually proposes or offers the possibility of gaining control of the life cycle earlier when we store and share the data far earlier in the life of the data. So it's a big potential here for data catalogs. It's not fully developed yet, but I see gaining control of the data life cycle as something that is a potentially big, big win for data catalogs.
Tim Gasper: Right. Yeah. You mentioned a couple of steps here as part of an overall life cycle. And just before I ask this next question, I want to mention that this episode is brought to you by data. world, the data catalog for data mesh, a whole new paradigm for data empowerment. To learn more, go to data. world. Ole, when you mentioned store and share, and you mentioned dispose, these are actually things that come from a framework, right? An information science framework of P- O- S- M- A- D, POSMAD, plan, obtain, store or share, maintain, apply and dispose, which I'll be honest, I was not very familiar with in the past. When you brought it up to Juan and I, I was like," Wow, this makes a lot of sense." It's actually a very simplifying framework for thinking about data life cycle and obviously catalog can play an important part across that entire life cycle. Is POSMAD something that you're thinking a lot of when you think about how a catalog can be effective, and do you see it playing a key role around that?
Ole Olesen-Bagneux: Yeah, totally. The impression I have of data catalog, so far I've worked with a couple of data catalogs in my professional life. Of course, now that I'm writing the book that I'm getting to meet a lot of data catalog vendors. The impression I have of data catalogs is that they simply reflect what is out there, so it's a mirror of the present. I don't know if this sounds too spacey, but it's like if you mirror what's just the data and production, you're not taking into account the data has a life of its own. The fact that data has a life of its own is something that is subject to many very difficult management questions around data. It's very, very difficult to get an overview of data. But once you have that overview of data, you can begin to control the life cycle of data, retention periods of data, which is a big, big thing and will become an even more important thing. The tendency that I've seen in data catalogs is simply to mirror what's out there in production. That's a nice thing. It's totally a nice thing, but the fact is that we have no other tool to control the life cycle of data. In all the source systems, it's just a matter of a lot of service delivery managers trying to provide specifications on how long time the data should be kept in all those systems. But if the data catalog can provide that, it becomes a key player. Not something that limits innovation or puts another layer of governance on top, nothing like that. It just provides smooth management of the data that you have in your organization in a life cycle perspective. That's not something that's present yet in data catalogs.
Tim Gasper: Well, and we have an interesting question here that I think piggybacks off of what you're talking about. On LinkedIn, one of our listeners asks," Any chief data officer or data product owner needs to have a focus on data monetization for their data products to survive. In modern data architecture, most of the cost on the compute side is on the compute side versus the storage side."" So how do we decide which data we want to remove, since data that today has no value can be really valuable in two or three years potentially?" So this is now the data's in the catalog. It's one thing to be a reflection of the present or to be a reflection of production. But then how do you actually leverage the catalog with your own knowledge to make good decisions about data?
Ole Olesen-Bagneux: Yeah. I think there are two answers to that question. The first answer is not an answer that I can give, I'm sorry. I wouldn't be able to provide a relevance, I think. Okay. So to try to provide an answer to both the dimensions in this question, I think it would be up to domain experts to assess the relevance of data and whether or not we should keep it or not. So if you're going with a domain- based approach, then you could rely on domains assessing the relevance of data in a couple of years from now. But there's another dimension to this that is not up for debate, and that is all the legal requirements or regulations that your company is subject to. The bigger your company is, the more regulated it's likely to be. If we take, for example, the petrochemical industries, they are heavily regulated. They need to document a lot of stuff and they need to keep this data for a certain period of time. So it's not up for debate. We need to keep the data. It's just a question of how we keep it and where it is stored, of course. How it's accessible and so on, but we need to keep it. For example, in the pharmaceutical sector where I worked in a substantial part of my work life, you were forced to keep a lot of information, a lot of data for the life of the product plus 35 years. That's the average lifetime of a patient that has been consuming or using your product. So imagine keeping data for that period of time. If you have no overview of that, how do you want to manage it? I have done that and it's totally possible to do with spreadsheets and very, very, very low- tech solutions. But what the data catalog really offers here in this space, is that you crawl the systems that are running and that are supporting the value chain of your company in the present. If you combine that with the retention period, you just get a data governance solution that is something that we have not seen before anywhere in the industry. That's the big potential of data catalogs.
Juan Sequeda: Yeah. The clear takeaway here is that we need to start thinking about what is the life cycle of data?
Ole Olesen-Bagneux: Yeah.
Juan Sequeda: And how that is being managed, tracked, the data catalog needs to be doing that. It's not doing it today, I would argue.
Ole Olesen-Bagneux: No. Yeah. It's not.
Juan Sequeda: That's the potential right now. This is a really, really clear, important takeaway. I think the whole POSMAD, this is a very important thing for everybody to listen. Plan, obtain, store, share, maintain, apply, and dispose. This is a really excellent framework. Another framework that we've talked a lot about, it's something dear to my heart because I always talk about the data first to the knowledge first.
Ole Olesen-Bagneux: Yeah.
Juan Sequeda: Data, information, knowledge, action results. Okay. Let's break this back into practical setting. How should that be related to data catalogs?
Ole Olesen-Bagneux: Yeah. So another thing, I'm very glad you bring this up now, Juan. If not, I would just have kept on ranting. But the DIKAR framework is really not a life cycle. It's more like an interpretation loop. So if we consider data as something that is generated by systems in combination with human activity, then once we look at that data, interpret it, we can understand it, and then the DIKAR frameworks proposes that it becomes information. It's only when we interpret this data, that we have in our source system that we understand it as something particular, and so it becomes information. Now, when we think further about the information, what it is, what it is used for, and in what context it is used, it becomes knowledge to us. We know that this kind of information is used in that context and so on. That's really what this framework proposes, is that we need to look at data and interpret it to be able to understand it as information. That over time, creates knowledge. With this knowledge, we can act and that will create results. So this is not a formula of how knowledge is derived in total, like in human understanding, in human thinking. But thinking specifically about data, this little framework proposes a way to move from data towards knowledge. I have, of course, followed you on LinkedIn. We've been discussing a lot, Juan. I actually think that it's very important that data catalogs move away from data and towards knowledge. I also argue this quite vividly in my book.
Juan Sequeda: Cheers.
Ole Olesen-Bagneux: Yeah.
Juan Sequeda: I want to cheers on this.
Tim Gasper: I'll virtually cheers.
Juan Sequeda: No. Look, the honest, no- BS thing here, this is not just because I work at a company, which is data. world and it's a data catalog, and we're trying to stand out and whatever. No, no. This is I genuinely, I personally believe my heart is in this, that it is not just about the fricking data that's inside the databases. What the fuck does this mean? And understand that context and the people behind that, because otherwise, insanity, keep doing the same thing over and over again, we're not going to understand. So we really need to have this shift. It's just so annoying when people are like," I just want data, I just want data, give more data." It's like," No. Okay, here's the data, so what? What are you going to do with it? Do you understand what this means? Go talk to somebody else about it." I think this is the shift. I am very, very happy that we're on the same page on this stuff. Actually, this is the call to arms to folks. Get out of just your data first world and start talking to other people around you. All right. That was my other rant of the day, Tim, back to you.
Tim Gasper: No, that's perfect. Well, one other comment that I'll have is that people all the time refer to that age- old analogy or progression of like," Well, data to information, information to knowledge, and then knowledge to wisdom." I think that's cute and it's fun, and it's easy to remember, but it's a framework that has a very static connotation. It's a passive connotation, which is we work so hard to build up all this context so that what? So that we can swim in all this context and be like,"Yeah, this is a fun pool to swim in"? Or is it to take action and to achieve results? I think that's cool about this DIKAR framework here. The actions and results, the A and the R, because that's a different way to think about why you're building up this knowledge in a way that's much more active. Honestly, I think has a lot more return on investment oriented around it. Because I think a lot of times people talk about knowledge, they talk about ontologies and things like that. A lot of times they go," How does that impact my business?" I think that you got to change the way you're thinking about it.
Juan Sequeda: To add to this and take it to the next topic before we have to go to our lightning round, because I told you time flies when we're having fun here.
Ole Olesen-Bagneux: Yeah. Yeah.
Juan Sequeda: So on one aspect, the knowledge part, and you brought this up earlier when we're talking about the background of information library sciences, I think this is where we start thinking about ontologies. I think in the past, ontologies has been this really weird word that you want to go say. But at the end of the day, it's really about documenting, cataloging what something means and even a different perspective. I think that data catalogs need to be dealing with ontologies. That needs to be one of the other types of first class citizens that they're cataloging, not just your tables and your columns, generate the lineage of this stuff. It's like you need to say," Hey, can you catalog ontologies too?" Would you agree with that or disagree with that?
Ole Olesen-Bagneux: No, totally. Totally. I would agree with that. Yeah. This is a boring answer, I just agree. I think to provide a little more context, and also going back to the DIKAR model, I don't think we can act probably just based on data. I don't think we can create the results we want just based on data. There's this middle layer where we need to understand what the data means. We can't do that just by looking at it. When we look at data, we understand it as information. We can say," Okay. This is, for example, clinical data." But if we look at clinical data, recognize it as such. Clinical data, because I've worked so much of my life in pharmaceutical industries. Clinical data interprets it as information, mean that humans recognize it as clinical data, but it takes thorough analysis to derive knowledge from that. Looking at certain numbers of figures saying," Okay. This actually looks like this person has cancer." That's just not something you look at something, some numbers, and then you can say," Okay, this is a result of a clinical study. We can see that." But to deduct something from that to provide a diagnosis, it takes further analysis, it takes a long time. That's why you need that knowledge layer before you can actually act.
Juan Sequeda: So one other thing connected to the knowledge in something that we talk a lot about ourselves here and just what we're seeing a lot in the industry. Is this notion of knowledge graphs, especially when it comes to data catalogs and just managing, integrating so many different heterogeneous, diverse sources of data and metadata. I'm in the particular belief, just again, honest, no- BS. Outside of the vendor perspective, a graph is just the ideal structure of how to integrate data, integrate anything because you can represent anything back into a graph. That's why I truly believe that a catalog needs to be represented as a graph and it's the basis of it. What are your perspectives about this? The market's going in so many different places, is this a big thing for you or it's like," Nah, it's just a feature or whatever"?
Ole Olesen-Bagneux: No, it's totally not a feature. A knowledge, graph- based data catalog is something fundamentally different than a data catalog that is not based on a knowledge graph, because it provides you with this flexible meter model, that allows you to precisely map the organization you are in. I think that holds a lot of potential. In my book, I discuss knowledge graphs as something that is key to future data catalogs, because it provides such a more smooth mapping of your organization. But first and foremost, it provides better search experiences.
Juan Sequeda: Okay. So a data catalog powered by a knowledge graph gives you the full flexibility of metadata, to really, precisely map what your organization means. Second, it gives us that whole first topic that we talked about a lot, the entire search expressivity.
Ole Olesen-Bagneux: Yeah, totally.
Juan Sequeda: I think those are two key fundamental aspects of a knowledge graph. Anything else to add around this? This is something that we're talking, people are thinking about is," Do I really need to pay attention to that or not?" Is there something else about that the listeners should consider?
Ole Olesen-Bagneux: Yeah. It's pretty much the same point, but elaborate it a little bit. I think that knowledge graphs really provide the possibility of the combination of simple search and browse features in a way that is very nice. I discuss applied search in data catalogs quite extensively in my book. Of course, different search patterns and especially knowledge graphs really provide this powerful, simple search experience with a browsing experience afterwards that is really nice, because everything of relevance is attached to the hit that you're finding.
Juan Sequeda: Right. Well, this has been a fascinating discussion and I have to say I am extremely, extremely excited. Before coming to this, we were having dinner, and then we'll spend tomorrow talking. We're going to be really just keeping this conversation going. Tim, I think it's time to go to our lightning round. Again, I wish we could continue and we should probably do, I think, future episodes of we'll stop and then we'll have bonus episodes of the episode that we had.
Tim Gasper: Yeah. If we're really on a roll, we should do VIP content afterwards. I don't know, maybe this is a special idea here. We need to have the VIP club and whoever's part of that can hear all the good stuff.
Juan Sequeda: All right. Anyway, let's move to our lightning round, which is again presented by data. world, the data catalog for successful cloud migration. Let me go first. Is reference data, master data?
Ole Olesen-Bagneux: Oh, that question one. That was really mean.
Juan Sequeda: These are lightning round questions, yes or no, and you can give a little bit of context.
Ole Olesen-Bagneux: No. Reference data is not master data.
Juan Sequeda: Give us some context.
Ole Olesen-Bagneux: Reference data can be just as subjective as anything else. Is that context enough?
Juan Sequeda: No, no. To clarify, I think master data we were discussing, the legacy view of this is like," Oh, it's a single version of the truth."
Ole Olesen-Bagneux: Yeah, totally.
Juan Sequeda: That's legacy.
Ole Olesen-Bagneux: Yeah, but it's necessary legacy, I want to add though. It runs the operational backbone of a company to have master data, so it's quite important. But it's not something that is very important in analytical data platform.
Juan Sequeda: All right.
Tim Gasper: That's interesting.
Juan Sequeda: Tim, you go.
Tim Gasper: Yeah. I feel like these things happen in waves. Reference and master data comes back and now people are asking a lot about it again, it's hot again. So next question. You mentioned in our chat today, that data shopping isn't necessarily a core part of the search focus that especially a catalog needs to focus on. But obviously, catalogs do get often pushed and we'll speak as a vendor, get pushed into things like workflow and governance. Do you see that governance workflow and policy is separate from catalog? There are two things that although they get coupled together, they're separate, or are they actually tightly coupled?
Ole Olesen-Bagneux: I think that they are somewhat coupled. I don't think you should try to resolve every data governance issue you have with the data catalog, far from it. But it can provide some basic features or capabilities that will enable you to do data governance. But data governance is something that really has mission creed built into it. So should watch out for that.
Juan Sequeda: All right, third question. Smarter search ontologies, someone has to do this work. We've been advocating for this role, the knowledge scientist or the knowledge engineer. Is this a role that you see that is emerging? Will it emerge?
Ole Olesen-Bagneux: Actually, Scott Hirlman asked me the same question on Data Mesh Radio. I think I answered no. The more I thought about it, I regretted that answer. So I'll try to provide an answer I won't regret in a couple of weeks from now. I totally think that the return of investment in people working with curating data, it's just the potential is just so big. So whatever you want to call it and wherever you want to place those people, I don't really care. It would be such a phenomenon value for companies to have that. Yeah.
Juan Sequeda: That was a safe answer.
Ole Olesen-Bagneux: Yeah. It's also something that I've been working in close to 20 years, not completely, 15 years. But working with data, trying to provide value, fighting my way through people that don't understand information management, data management, don't understand data technologies such as data catalogs. You just want to provide value to the company that you're in. I think that value provided by such people, it's just so much more proportionally to the salary that these people are paid that personally, I can't understand why it hasn't more attention.
Juan Sequeda: All right. Tim, last one.
Tim Gasper: All right, last question. Will the catalog space still exist in five years, or is it turning into something else like knowledge search or some other thing?
Ole Olesen-Bagneux: Yeah. Personally, I think that you have also mentioned this earlier while, so I think it's okay to say that it wasn't a marketing person that invented the term data catalog. It's difficult because people don't know what it is. The core capability, seen from an enterprise architecture perspective, the core capability will remain. It will just evolve, become more powerful. Whatever we call it, may change but the capability itself will remain also in five years.
Tim Gasper: That's a good answer.
Juan Sequeda: More of a naming and marketing thing, but the capabilities are there. I agree with this.
Ole Olesen-Bagneux: We need to have an overview of data, we need to search for data.
Juan Sequeda: Yeah, and knowledge.
Ole Olesen-Bagneux: Yeah, totally. Knowledge in a data world, data is a prerequisite for knowledge.
Tim Gasper: Okay. Well, and sometimes these terms are sticky. How many people are in the BI space and love the term BI?
Ole Olesen-Bagneux: Yeah. Yeah, exactly.
Juan Sequeda: All right. Tim, TTT Tim takes us away with takeaways. You go first, my friend.
Tim Gasper: All right. So great discussion today. Today, we focused on catalogs, which a lot of times we don't. We don't always focus directly on catalog. We talk about how all these other things that are going on with the data landscape that catalog intersects with, so this is awesome. Really love this conversation. Ole, you're obviously a huge expert in this area, so honored to have you here. So you started off with like," Well, what should a catalog do and what makes it unique?" You said that it really has to effectively allow you to search and discover your structured and your unstructured data. You really focused on when Juan asked," What are folks not thinking about enough when it comes to a catalog?" I think the strong focus on a good search experience was a really, really clear theme on a lot of what you were talking about. Really pushing what are folks not thinking enough about? Well, they're not thinking enough about how they can efficiently organize their metadata and their knowledge for good search. When we talked more about search, you went into some of the different use cases around search. Some of them are going to be much more, I wrote down, wide aperture like your sales. You just want to see stuff on sales or something like that. That's going to be a much wider search. Then you're going to refine from there. But sometimes you really want to narrow aperture where you're really trying to do a precise search, you want less hits. That's where maybe you're chaining lots of things together. You're getting very specific about what it is you're looking for. I think this is where knowledge comes in a little bit. Well, who are you, and what's your role? What's the context that can affect how you can get to a more narrow aperture? So search though, search, search, search, and Google as an example of an inspiration around this. We mentioned a little bit about data shopping and how data shopping may or may not really be a core part of this. It's more complimentary to this, but it was interesting to go into that discussion a bit. You talked about a little bit around search in data versus for data, and how there might be different search scenarios depending on what you're doing there. I argued that maybe ideally in the future they're more integrated. Then in general, we talked also about information science and how really information science, library science, these things apply a lot to the world of catalog as well, which I think, Juan, that's a good segue to pass to you for your takeaways.
Juan Sequeda: Yeah. All right, so many here. One, the information science, and I think this is a call to action for folks listening. One, you definitely have to go see Ole's book. I am so lucky that I've been able to go review it and see what's going on. Please go look at information library science, see it from a different perspective. We talked about the information retrieval query language, which is something from your perspective, how you think about it as going back to search where you have the spectrum around search. We really talked about ontologies. How this is just the way to represent knowledge and how the business works, and we need to start cataloging knowledge around this. How to go do this, you start with a business glossary, you go to a thesaurus. Then you can start extending more. Catalogs all today do glossaries, but we need to start pushing for more. So if you have a catalog, you're thinking about knowledge, it's much more than a glossary. It's a starting point, but we need to be able to go represent and catalog more of that knowledge right there. Second, the data life cycle is something that I have to say that I was not thinking about this six months until I started talking to you, is the POSMAD. I'm looking here just on Michael Lee's comment. This conversation was so important. You have given me so much to think about, POSMAD and DIKAR. POSMAD, plan, obtain, store, share, maintain, apply, and dispose. You need to think about the data around this. This life cycle needs to be managed within your data catalog. How do you know if you want to keep the data or not? That depends on the industry requirements and regulations, depends on your particular domain. Data becomes information, which becomes knowledge, actions, and then results. We can't create results just on data. We need that middle layer, which is about what does this data actually mean, so we can actually generate some results? At the end, what we want is to have a knowledge graph powered by a data catalog. I think the two very specific things that you're saying. One, is a data catalog powered by a knowledge graph gives you that flexible metadata model that other non- knowledge graph catalogs can give you. So you can precisely map your organization, actually does what it means. And second, gives you that entire spectrum of search, just from simple keyword search to all that very detailed level. If you have those requirements of having a flexible way of representing your business and having different spectrums of how you want to go search, then that's the requirement. You need to have a data account powered by a knowledge graph. At the end of the day, it's about search, talked about ontologies and knowledge graph, knowledge representing your business, data life cycles and focusing on the results. And to really achieve everything, search on knowledge, data life cycle and results, it's a knowledge graph.
Ole Olesen-Bagneux: Totally.
Juan Sequeda: How did we do? What did we miss on takeaways?
Ole Olesen-Bagneux: I think you make me sound more clever than I am.
Juan Sequeda: We're just summarizing what you just said.
Ole Olesen-Bagneux: Thank you. Thank you. Thank you a lot. Thank you.
Juan Sequeda: All right. We're going to throw this back to you quickly, so three questions. One, what's your advice about data, about life or whatever? Second, who should we invite next? And third, what are the resources that you follow, people, blogs, vlogs, podcasts, whatever?
Ole Olesen-Bagneux: Okay. First, I think some advice. Yeah, I thought a little about this and actually prepared something. This is professional advice and I have always followed a principle very discreetly, but it's not a secret but I've just never spoken about it. I have always surrounded myself with people that are more clever than myself. I have never, ever insisted in being right when I discuss with these people, but I have always insisted on what I know. That is something that has given me a very, very nice work life, because it gives you the possibility to grow without discussion but you need to find people. Don't follow famous people. Follow people at work that you think are more clever than yourself. Ask them anything you want to know. Insist on what you know and learn from that. I think that is true for where I work currently, actually. We have a lot of issues where I work technically, but I am very fond to be part of the very small enterprise architecture team and very fond of my CIO.
Juan Sequeda: All right. Well, that's a very beautiful piece of advice, especially that last part. What you said is always insist on what you know.
Ole Olesen-Bagneux: Yeah, totally. Never insist on being right, but always insist on what you know.
Juan Sequeda: Okay. Who should be right next?
Ole Olesen-Bagneux: I think you should invite the Swedish professor that is called Jutta Haider. She has written a book, co- authored a book that is called Invisible Search and Online Search Engines. It's second chapter is, I think, the most comprehensive overview of the study of search in the 20th century, actually.
Juan Sequeda: Oh, wow.
Ole Olesen-Bagneux: Yeah.
Juan Sequeda: All right.
Ole Olesen-Bagneux: Fantastic book.
Juan Sequeda: All right. Finally, what resources would you like to share with our audience?
Ole Olesen-Bagneux: Yeah. That one I didn't prepare. I don't really know. I follow so many data podcasts. I follow your podcast quite intensively. Monday Morning Data Chat, Data Mesh Radio, Data Engineering Podcast. I follow, let me see. I follow the Data Strategy Show, the Data Skeptic, Data Framed, Meet DMA Norway, that's Data Management Norway, the Data Chief, Experiencing Data, Soda Podcast, Data Lab Dialogues, Data Creators by Mehdio. He also has a Data Creators Club website, Agile Data, the Data Download. I could go on and on.
Juan Sequeda: Wow. Thank you for shouting out for everybody, because I think all those podcasts are fantastic. It's almost full- time to go follow everybody there, but Ole, this was fantastic. I am so excited. Thank you so much for meeting us. And just a quick reminder, this was episode 99. We've been doing this for 99 weeks. We've had bonus episodes or done more than that, but Tim, we've been doing this for over two and a half years. I can't believe this.
Tim Gasper: I know, crazy. Next week then is going to be 100.
Juan Sequeda: 100. This is crazy, 100th episode and it's going to be actually with the VP of product of Fivetran with Fraser Harris. Next week, it's week 100. We are going to be in London. Tim and I are both going to be in London. We're going to do the episode. Fraser unfortunately couldn't make it to London, but we'll be there. We're going to do a special episode on Wednesday and on Thursday right after our summit. If you find us at Big Data London, come reach out to us. We have T- shirts for every person who comes to us and tells us who is their favorite guest and why. We're so much looking forward to meeting a lot of our audience at Big Data London. With that, Ole, thank you so much.
Ole Olesen-Bagneux: Thank you, Juan. Thank you, Tim.
Tim Gasper: Cheers, Ole.
Juan Sequeda: Cheers, everybody.
Speaker 1: This is Catalog& Cocktails. A special thanks to data.world for supporting the show. Karli Burghoff for producing, John Williams and Brian Jacob for the show music. Thank you to the entire Catalog& Cocktails fan base. Don't forget to subscribe, rate and review wherever you listen to your podcasts.
DESCRIPTION
Data Catalogs are at the center of every enterprise’s data strategy. It’s important to explore the current state and how data catalogs are evolving. Who better to talk about Data Catalog than the author of the upcoming O’Reilly book “The Enterprise Data Catalog”, Ole Olesen-Bagneux from GN.
Join Tim, Juan, Ole to discuss the future of data catalogs and why knowledge is at the center.
Today's Host
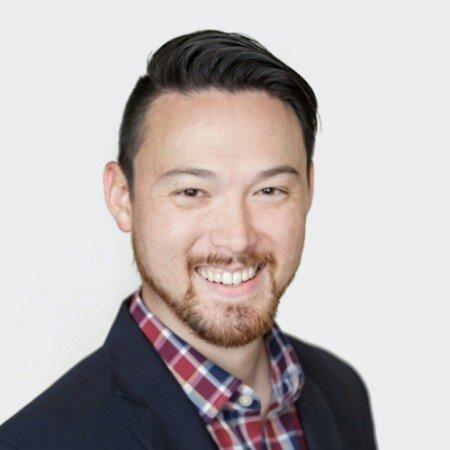
Tim Gasper
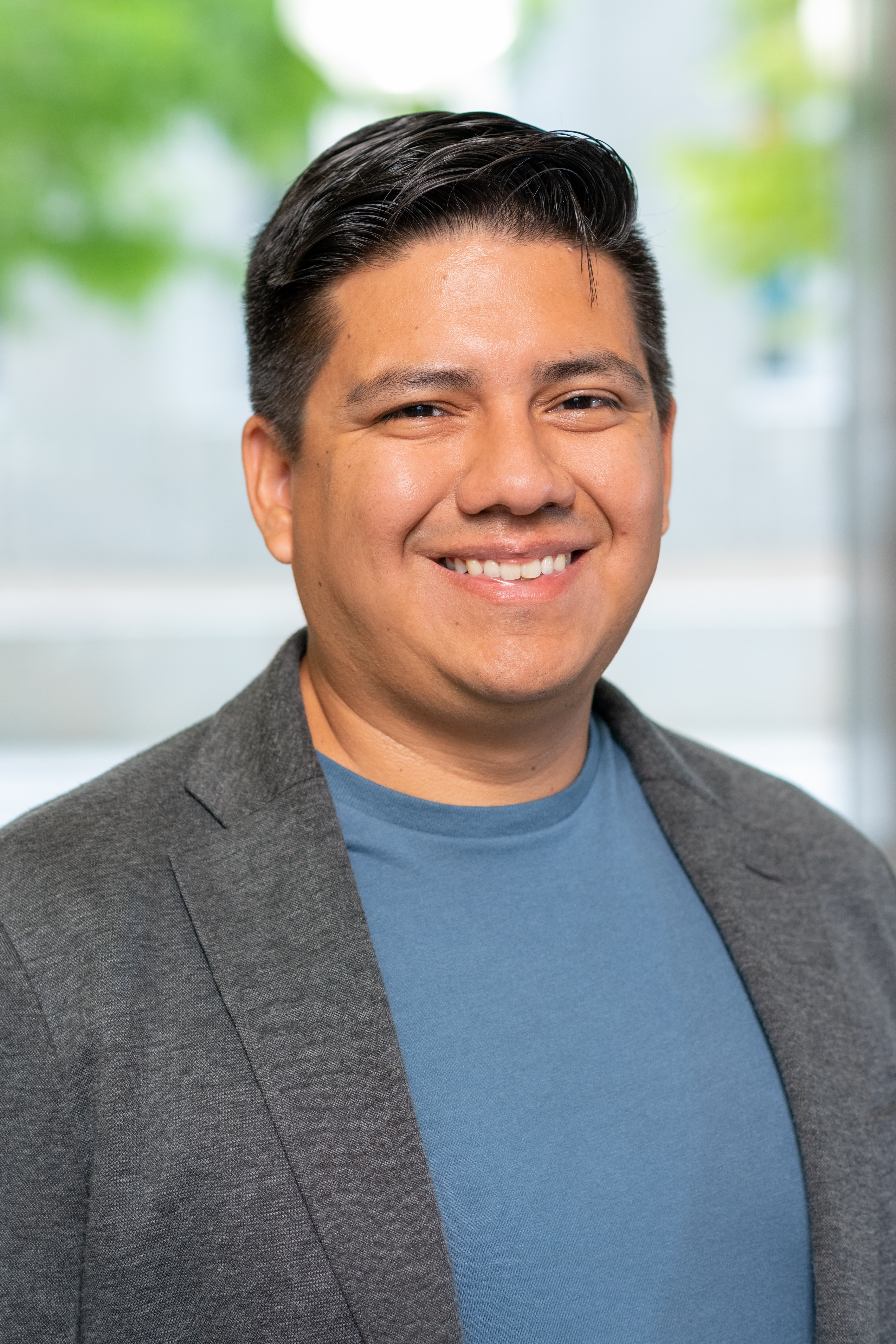
Juan Sequeda
Today's Guests
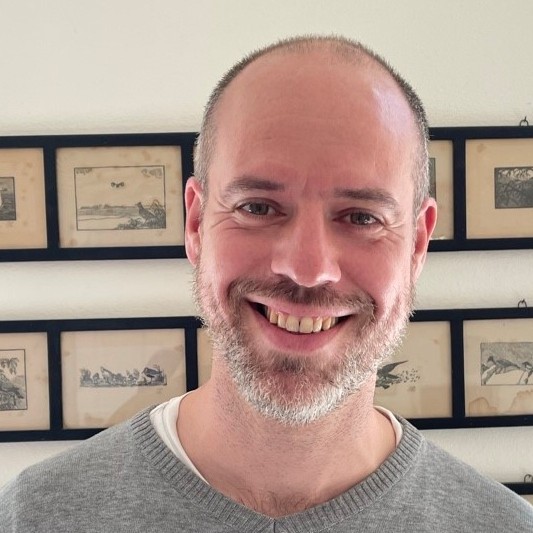